Improving Statistical Model-Based Speech Enhancement With Deep Neural Networks
2018 16TH INTERNATIONAL WORKSHOP ON ACOUSTIC SIGNAL ENHANCEMENT (IWAENC)(2018)
摘要
This paper presents a framework for improving the performance of statistical model-based single-channel speech enhancement systems by using a deep neural network (DNN). A DNN is trained to predict speech presence in the input signal, and this information is lever-aged to design novel methods for noise tracking and a priori signal-to-noise ratio (SNR) estimation, which remain the most challenging tasks in conventional systems. The proposed framework provides increased flexibility for various aspects of system design such as gain estimation, relative to end-to-end DNN-based systems. Additionally, the DNN can be trained to detect speech in the presence of both noise and reverberation, leading to joint suppression of additive noise and reverberation. The proposed framework provides significant improvements in objective speech quality metrics relative to baseline systems, and the proposed system was heavily favored in a subjective preference test.
更多查看译文
关键词
Single-channel speech enhancement, reverberation suppression, deep neural network, noise estimation
AI 理解论文
溯源树
样例
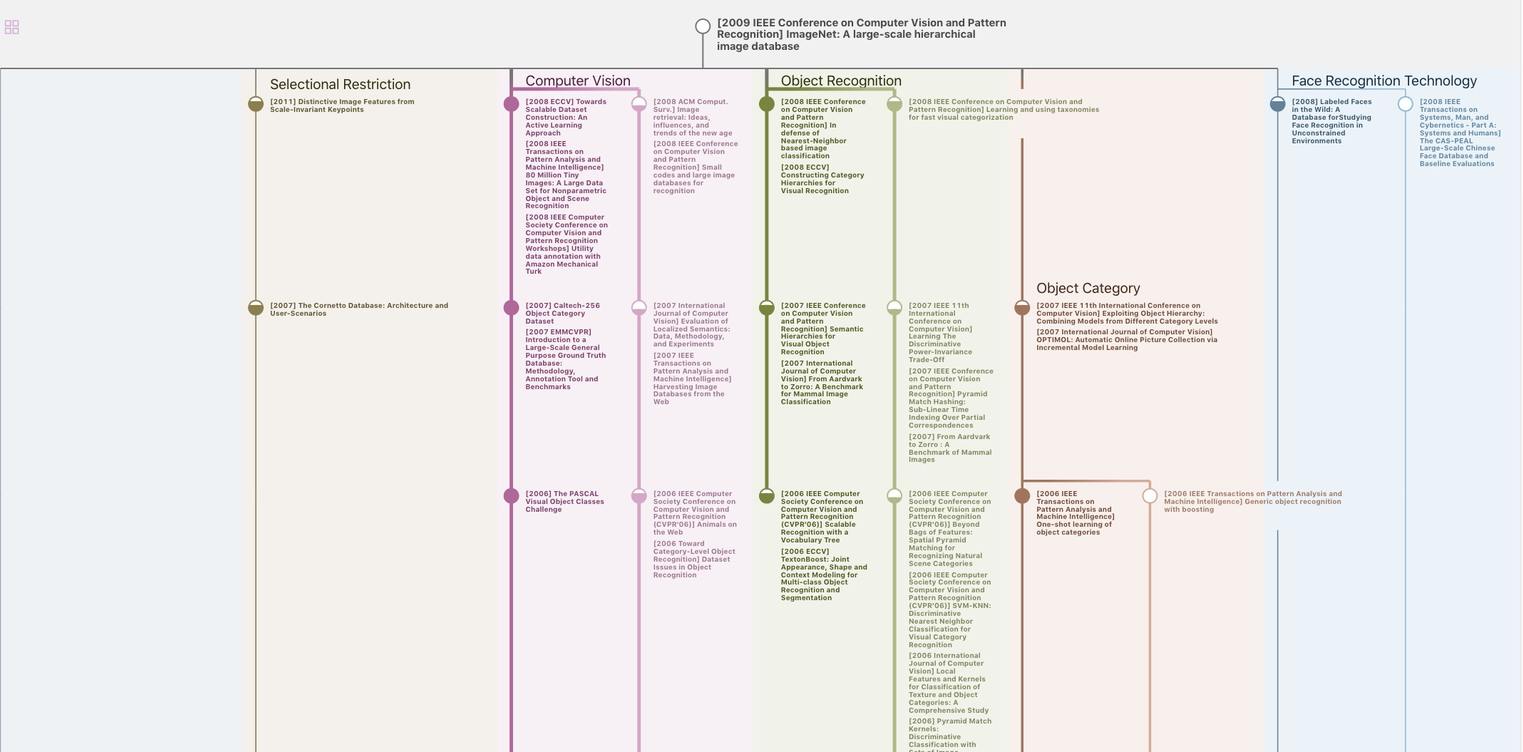
生成溯源树,研究论文发展脉络
Chat Paper
正在生成论文摘要