A Hierarchical Conditional Attention-Based Neural Networks for Paraphrase Generation.
MIWAI(2018)
摘要
Sequence-to-Sequence (Seq2Seq) learning has immense interest in recent years. The prosperous approach of end-to-end training fashion using encoder-decoder neural networks like machine translation has sprouted active research in transduction tasks such as abstractive summarization or especially Paraphrase Generation (PG). Dealing with paraphrase generation problem, one of the most intrinsic obstruction of existing solutions do not pay enough attention to the fact that words and sentences in particular context own differential importance. Consequently, the loss of crucial information probably occurs and irrelevant paraphrasing components are generated. To overcome these barriers, an emerging Hierarchical Conditional Attention-based Neural Networks (HCANN) architecture to construct end-to-end text generation framework is proposed. More specifically, included method in that represents hierarchy of document along with conditional decoder for paraphrase generation processes. Quantitative evaluation of the method on several benchmark paraphrase datasets demonstrates its efficiency and performance capability by a significant margin.
更多查看译文
关键词
Sequence-to-sequence learning, Hierarchical Conditional Attention-based Neural Networks, Paraphrase generation
AI 理解论文
溯源树
样例
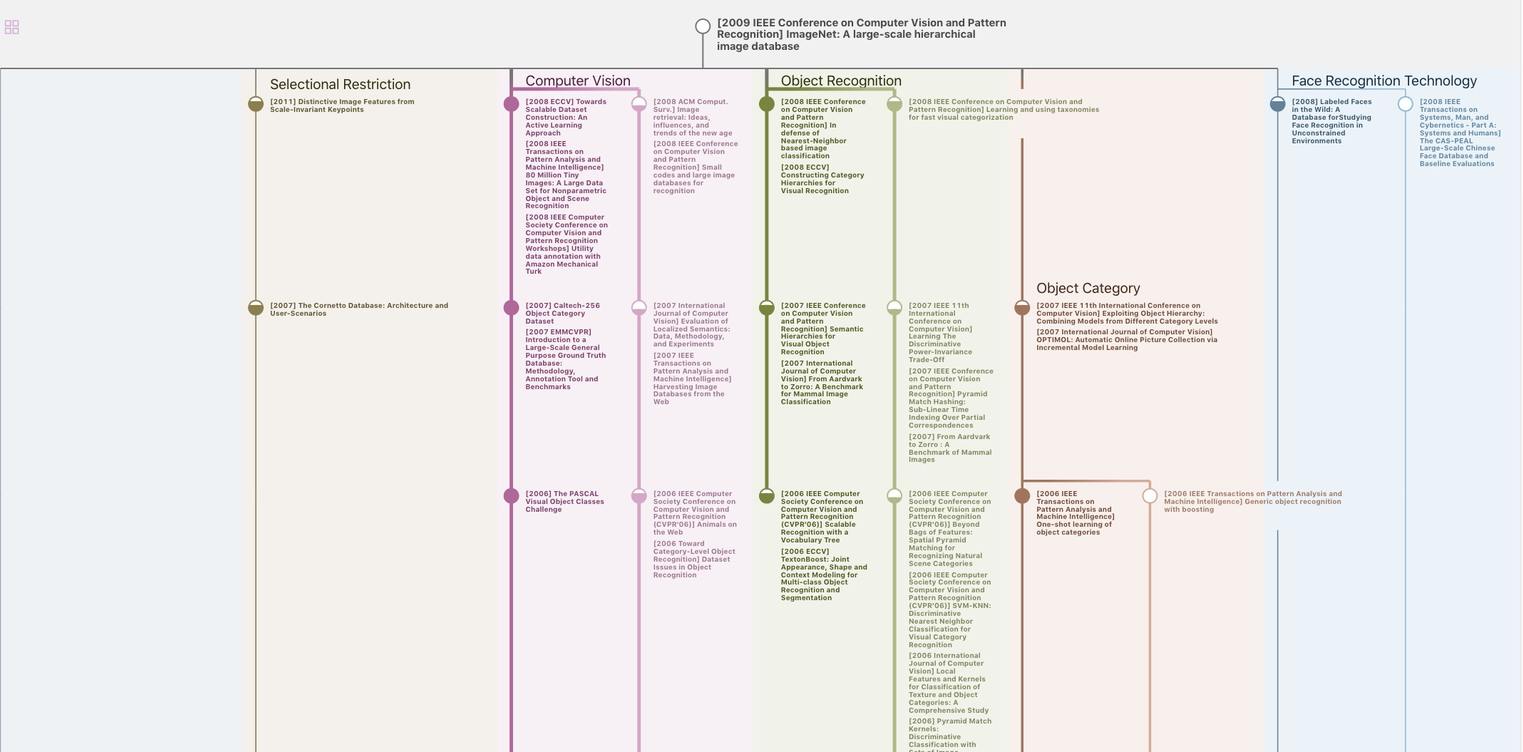
生成溯源树,研究论文发展脉络
Chat Paper
正在生成论文摘要