Instance and feature weighted k-nearest-neighbors algorithm.
ESANN(2016)
摘要
We present a novel method that aims at providing a more stable selection of feature subsets when variations in the training process occur. This is accomplished by using an instance-weighting process -assigning different importances to instances as a preprocessing step to a feature weighting method that is independent of the learner, and then making good use of both sets of computed weigths in a standard Nearest-Neighbours classifier.We report extensive experimentation in well-known benchmarking datasets as well as some challenging microarraygene expression problems. Our results show increases in stability for most subset sizes and most problems, withoutcompromising prediction accuracy.
更多查看译文
AI 理解论文
溯源树
样例
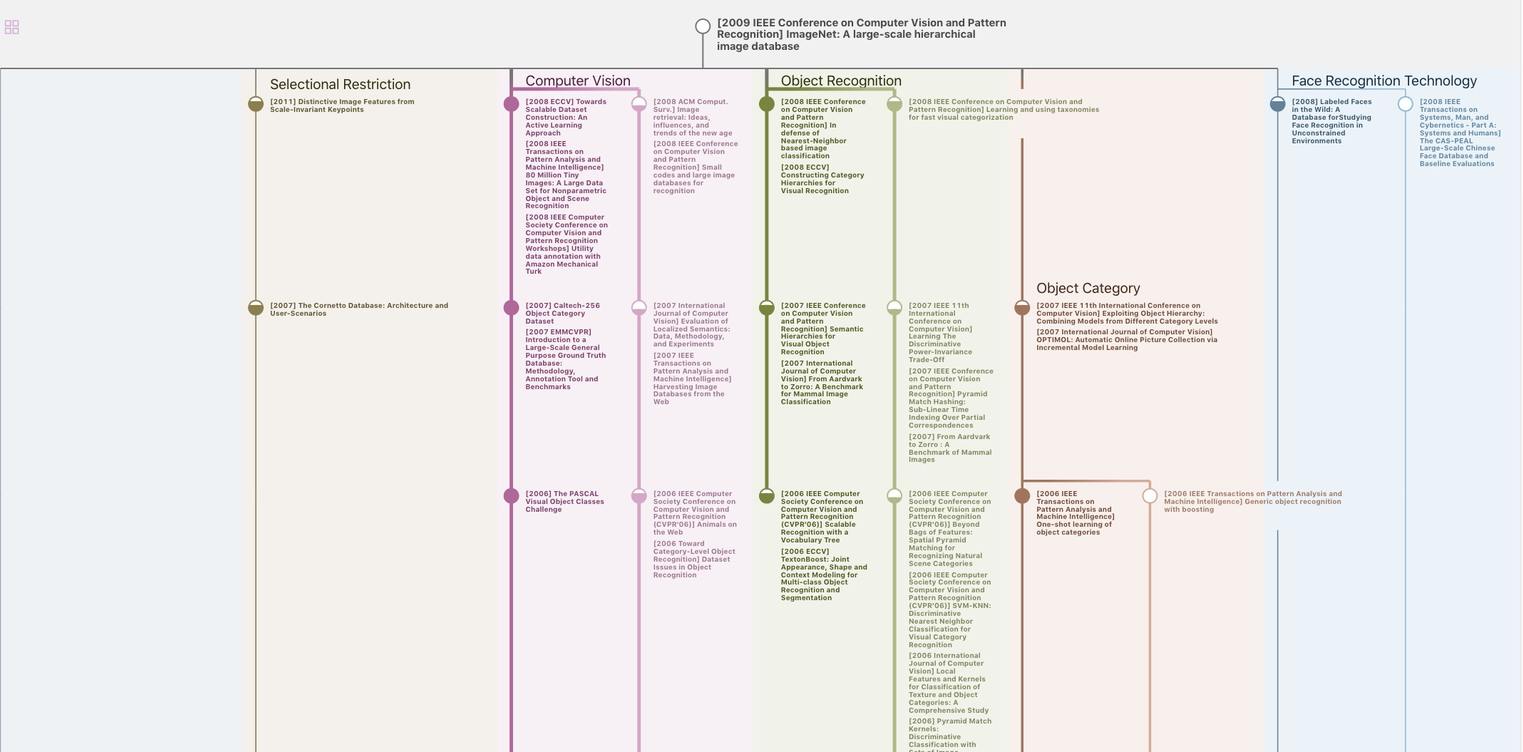
生成溯源树,研究论文发展脉络
Chat Paper
正在生成论文摘要