Discriminative Learning Of Point Cloud Feature Descriptors Based On Siamese Network
IGARSS 2018 - 2018 IEEE INTERNATIONAL GEOSCIENCE AND REMOTE SENSING SYMPOSIUM(2018)
摘要
It is challenging to direct extract the feature descriptors of the object in the point cloud, although deep learning has been widely used with the classification and detection in the point cloud, those methods hidden feature presentation in the network. Since the point cloud scanned by the Laser Scanner usually have different point density, unordered and even the different occlusion, which go beyond the reach of hand-crafted descriptors, e.g. FPH, FPFH, VFH, ROPS. In this paper, we aim to direct extract the feature descriptors of the point cloud object through the raw point cloud. Inspired by the recent success of the Siamese networks[6], PointNet[7] and PointNet++[8], we propose a novel network to direct extract the feature descriptors of the whole point cloud object. We train our network with the Euclidean distance as the loss function which reflects feature descriptors similarity. The experiment object datasets were acquired by Mobile Laser Scanning (MLS) system which contains 6 categories. Experiment result shows that our network has a robust generalization, which can well direct extract the feature descriptors of the whole point cloud object.
更多查看译文
关键词
Point cloud, feature description, mobile laser scanning, siamese network
AI 理解论文
溯源树
样例
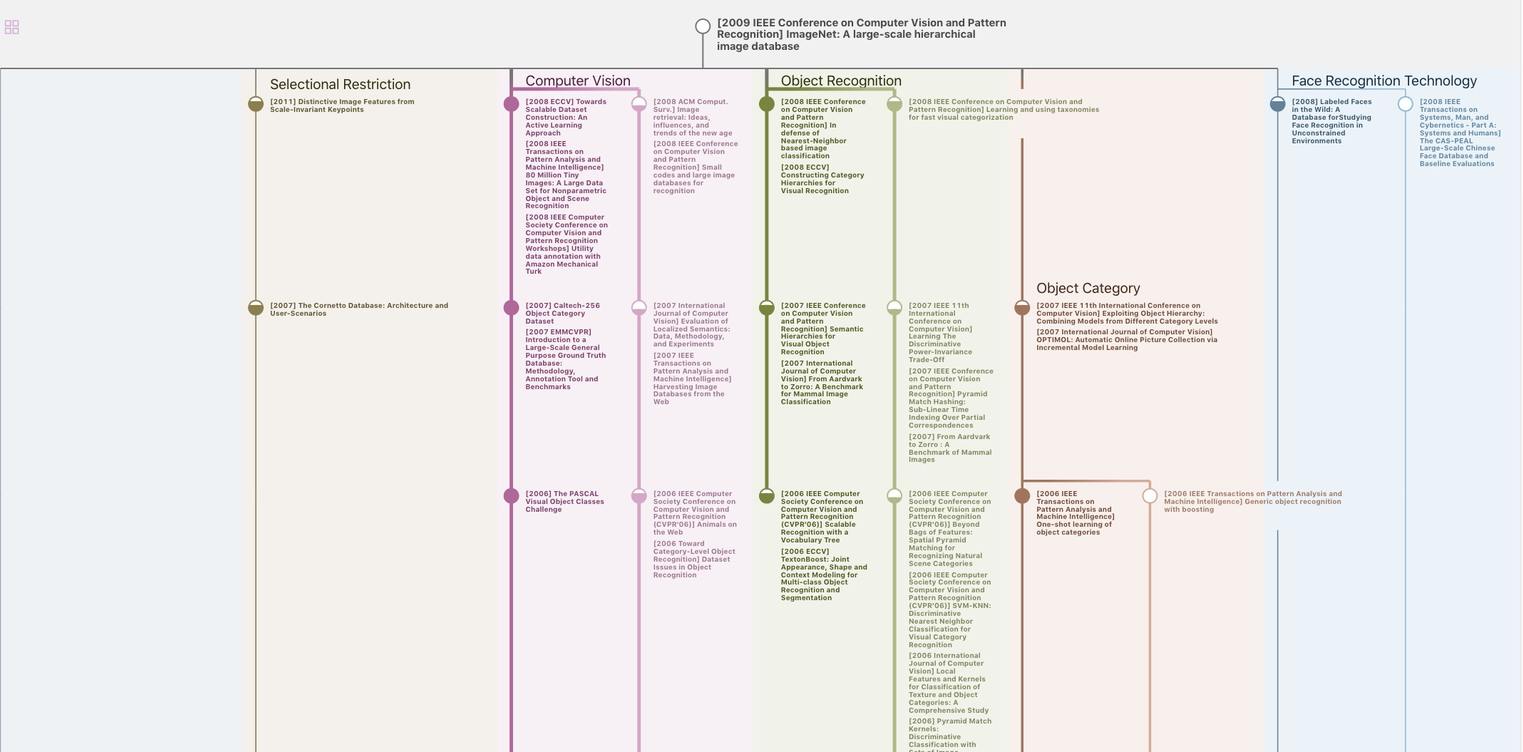
生成溯源树,研究论文发展脉络
Chat Paper
正在生成论文摘要