Improving Span-based Question Answering Systems with Coarsely Labeled Data.
arXiv: Computation and Language(2018)
摘要
We study approaches to improve fine-grained short answer Question Answering models by integrating coarse-grained data annotated for paragraph-level relevance and show that coarsely annotated data can bring significant performance gains. Experiments demonstrate that the standard multi-task learning approach of sharing representations is not the most effective way to leverage coarse-grained annotations. Instead, we can explicitly model the latent fine-grained short answer variables and optimize the marginal log-likelihood directly or use a newly proposed emph{posterior distillation} learning objective. Since these latent-variable methods have explicit access to the relationship between the fine and coarse tasks, they result in significantly larger improvements from coarse supervision.
更多查看译文
AI 理解论文
溯源树
样例
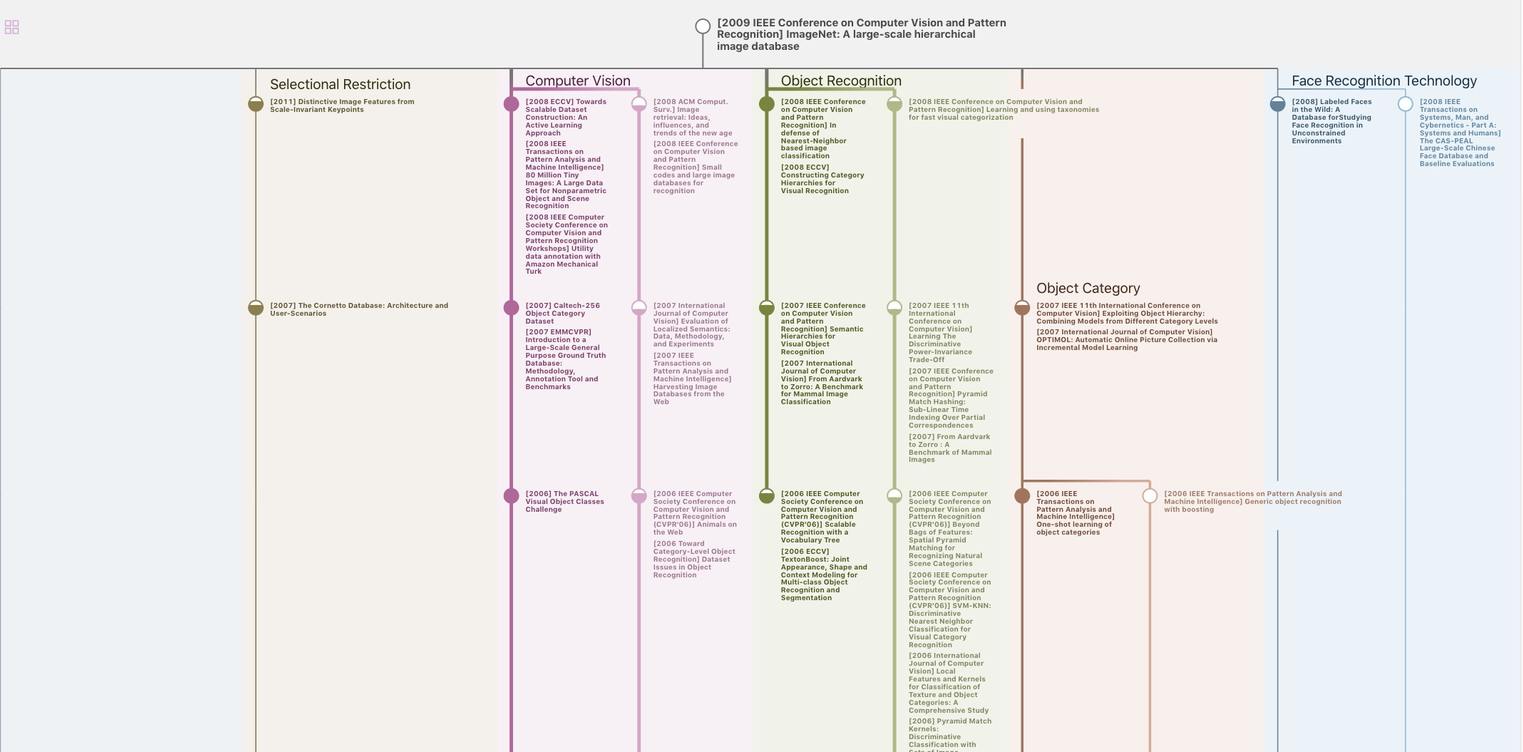
生成溯源树,研究论文发展脉络
Chat Paper
正在生成论文摘要