Inclusion of edaphic predictors for enhancement of models to determine distribution of soil-transmitted helminths: the case of Zimbabwe
Parasites & vectors(2018)
摘要
Background Reliable mapping of soil-transmitted helminth (STH) parasites requires rigorous statistical and machine learning algorithms capable of integrating the combined influence of several determinants to predict distributions. This study tested whether combining edaphic predictors with relevant environmental predictors improves model performance when predicting the distribution of STH, Ascaris lumbricoides and hookworms at a national scale in Zimbabwe. Methods Geo-referenced parasitological data obtained from a 2010/2011 national survey indicating a confirmed presence or absence of STH among school children aged 10–15 years was used to calibrate ten species distribution models (SDMs). The performance of SDMs calibrated with a set of environmental and edaphic variables was compared to that of SDMs calibrated with environmental variables only. Model performance was evaluated using the true skill statistic and receiver operating characteristic curve. Results Results show a significant improvement in model performance for both A. lumbricoides and hookworms for all ten SDMs after edaphic variables were combined with environmental variables in the modelling of the geographical distribution of the two STHs at national scale. Using the top three performing models, a consensus prediction was developed to generate the first continuous maps of the potential distribution of the two STHs in Zimbabwe. Conclusions The findings from this study demonstrate significant model improvement if relevant edaphic variables are included in model calibration resulting in more accurate mapping of STH. The results also provide spatially-explicit information to aid targeted control of STHs in Zimbabwe and other countries with STH burden.
更多查看译文
关键词
Ascaris lumbricoides,Hookworms,Gradient boosted model,Maxent,Species distribution,Soil-transmitted helminths
AI 理解论文
溯源树
样例
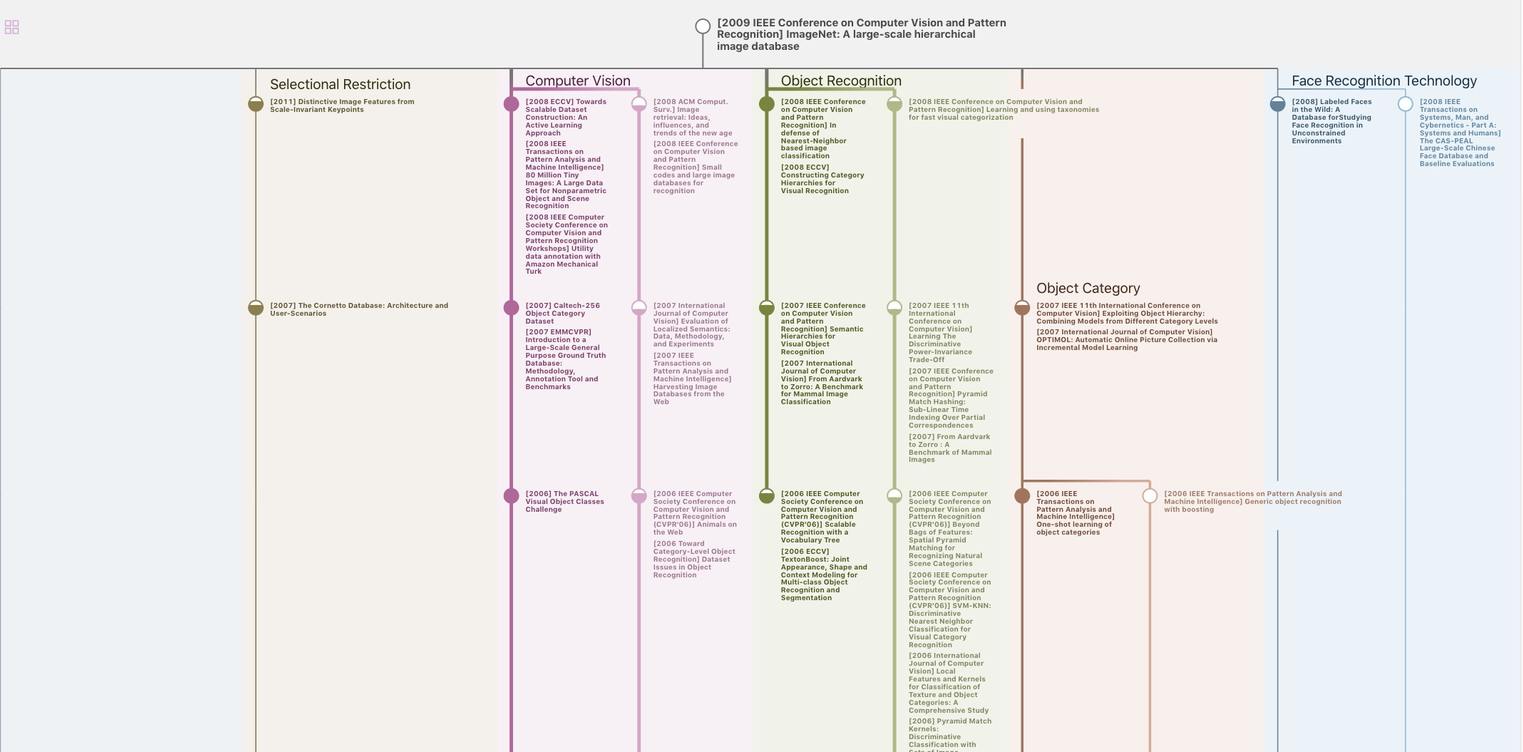
生成溯源树,研究论文发展脉络
Chat Paper
正在生成论文摘要