An abstract proximal point algorithm
J. Global Optimization(2018)
Abstract
The proximal point algorithm is a widely used tool for solving a variety of convex optimization problems such as finding zeros of maximally monotone operators, fixed points of nonexpansive mappings, as well as minimizing convex functions. The algorithm works by applying successively so-called “resolvent” mappings associated to the original object that one aims to optimize. In this paper we abstract from the corresponding resolvents employed in these problems the natural notion of jointly firmly nonexpansive families of mappings. This leads to a streamlined method of proving weak convergence of this class of algorithms in the context of complete CAT(0) spaces (and hence also in Hilbert spaces). In addition, we consider the notion of uniform firm nonexpansivity in order to similarly provide a unified presentation of a case where the algorithm converges strongly. Methods which stem from proof mining, an applied subfield of logic, yield in this situation computable and low-complexity rates of convergence.
MoreTranslated text
Key words
Convex optimization,Proximal point algorithm,CAT(0) spaces,Jointly firmly nonexpansive families,Uniformly firmly nonexpansive mappings,Proof mining,Rates of convergence,90C25,46N10,47J25,47H09,03F10
AI Read Science
Must-Reading Tree
Example
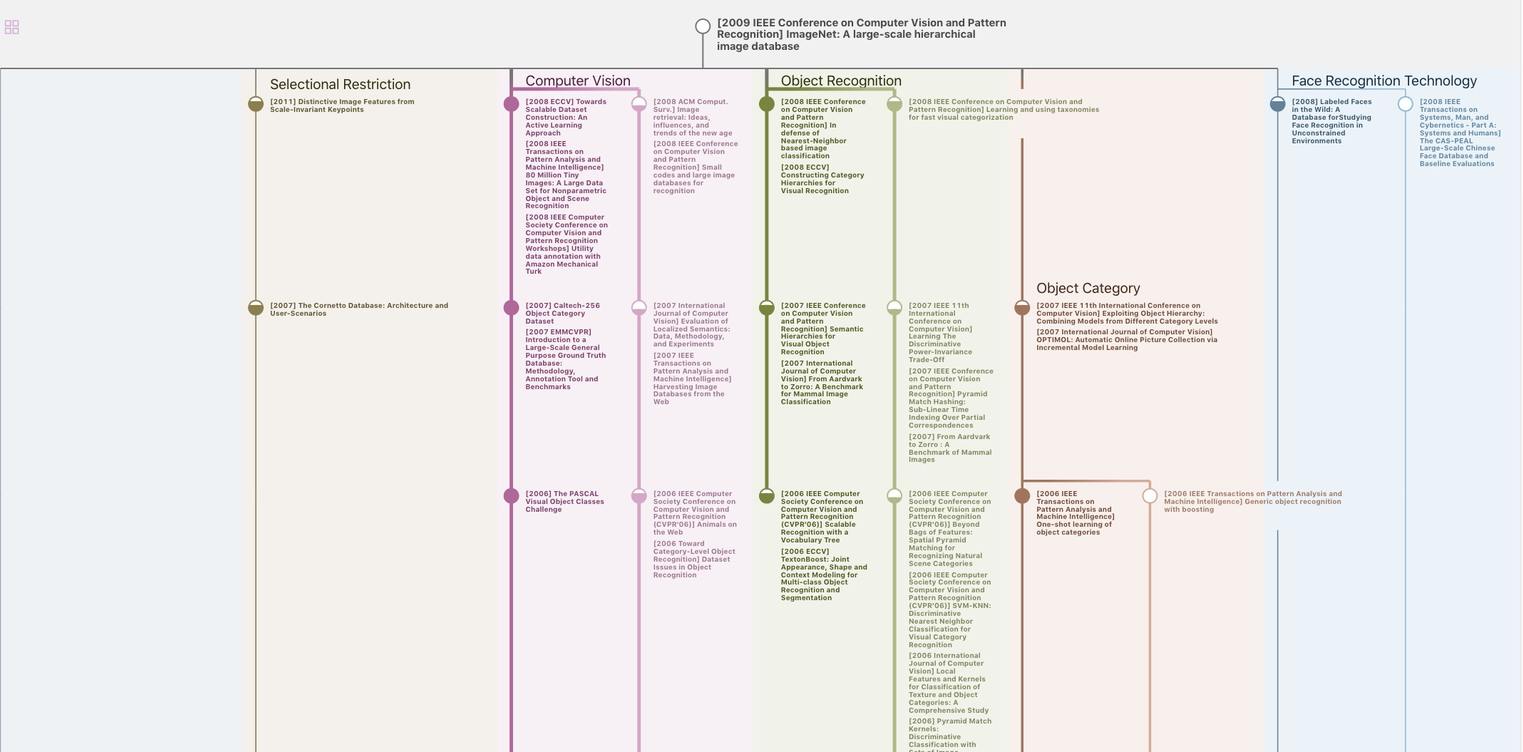
Generate MRT to find the research sequence of this paper
Chat Paper
Summary is being generated by the instructions you defined