The iNaturalist Species Classification and Detection Dataset
2018 IEEE/CVF CONFERENCE ON COMPUTER VISION AND PATTERN RECOGNITION (CVPR)(2018)
摘要
Existing image classification datasets used in computer vision tend to have a uniform distribution of images across object categories. In contrast, the natural world is heavily imbalanced, as some species are more abundant and easier to photograph than others. To encourage further progress in challenging real world conditions we present the iNaturalist species classification and detection dataset, consisting of 859,000 images from over 5,000 different species of plants and animals. It features visually similar species, captured in a wide variety of situations, from all over the world. Images were collected with different camera types, have varying image quality, feature a large class imbalance, and have been verified by multiple citizen scientists. We discuss the collection of the dataset and present extensive baseline experiments using state-of-the-art computer vision classification and detection models. Results show that current non-ensemble based methods achieve only 67% top one classification accuracy, illustrating the difficulty of the dataset. Specifically, we observe poor results for classes with small numbers of training examples suggesting more attention is needed in low-shot learning.
更多查看译文
关键词
image classification datasets,image quality,low-shot learning,iNaturalist species classification,computer vision classification
AI 理解论文
溯源树
样例
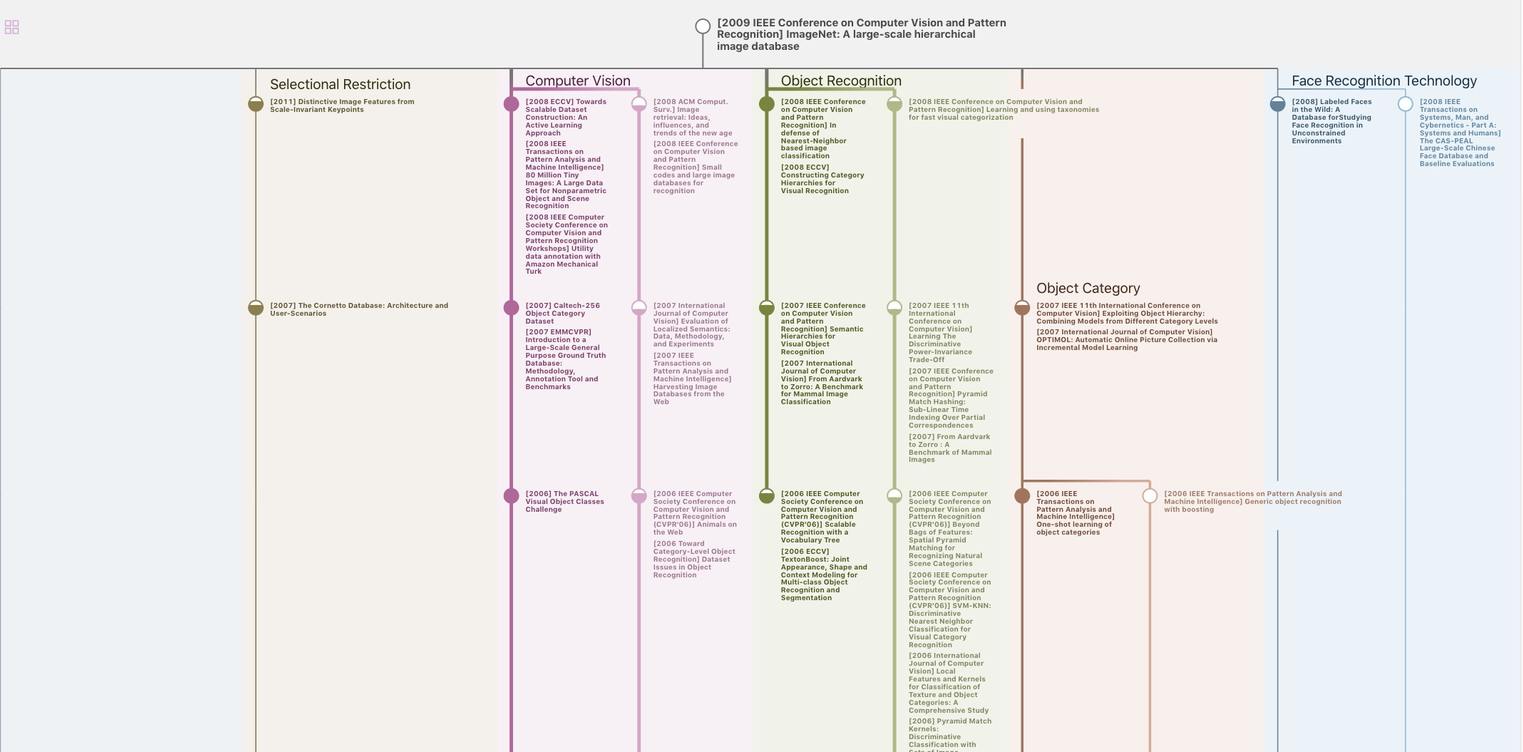
生成溯源树,研究论文发展脉络
Chat Paper
正在生成论文摘要