An Active Learning Method Using Clustering And Committee-Based Sample Selection For Sound Event Classification
2018 16TH INTERNATIONAL WORKSHOP ON ACOUSTIC SIGNAL ENHANCEMENT (IWAENC)(2018)
Abstract
This paper proposes an active learning method to control a labeling process for efficient annotation of acoustic training material, which is used for training sound event classifiers. The proposed method performs K-medoids clustering over an initially unlabeled dataset, and medoids as local representatives, are presented to an annotator for manual annotation. The annotated label on a medoid propagates to other samples in its cluster for label prediction. After annotating the medoids, the annotation continues to the unexamined sounds with mismatched prediction results from two classifiers, a nearest-neighbor classifier and a model-based classifier, both trained with annotated data. The annotation on the segments with mismatched predictions are ordered by the distance to the nearest annotated sample, farthest first. The evaluation is made on a public environmental sound dataset. The labels obtained through a labeling process controlled by the proposed method are used to train a classifier, using supervised learning. Only 20% of the data needs to be manually annotated with the proposed method, to achieve the accuracy with all the data annotated. In addition, the proposed method clearly outperforms other active learning algorithms proposed for sound event classification through all the experiments, simulating varying fraction of data that is manually labeled.
MoreTranslated text
Key words
active learning, K-medoids clustering, committee-based sample selection, sound event classification
AI Read Science
Must-Reading Tree
Example
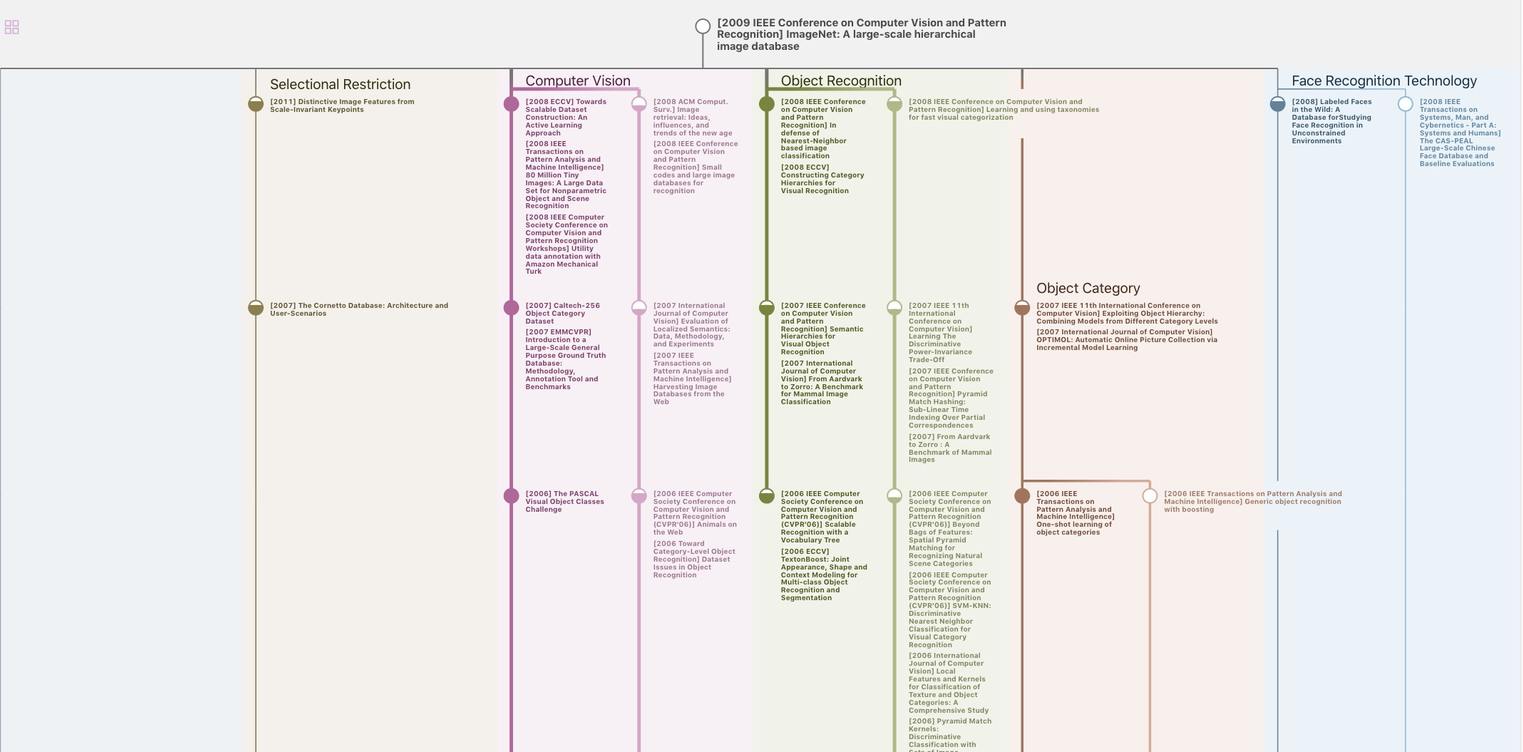
Generate MRT to find the research sequence of this paper
Chat Paper
Summary is being generated by the instructions you defined