Classification Of Radiology Reports By Modality And Anatomy: A Comparative Study
PROCEEDINGS 2018 IEEE INTERNATIONAL CONFERENCE ON BIOINFORMATICS AND BIOMEDICINE (BIBM)(2018)
摘要
Data labeling is currently a time-consuming task that often requires expert knowledge. In research settings, the availability of correctly labeled data is crucial to ensure that model predictions are accurate and useful. We propose relatively simple machine learning-based models that achieve high performance metrics in the binary and multiclass classification of radiology reports. We compare the performance of these algorithms to that of a data-driven approach based on NLP, and find that the logistic regression classifier outperforms all other models, in both the binary and multiclass classification tasks. We then choose the logistic regression binary classifier to predict chest X-ray (CXR)/non-chest X-ray (non-CXR) labels in reports from different datasets, unseen during any training phase of any of the models. Even in unseen report collections, the binary logistic regression classifier achieves average precision values of above 0.9. Based on the regression coefficient values, we also identify frequent tokens in CXR and non-CXR reports that are features with possibly high predictive power.
更多查看译文
关键词
text classification, machine learning, logistic regression, SVM, NLP
AI 理解论文
溯源树
样例
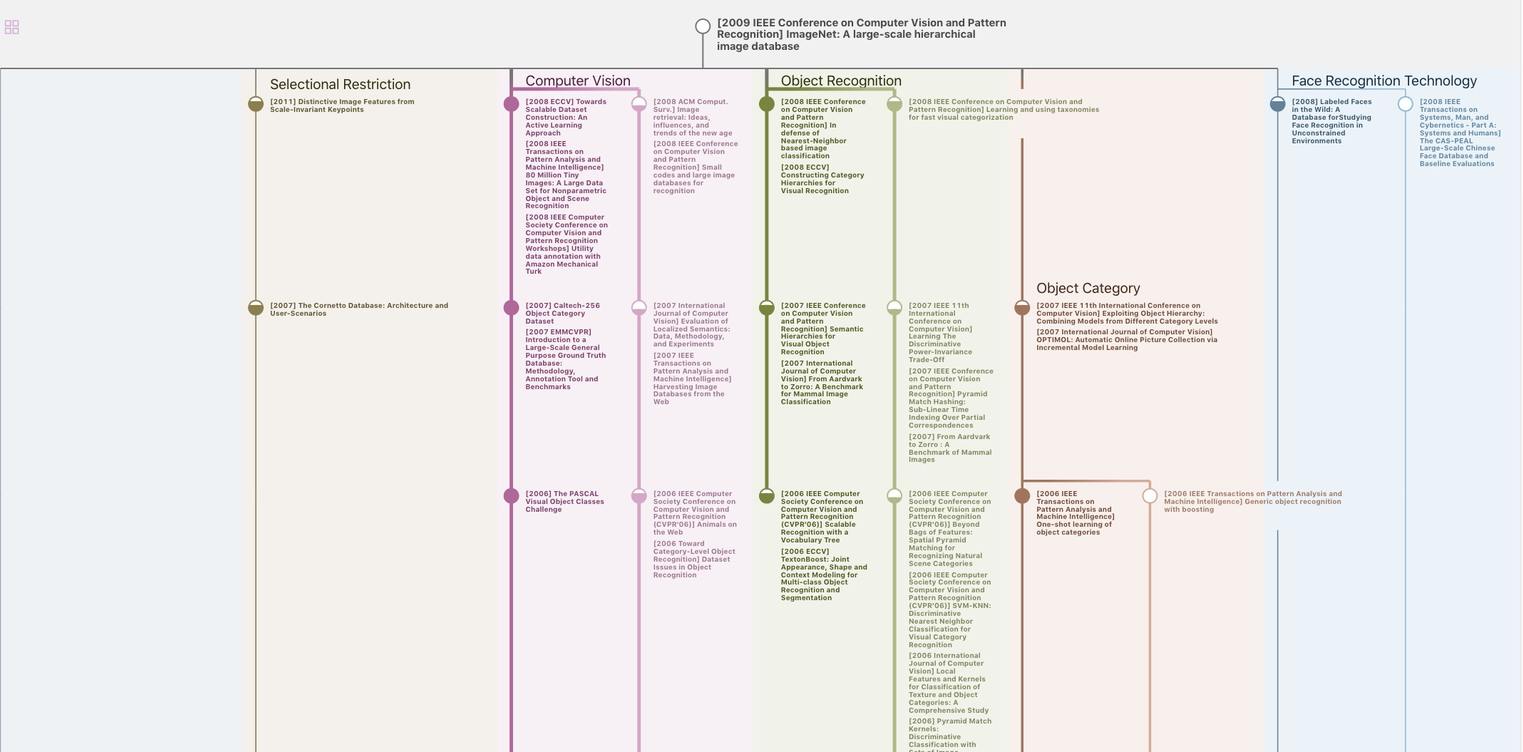
生成溯源树,研究论文发展脉络
Chat Paper
正在生成论文摘要