A Minimum Discounted Reward Hamilton-Jacobi Formulation for Computing Reachable Sets
IEEE TRANSACTIONS ON AUTOMATIC CONTROL(2024)
摘要
We propose a novel formulation for approximating reachable sets through a minimum discounted reward optimal control problem. The formulation yields a continuous solution that can be obtained by solving a Hamilton-Jacobi equation. Furthermore, the numerical approximation to this solution is the unique fixed-point to a contraction mapping. This allows for more efficient solution methods that are not applicable under traditional formulations for solving reachable sets. Lastly, this formulation provides a link between reinforcement learning and learning reachable sets for systems with unknown dynamics, allowing algorithms from the former to be applied to the latter. We use two benchmark examples, double integrator, and pursuit-evasion games, to show the correctness of the formulation as well as its strengths in comparison to previous work.
更多查看译文
关键词
Trajectory,Games,Infinite horizon,Convergence,Q measurement,Viscosity,Standards,Approximate reachability,machine learning,reachability analysis,safety analysis
AI 理解论文
溯源树
样例
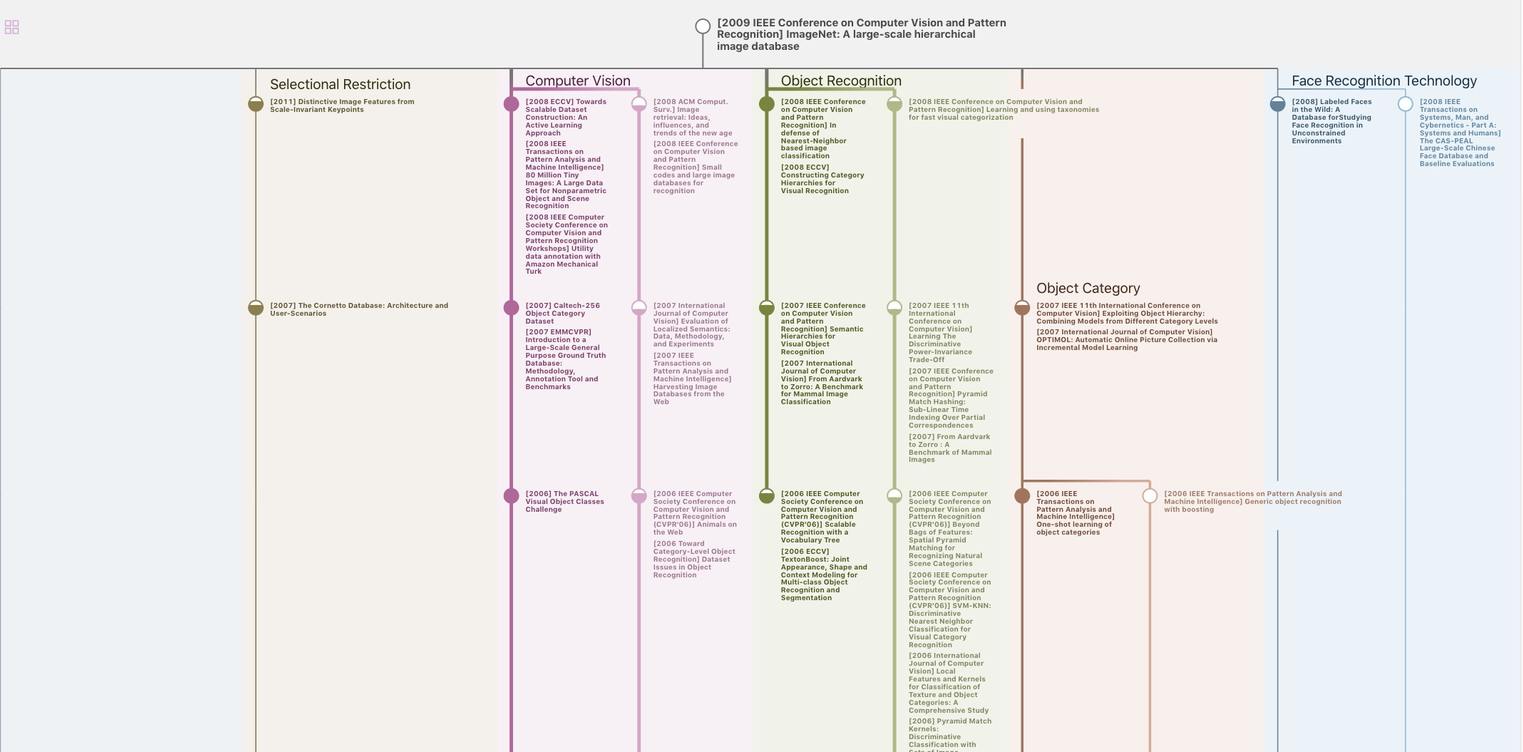
生成溯源树,研究论文发展脉络
Chat Paper
正在生成论文摘要