Estimating personalized diagnostic rules depending on individualized characteristics.
STATISTICS IN MEDICINE(2017)
Abstract
There is an increasing demand for personalization of disease screening based on assessment of patient risk and other characteristics. For example, in breast cancer screening, advanced imaging technologies have made it possible to move away from one-size-fits-all' screening guidelines to targeted risk-based screening for those who are in need. Because diagnostic performance of various imaging modalities may vary across subjects, applying the most accurate modality to the patients who would benefit the most requires personalized strategy. To address these needs, we propose novel machine learning methods to estimate personalized diagnostic rules for medical screening or diagnosis by maximizing a weighted combination of sensitivity and specificity across subgroups of subjects. We first develop methods that can be applied when competing modalities or screening strategies that are observed on the same subject (paired design). Next, we present methods for studies where not all subjects receive both modalities (unpaired design). We study theoretical properties including consistency and risk bound of the personalized diagnostic rules and conduct simulation studies to examine performance of the proposed methods. Lastly, we analyze data collected from a brain imaging study of Parkinson's disease using positron emission tomography and diffusion tensor imaging with paired and unpaired designs. Our results show that in some cases, a personalized modality assignment is estimated to improve empirical area under the receiver operating curve compared with a one-size-fits-all' assignment strategy. Copyright (c) 2016 John Wiley & Sons, Ltd.
MoreTranslated text
Key words
weighted support vector machine,personalized screening,Parkinson's disease
AI Read Science
Must-Reading Tree
Example
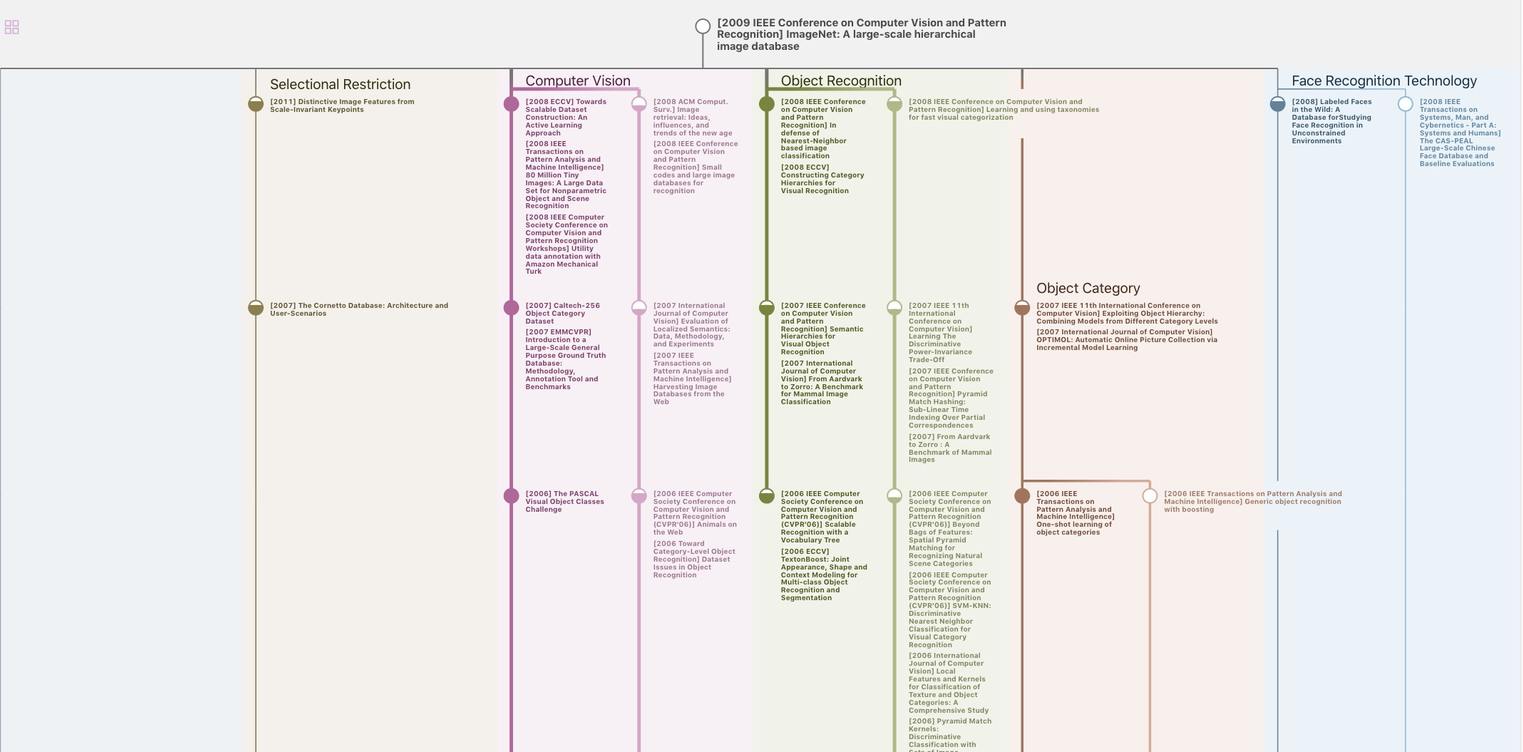
Generate MRT to find the research sequence of this paper
Chat Paper
Summary is being generated by the instructions you defined