Semi-Supervised Classification Of Hyperspectral Data For Geologic Body Based On Generative Adversarial Networks At Tianshan Area
IGARSS 2018 - 2018 IEEE INTERNATIONAL GEOSCIENCE AND REMOTE SENSING SYMPOSIUM(2018)
摘要
Hyperspectral remote sensing data contains near continuous spectral information of the object, which is very suitable for mineral classification and geologic body mapping. However, the collecting of a lot of labeled hyperspectral data is expensive, time-consuming and labor-intensive. We choose a semi-supervised method to classify hyperspectral data based on a generative adversarial nertwork (GAN), just use a small amount of labeled data, named HSGAN. The GAN is made up of a generator and a discriminator, and the generator generates data similar to the real data so that the discriminator cannot tell if it is real data or generated data. We designed a one-dimensional GAN to extract spectral features from hyperspectral data. Using this method, we test the Tianshan hyperspectral data and use the actual geological map as the ground-truth produced by us. We find that HSGAN still achieves better results than the traditional CNN and SVM.
更多查看译文
关键词
geological mapping, semi-supervised learning (SSL), generative adversarial networks (GAN), deep learning, Hymap data
AI 理解论文
溯源树
样例
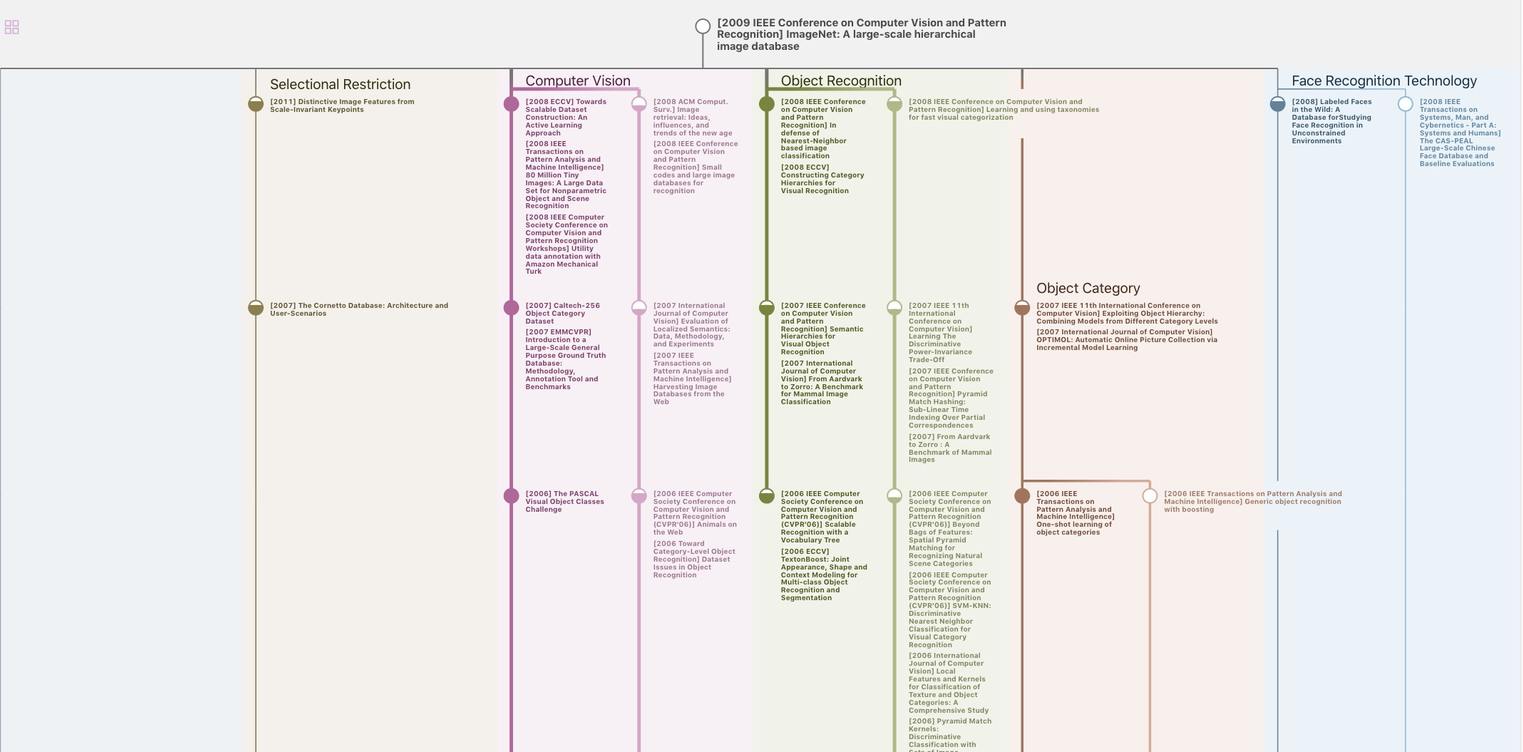
生成溯源树,研究论文发展脉络
Chat Paper
正在生成论文摘要