Identifying and Investigating Unexpected Response to Treatment: A Diabetes Case Study.
BIG DATA(2016)
摘要
The availability of electronic health records creates fertile ground for developing computational models of various medical conditions. We present a new approach for detecting and analyzing patients with unexpected responses to treatment, building on machine learning and statistical methodology. Given a specific patient, we compute a statistical score for the deviation of the patient's response from responses observed in other patients having similar characteristics and medication regimens. These scores are used to define cohorts of patients showing deviant responses. Statistical tests are then applied to identify clinical features that correlate with these cohorts. We implement this methodology in a tool that is designed to assist researchers in the pharmaceutical field to uncover new features associated with reduced response to a treatment. It can also aid physicians by flagging patients who are not responding to treatment as expected and hence deserve more attention. The tool provides comprehensive visualizations of the analysis results and the supporting data, both at the cohort level and at the level of individual patients. We demonstrate the utility of our methodology and tool in a population of type II diabetic patients, treated with antidiabetic drugs, and monitored by the HbA(1C) test.
更多查看译文
关键词
association tests,contextual anomaly detection,electronic medical records,machine learning application,metric learning,patient similarity
AI 理解论文
溯源树
样例
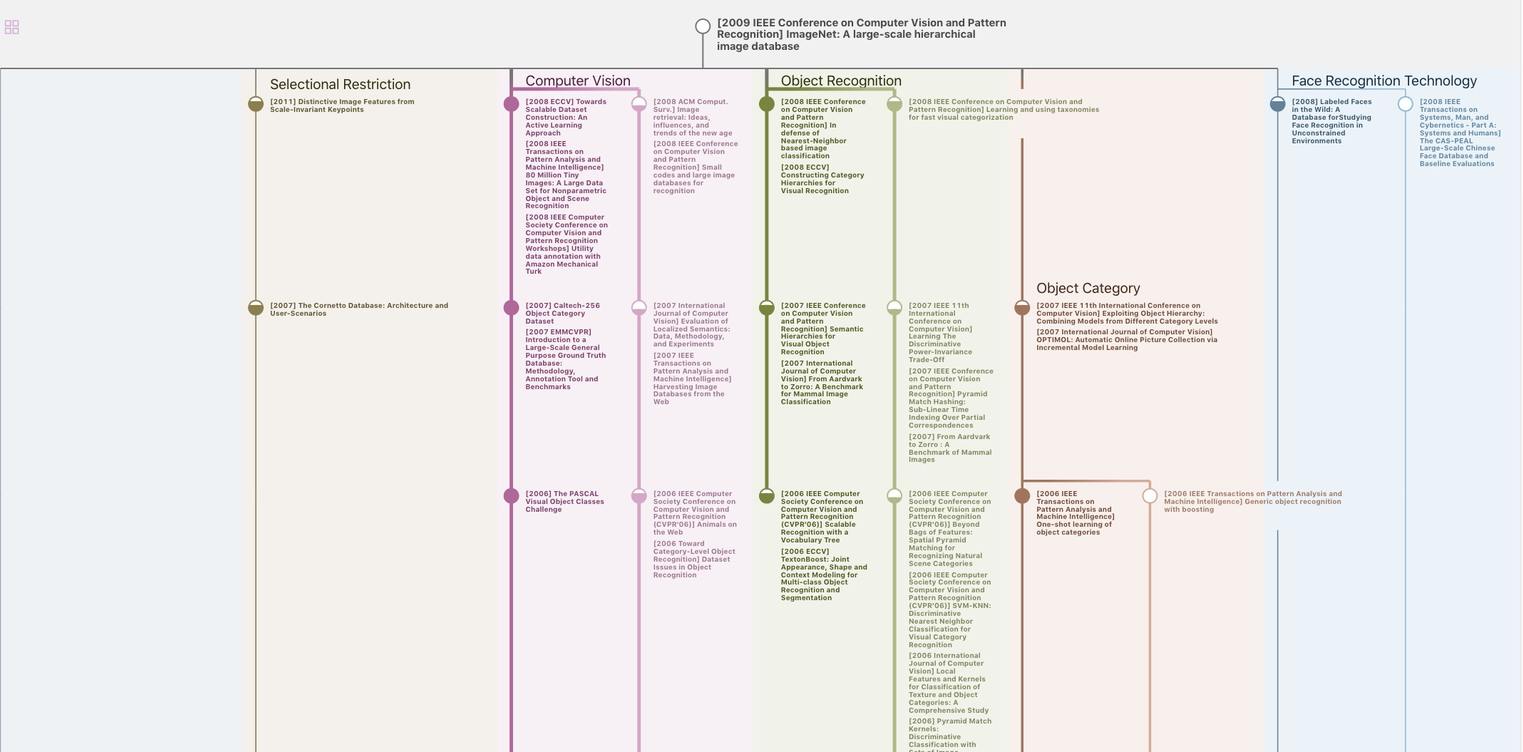
生成溯源树,研究论文发展脉络
Chat Paper
正在生成论文摘要