A Bayesian parametric model for quantifying brain maturation from sleep-EEG in the vulnerable newborn baby
2018 40th Annual International Conference of the IEEE Engineering in Medicine and Biology Society (EMBC)(2018)
摘要
Newborn babies, particularly preterms, can exhibit early deviations in sleep maturation as seen by Electroencephalogram (EEG) recordings. This may be indicative of cognitive problems by school-age. The current `clinically-driven' approach uses separate algorithms to first extract sleep states and then predict EEG `brain-age'. Maturational deviations are identified when the brain-age no longer matches the Postmenstrual Age (PMA, the age since the last menstrual cycle of the mother). However, the PMA range where existing sleep staging algorithms perform optimally, is limited, which subsequently limits the PMA range for brain-age prediction. We introduce a Bayesian Parametric Model (BPM) as a single end-to-end solution to directly estimate brain-age, modelling for sleep state maturation without requiring a separately optimized sleep staging algorithm. Comparison of this model with a traditional multi-stage approach, yields a similar Krippendorff's α = 0.92 (a performance measure ranging from 0 (chance agreement) to 1 (perfect agreement)) with the BPM performing better at younger ages <;30 weeks PMA. The BPM's potential to detect maturational deviations is also explored on a few preterm babies who were abnormal at 9 months follow-up.
更多查看译文
关键词
Algorithms,Bayes Theorem,Brain,Electroencephalography,Female,Humans,Infant,Infant, Newborn,Infant, Premature,Sleep,Sleep Stages
AI 理解论文
溯源树
样例
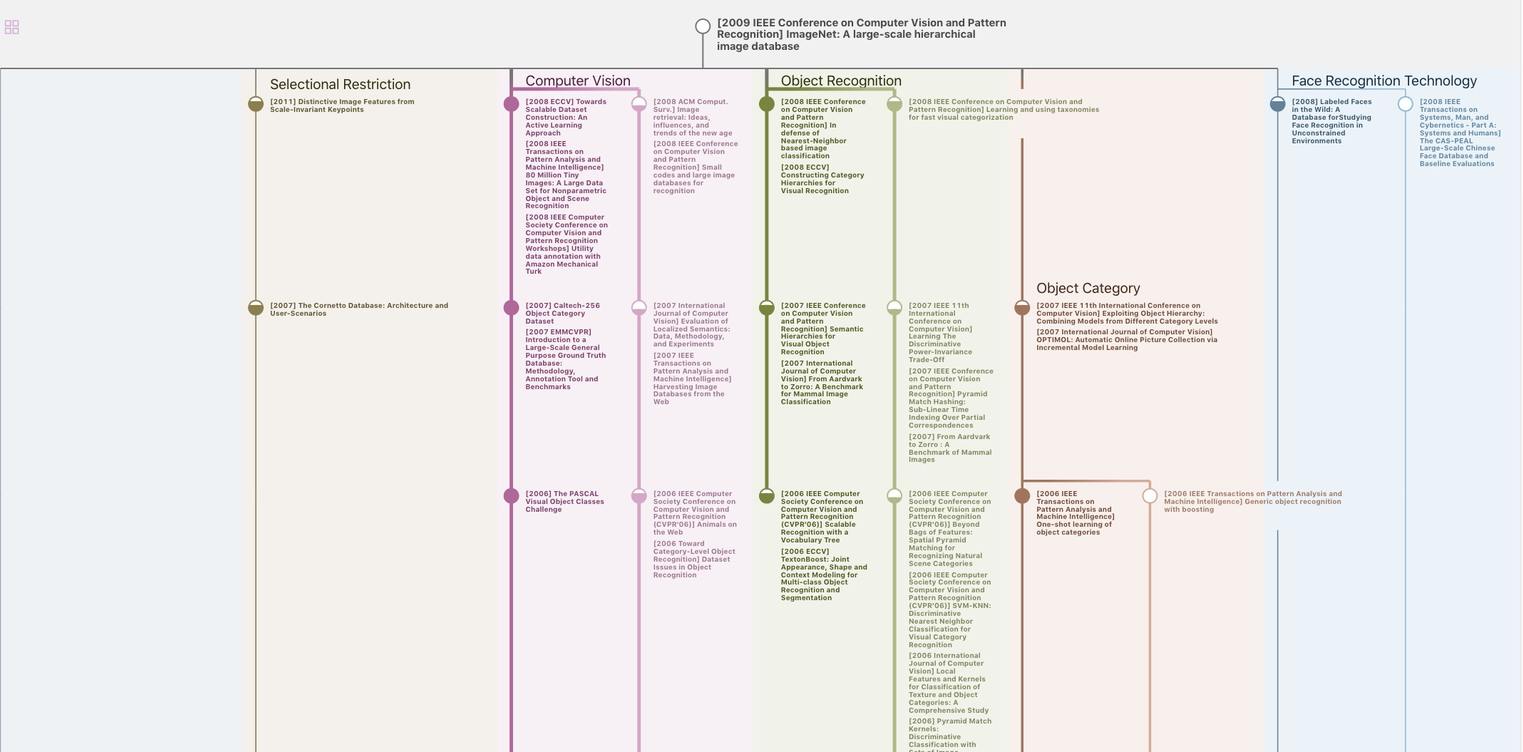
生成溯源树,研究论文发展脉络
Chat Paper
正在生成论文摘要