Deep Learning: Extrapolation Tool for Ab Initio Nuclear Theory.
PHYSICAL REVIEW C(2019)
摘要
Ab initio approaches in nuclear theory, such as the no-core shell model (NCSM), have been developed for approximately solving finite nuclei with realistic strong interactions. The NCSM and other approaches require an extrapolation of the results obtained in a finite basis space to the infinite basis space limit and assessment of the uncertainty of those extrapolations. Each observable requires a separate extrapolation and many observables have no proven extrapolation method. We propose a feed-forward artificial neural network (ANN) method as an extrapolation tool to obtain the ground-state energy and the ground-state point-proton root-mean-square (rms) radius along with their extrapolation uncertainties. The designed ANNs are sufficient to produce results for these two very different observables in Li-6 from the ab initio NCSM results in small basis spaces that satisfy the following theoretical physics condition: independence of basis space parameters in the limit of extremely large matrices. Comparisons of the ANN results with other extrapolation methods are also provided.
更多查看译文
关键词
Mean-Field Models
AI 理解论文
溯源树
样例
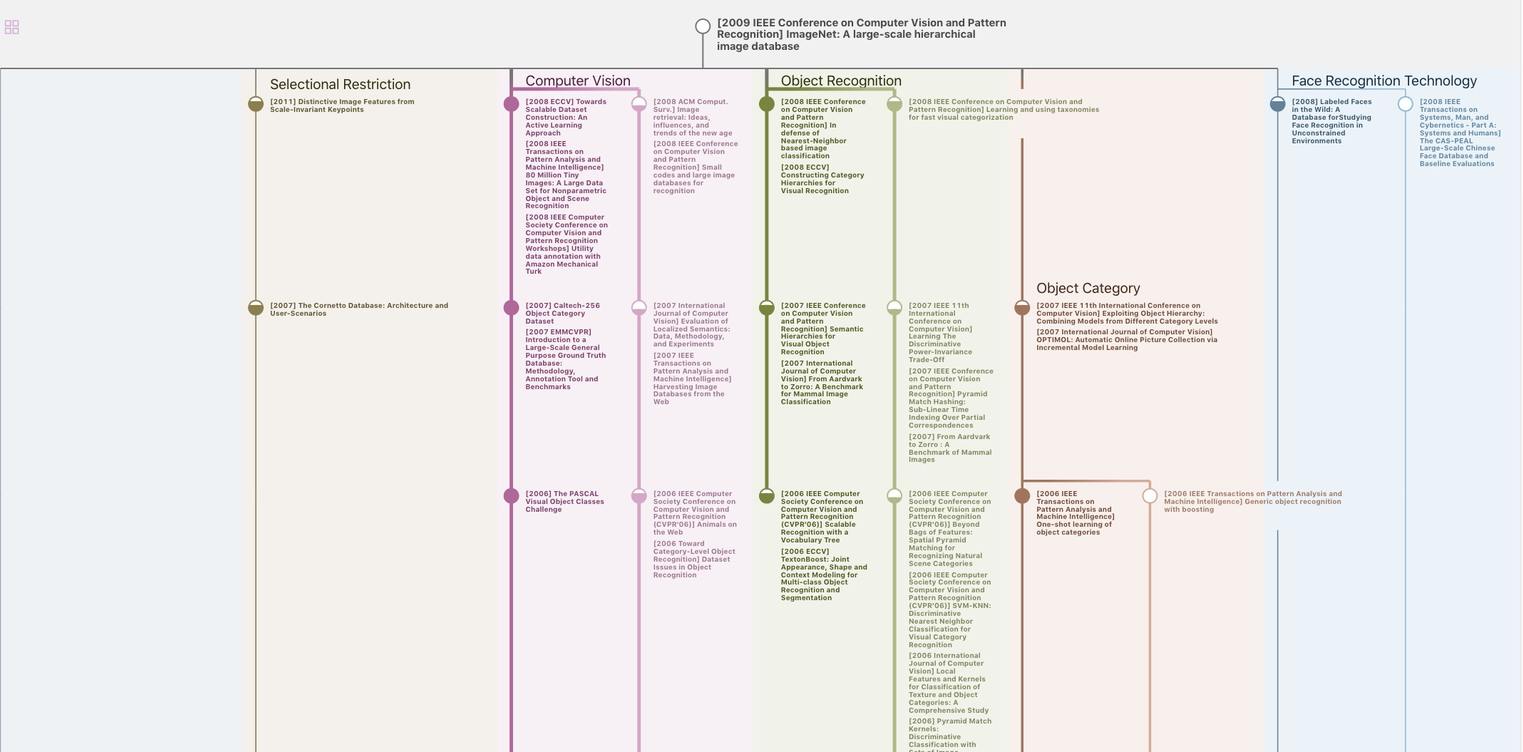
生成溯源树,研究论文发展脉络
Chat Paper
正在生成论文摘要