Fast block-wise partitioning for extreme multi-label classification
arXiv: Learning(2023)
摘要
Extreme multi-label classification aims to learn a classifier that annotates an instance with a relevant subset of labels from an extremely large label set. Many existing solutions embed the label matrix to a low-dimensional linear subspace, or examine the relevance of a test instance to every label via a linear scan. In practice, however, those approaches can be computationally exorbitant. To alleviate this drawback, we propose a Block-wise Partitioning (BP) pretreatment that divides all instances into disjoint clusters, to each of which the most frequently tagged label subset is attached. One multi-label classifier is trained on one pair of instance and label clusters, and the label set of a test instance is predicted by first delivering it to the most appropriate instance cluster. Experiments on benchmark multi-label data sets reveal that BP pretreatment significantly reduces prediction time, and retains almost the same level of prediction accuracy.
更多查看译文
关键词
Extreme multi-label problems,Non-convex optimization,Scalable classification,Supervised learning
AI 理解论文
溯源树
样例
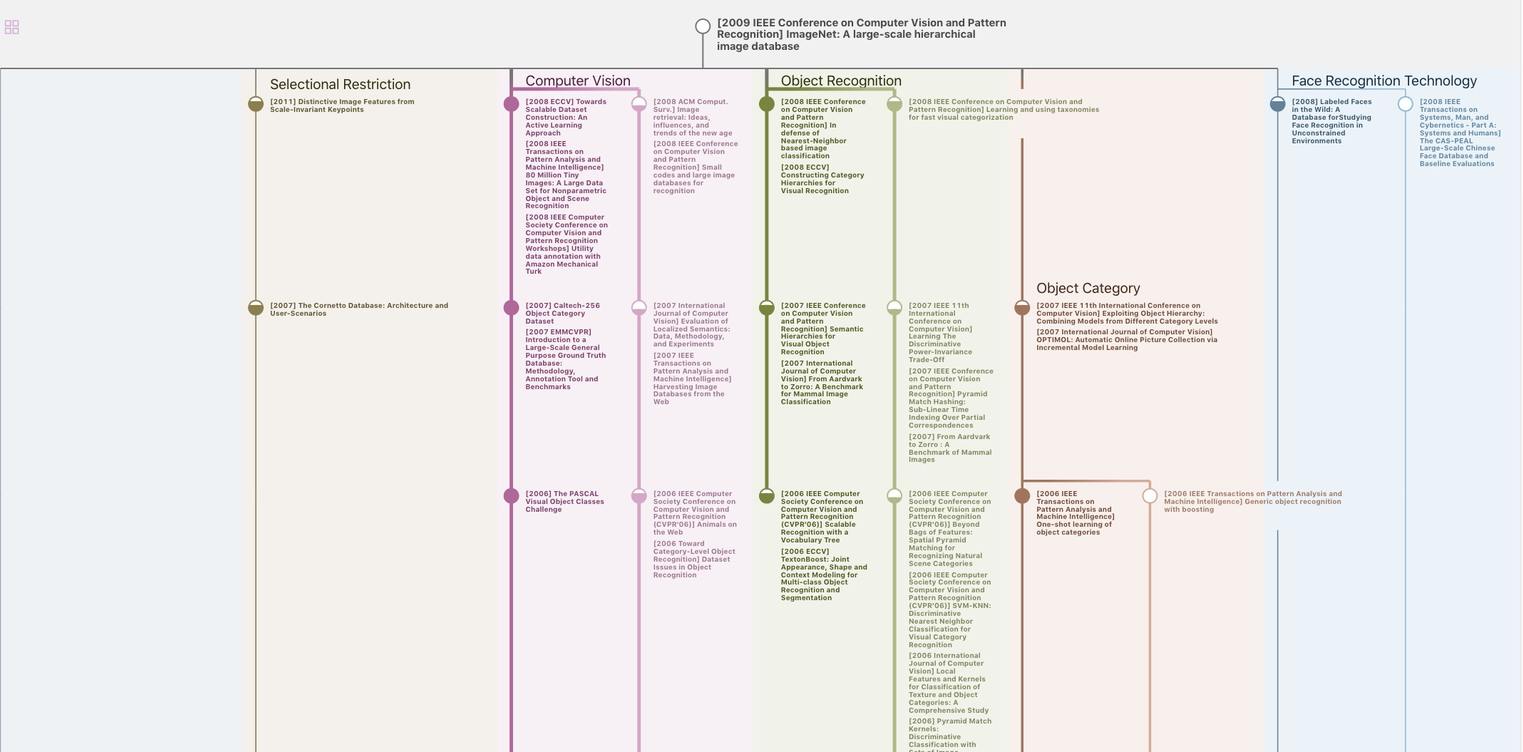
生成溯源树,研究论文发展脉络
Chat Paper
正在生成论文摘要