Residual-Based Video Restoration For Hevc Intra Coding
2018 IEEE FOURTH INTERNATIONAL CONFERENCE ON MULTIMEDIA BIG DATA (BIGMM)(2018)
摘要
Inspired by the great success of convolutional neural network (CNN) in computer vision, CNN-based post-processing methods at the decoder side have achieved significant advances in improving the video quality. However, these methods only learn a mapping from the decoded frame to an artifact-free reconstruction, which ignores that the distortion comes from quantization of the prediction residual transform coefficients. In this paper, we propose a residual-based video restoration network (Residual-VRN) to improve the quality of decoded video, in which the coded prediction residual is combined with the prediction frame as the input of the network. Meanwhile, the activation function, the residual learning framework and the loss function can also be optimized to achieve better quality enhancement. Experimental results show that the proposed Residual-VRN leads to an average 7.41% BD-rate reduction compared to the HEVC intra coding baseline, outperforming the conventional CNN-based video restoration algorithms. In deeper CNN architectures, our method achieves 8.0%, 9.0% and 11.1% BD-rate savings, much higher than the conventional CNN-based post-processing methods.
更多查看译文
关键词
Decoder-side enhancement, Artifact reduction, Convolutional neural network (CNN), High Efficiency Video Coding (HEVC), Intra coding, Post-processing
AI 理解论文
溯源树
样例
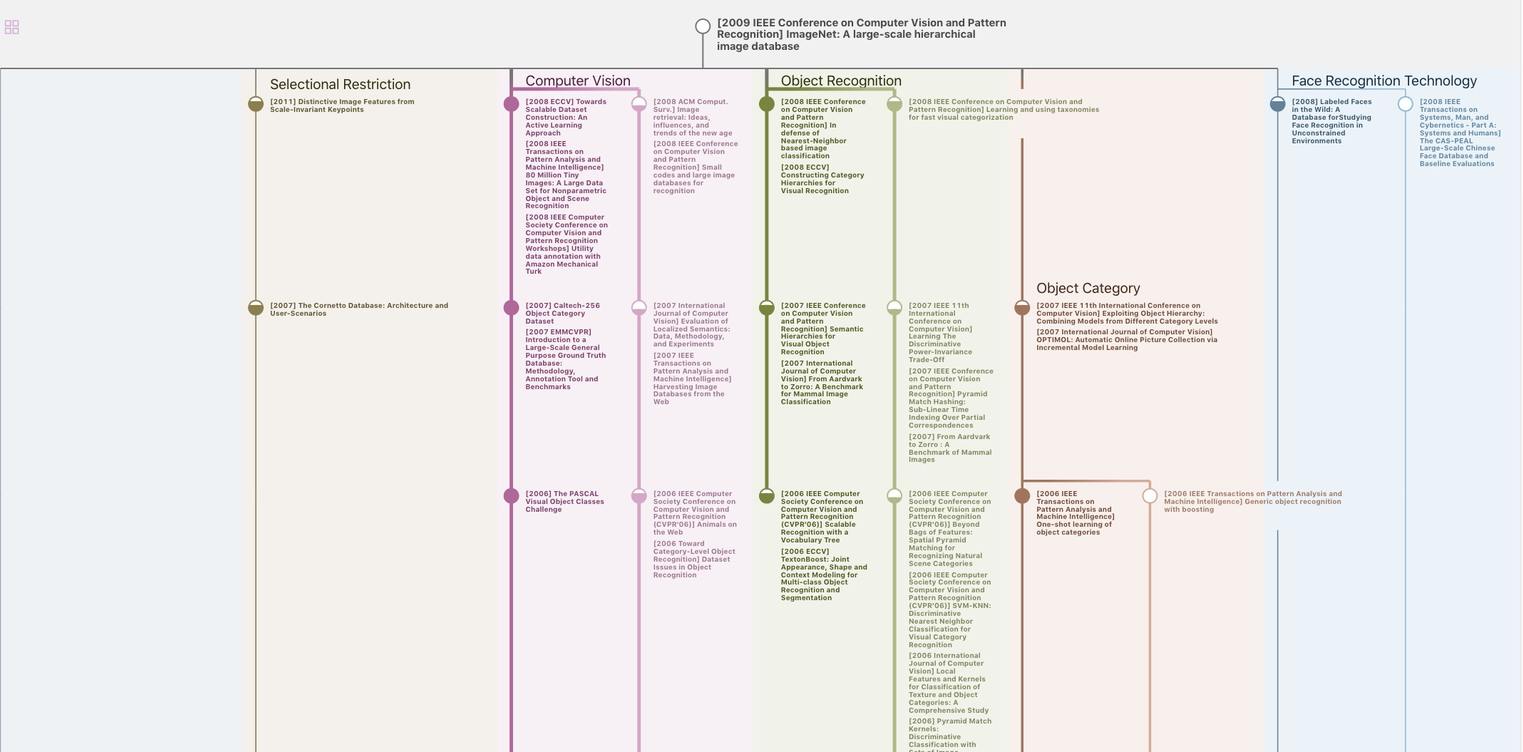
生成溯源树,研究论文发展脉络
Chat Paper
正在生成论文摘要