Semi-Supervised Classification Of Hyperspectral Data Based On Generative Adversarial Networks And Neighborhood Majority Voting
IGARSS 2018 - 2018 IEEE INTERNATIONAL GEOSCIENCE AND REMOTE SENSING SYMPOSIUM(2018)
摘要
How to classify hyperspectral images using few training samples is an important and challenging problem because the collection of the samples is difficult and expensive. Because semi-supervised approaches can utilize information contained in the unlabeled samples and labeled samples, it is a suitable choice. A novel semi-supervised spectral-spatial classification method for hyperspectral data based on generative adversarial network (GAN) is proposed in this paper. First, we use a custom one-dimensional GAN to train the hyperspectral data to obtain spectral features. After using a new small convolutional neural network (CNN) to classify the spectral features, we use a new classification method based on a majority voting strategy further to improve the classification result. The performance of our method is evaluated on ROSIS image data, and the results show that the proposed method can acquire satisfactory results when compared with traditional methods using a few of labeled samples.
更多查看译文
关键词
Hyperspectral images classification, semi-supervised learning (SSL), generative adversarial networks (GAN), deep learning, spectral-spatial classification
AI 理解论文
溯源树
样例
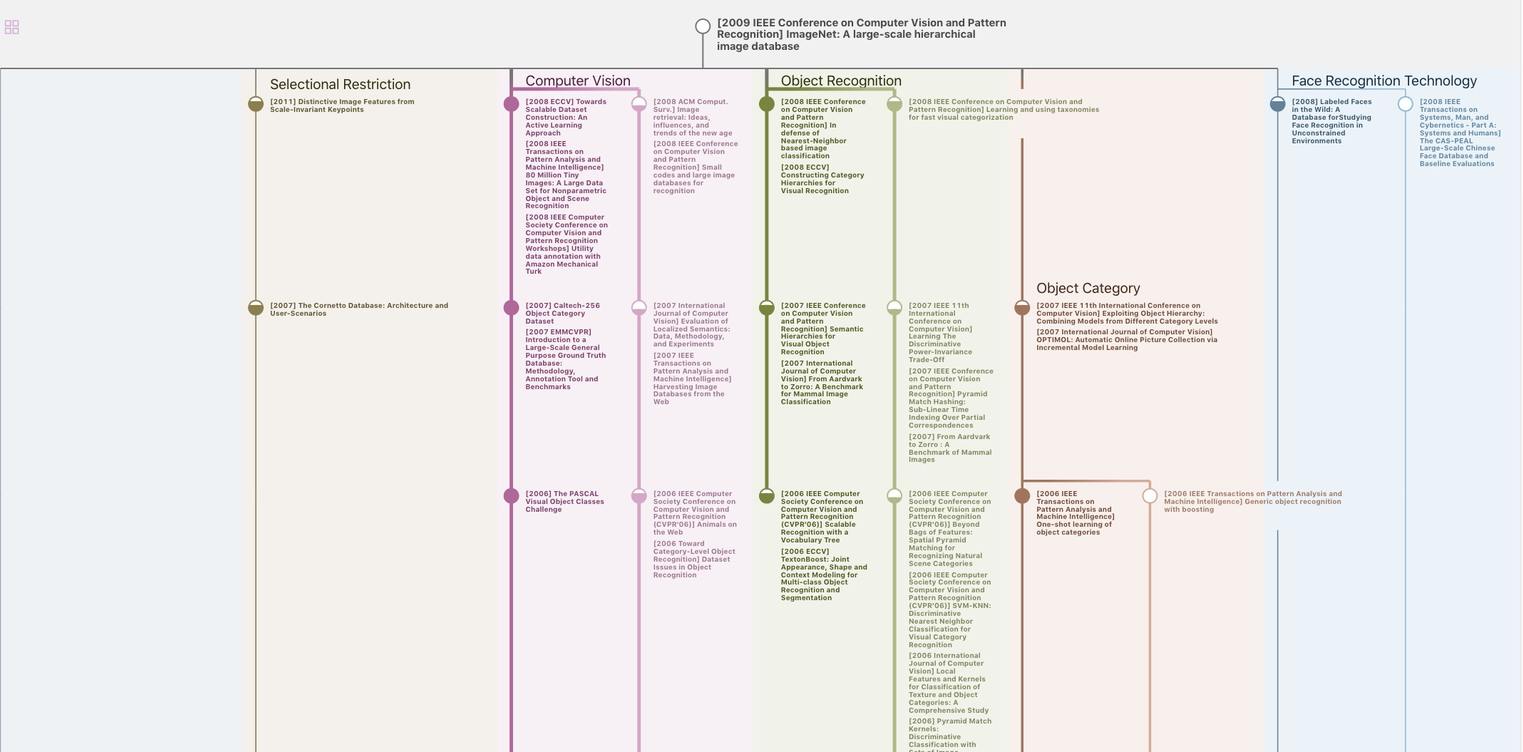
生成溯源树,研究论文发展脉络
Chat Paper
正在生成论文摘要