Improving Hospital Mortality Prediction with Medical Named Entities and Multimodal Learning.
arXiv: Computation and Language(2018)
摘要
Clinical text provides essential information to estimate the acuity of a patient during hospital stays in addition to structured clinical data. In this study, we explore how clinical text can complement a clinical predictive learning task. We leverage an internal medical natural language processing service to perform named entity extraction and negation detection on clinical notes and compose selected entities into a new text corpus to train document representations. We then propose a multimodal neural network to jointly train time series signals and unstructured clinical text representations to predict the in-hospital mortality risk for ICU patients. Our model outperforms the benchmark by 2% AUC.
更多查看译文
AI 理解论文
溯源树
样例
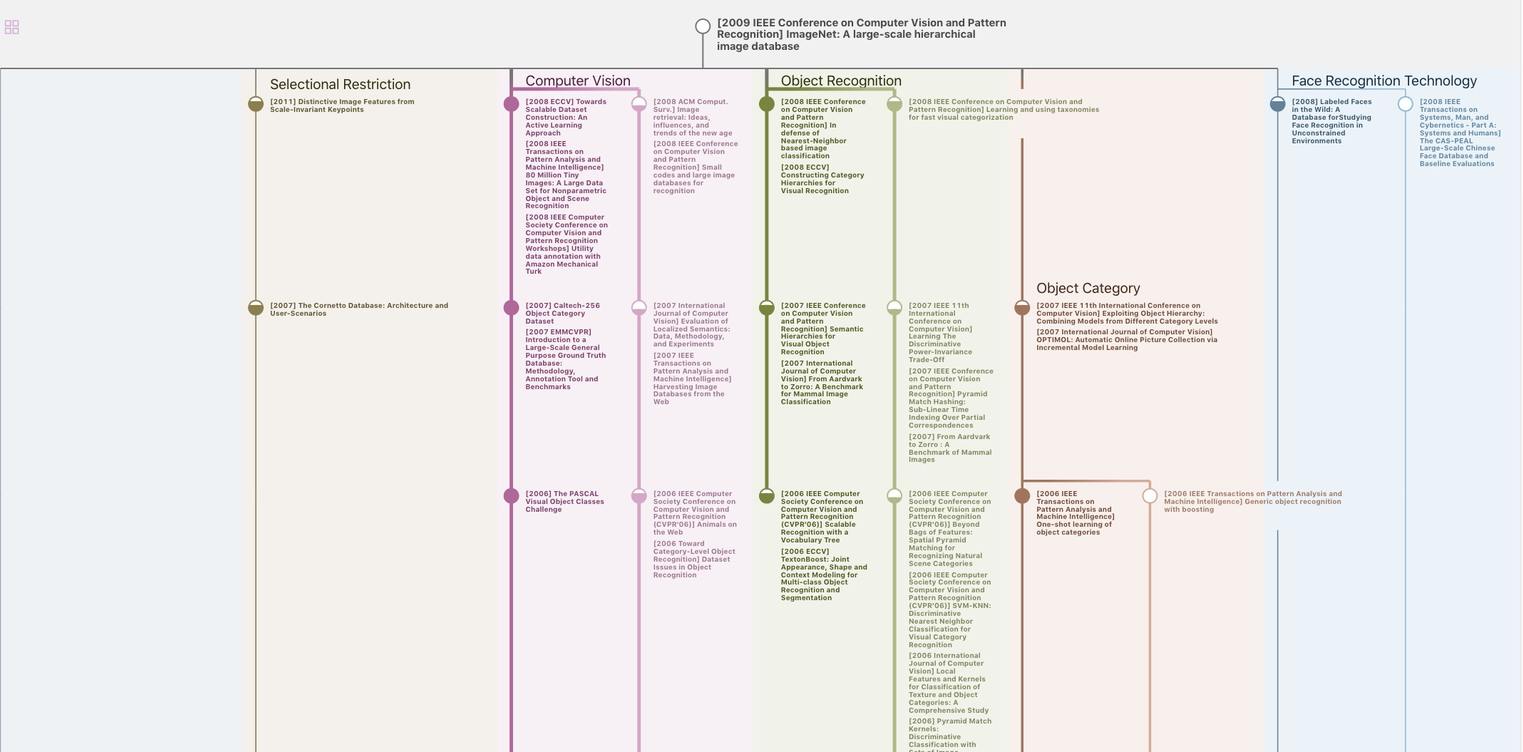
生成溯源树,研究论文发展脉络
Chat Paper
正在生成论文摘要