The impact on high-grade serous ovarian cancer of obesity and lipid metabolism-related gene expression patterns: the underestimated driving force affecting prognosis.
JOURNAL OF CELLULAR AND MOLECULAR MEDICINE(2018)
Abstract
To investigate whether specific obesity/metabolism-related gene expression patterns affect the survival of patients with ovarian cancer. Clinical and genomic data of 590 samples from the high-grade ovarian serous carcinoma (HGOSC) study of The Cancer Genome Atlas (TCGA) and 91 samples from the Australian Ovarian Cancer Study were downloaded from the International Cancer Genome Consortium (ICGC) portal. Clustering of mRNA microarray and reverse-phase protein array (RPPA) data was performed with 83 consensus driver genes and 144 obesity and lipid metabolism-related genes. Association between different clusters and survival was analyzed with the Kaplan-Meier method and a Cox regression. Mutually exclusive, co-occurrence and network analyses were also carried out. Using RNA and RPPA data, it was possible to identify two subsets of HGOSCs with similar clinical characteristics and cancer driver mutation profiles (e.g. TP53), but with different outcome. These differences depend more on up-regulation of specific obesity and lipid metabolism-related genes than on the number of gene mutations or copy number alterations. It was also found that CD36 and TGF-beta are highly up-regulated at the protein levels in the cluster with the poorer outcome. In contrast, BSCL2 is highly up-regulated in the cluster with better progression-free and overall survival. Different obesity/metabolism-related gene expression patterns constitute a risk factor for prognosis independent of the therapy results in the Cox regression. Prognoses were conditioned by the differential expression of obesity and lipid metabolism-related genes in HGOSCs with similar cancer driver mutation profiles, independent of the initial therapeutic response.
MoreTranslated text
Key words
ovarian cancer,lipid metabolism,survival statistics,obesity,clusters,microarray,bioinformatics
AI Read Science
Must-Reading Tree
Example
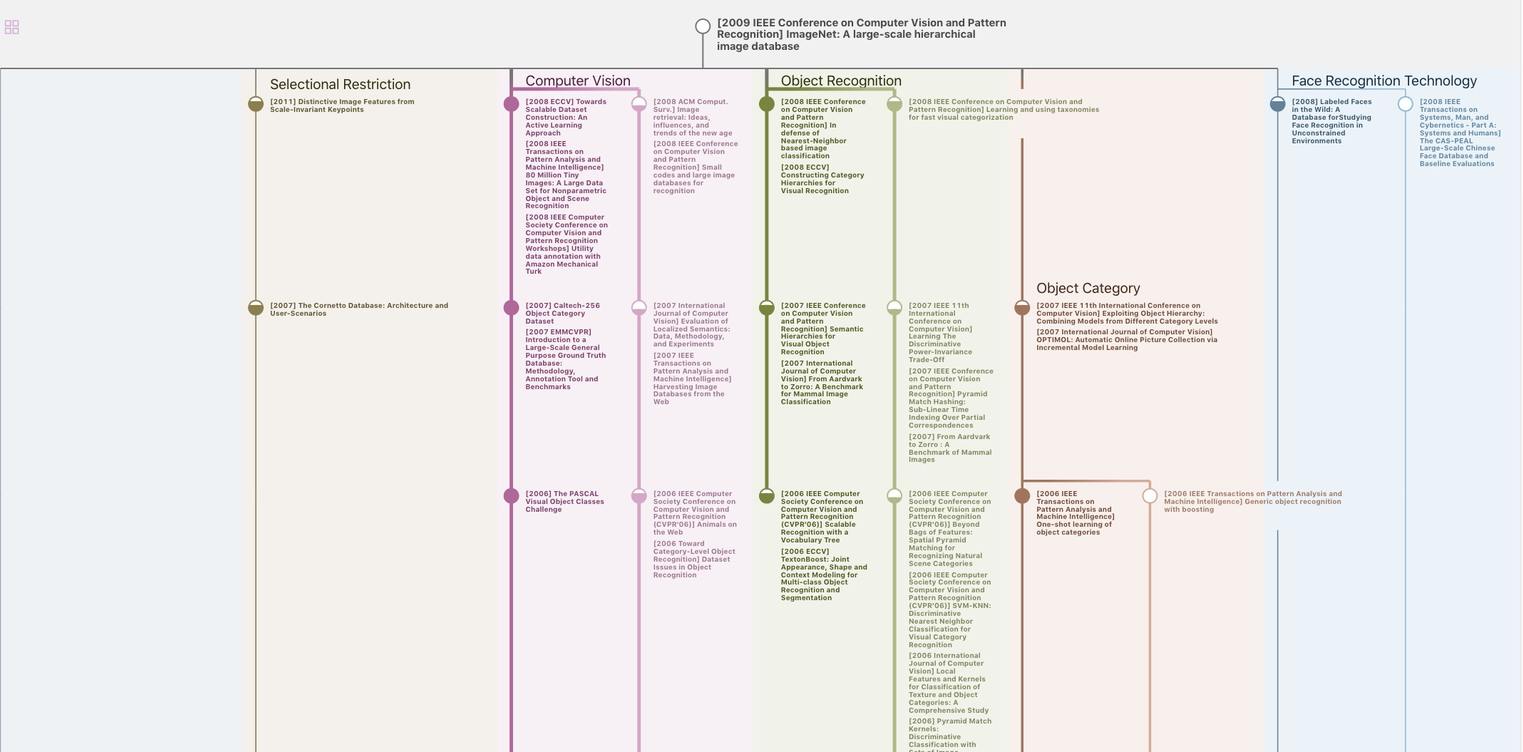
Generate MRT to find the research sequence of this paper
Chat Paper
Summary is being generated by the instructions you defined