Learning to Defense by Learning to Attack.
arXiv: Learning(2018)
摘要
Adversarial training provides a principled approach for training robust neural networks. From an optimization perspective, the adversarial training is essentially solving a minmax robust optimization problem. The outer minimization is trying to learn a robust classifier, while the inner maximization is trying to generate adversarial samples. Unfortunately, such a minmax problem is very difficult to solve due to the lack of convex-concave structure. This work proposes a new adversarial training method based on a general learning-to-learn framework. Specifically, instead of applying the existing hand-design algorithms for the inner problem, we learn an optimizer, which is parametrized as a convolutional neural network. At the same time, a robust classifier is learned to defense the adversarial attack generated by the learned optimizer. Our experiments demonstrate that our proposed method significantly outperforms existing adversarial training methods on CIFAR-10 and CIFAR-100 datasets.
更多查看译文
关键词
learning,defense,attack
AI 理解论文
溯源树
样例
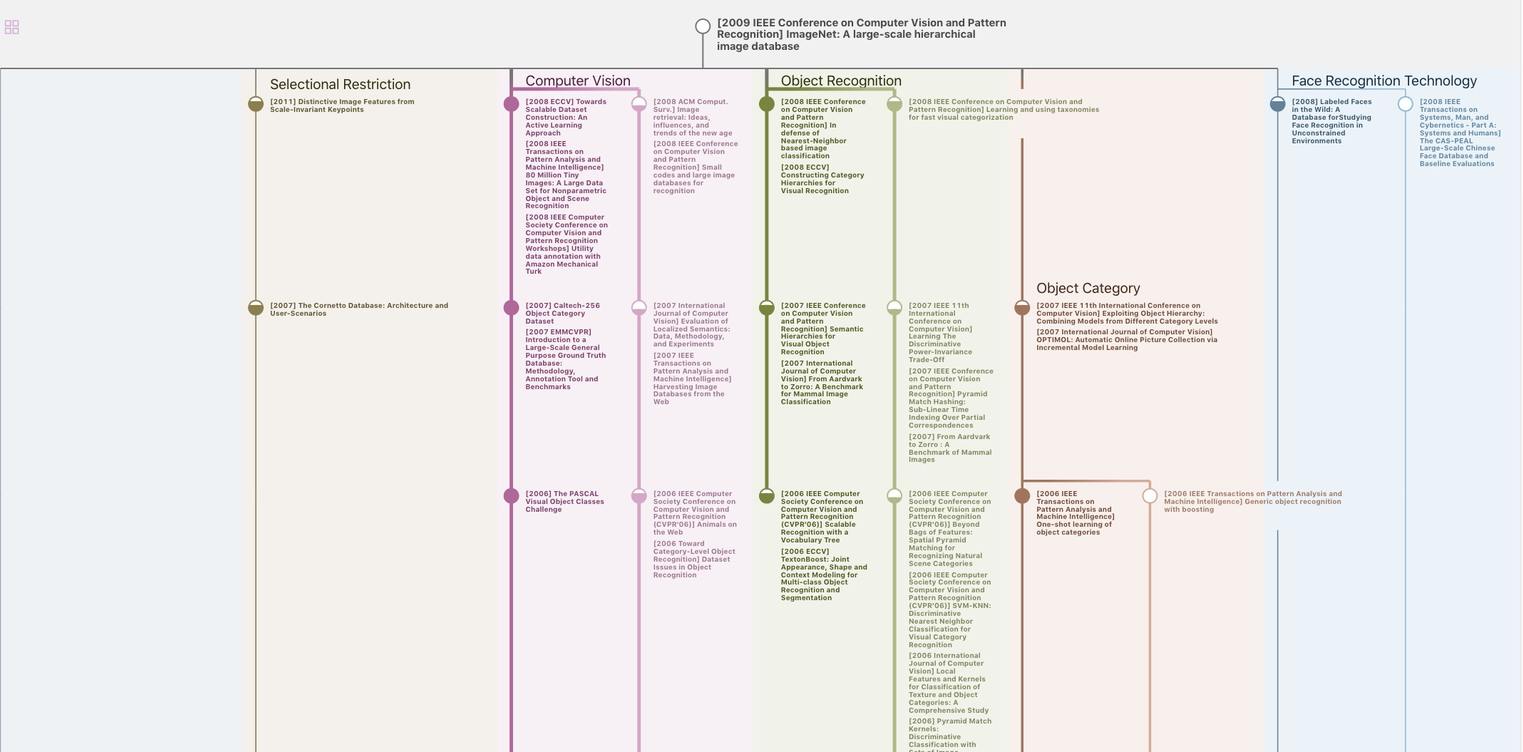
生成溯源树,研究论文发展脉络
Chat Paper
正在生成论文摘要