Listening for Sirens: Locating and Classifying Acoustic Alarms in City Scenes
arXiv: Sound(2022)
摘要
This paper is about acoustic event detection and sound source localisation in urban scenarios. Specifically, we are interested in detecting and localising horns and sirens of emergency vehicles. Urban scenarios, though, can be characterised by copious, unstructured and unpredictable traffic noise, which can severely compromise the performance and effectiveness of traditional filtering techniques. By analysing the spectrograms of incoming stereo signals as images, we can leverage image processing techniques and obtain a demonstrably robust system. Indeed, image processing methods, such as convolutional neural networks, which do not operate locally, offer interesting mechanisms for background foreground separation. When applied to spectrograms, those mechanisms allow using the entire context of the soundscape to discover and learn correlations both in the time and frequency domains, de facto implementing noise detection through semantic segmentation. In a multi-task learning scheme, together with signal denoising, we perform acoustic event classification to identify the nature of the alerting sound. Lastly, we use the denoised signals to localise the acoustic source on the ground plane, by regressing the direction of arrival of the sound. Our experimental evaluation shows an average classification rate of 94%, and a median absolute error on the localisation of 7.5 degrees when operating on audio frames of 0.5 s, and of 2.5 degrees when operating on frames of 2.5 s. The system offers excellent performance in particularly challenging scenarios, where the noise level is remarkably high.
更多查看译文
关键词
Acoustics,Signal to noise ratio,Spectrogram,Image segmentation,Direction-of-arrival estimation,Multitasking,Deep learning,Acoustic event classification,siren detection,semantic segmentation,smart vehicles,deep learning
AI 理解论文
溯源树
样例
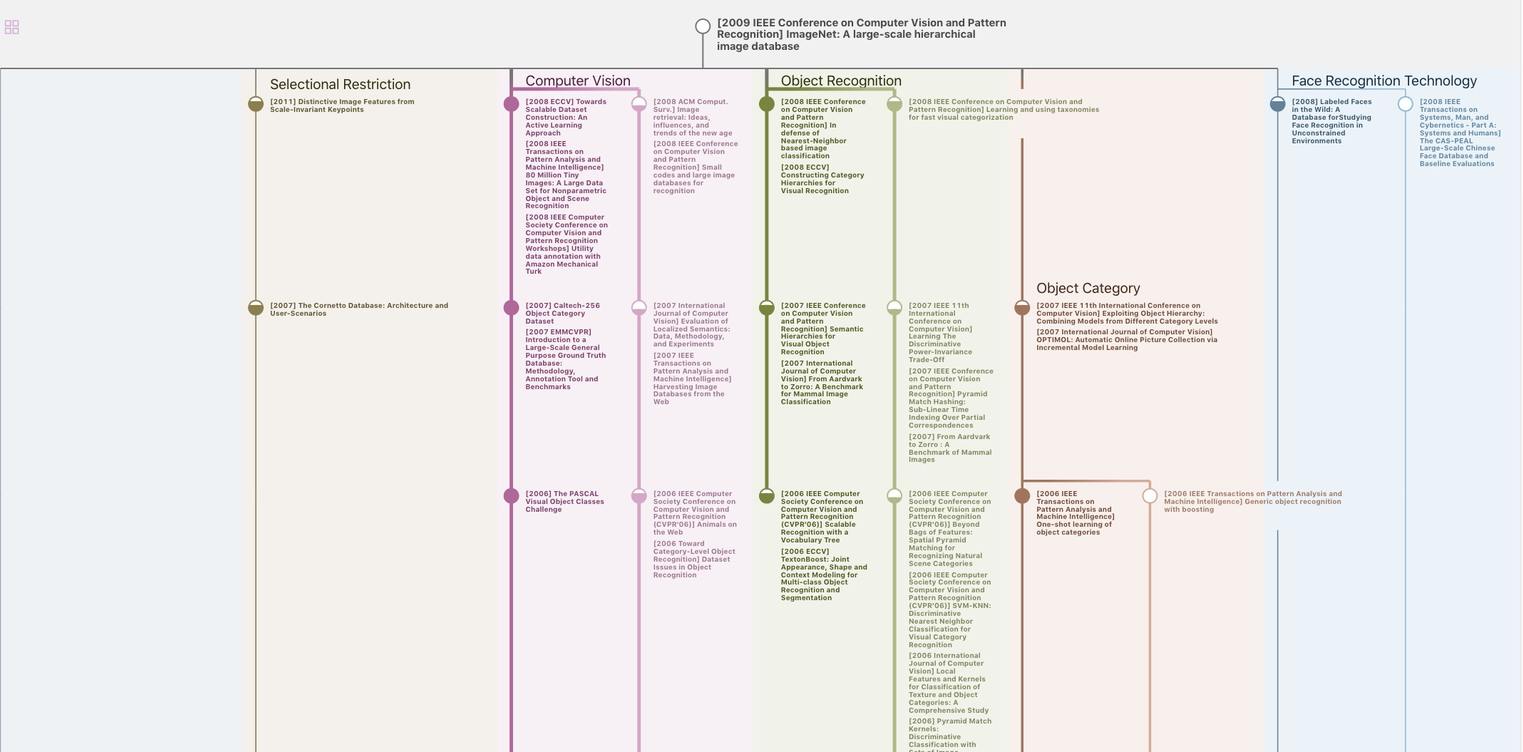
生成溯源树,研究论文发展脉络
Chat Paper
正在生成论文摘要