Gradient-based Representational Similarity Analysis with Searchlight for Analyzing fMRI Data
Pattern Recognition and Computer Vision: First Chinese Conference, PRCV 2018, Guangzhou, China, November 23-26, 2018, Proceedings, Part III(2018)
摘要
Representational Similarity Analysis (RSA) aims to explore similarities between neural activities of different stimuli. Classical RSA techniques employ the inverse of the covariance matrix to explore a linear model between the neural activities and task events. However, calculating the inverse of a large-scale covariance matrix is time-consuming and can reduce the stability and robustness of the final analysis. Notably, it becomes severe when the number of samples is too large. For facing this shortcoming, this paper proposes a novel RSA method called gradient-based RSA (GRSA). Moreover, the proposed method is not restricted to a linear model. In fact, there is a growing interest in finding more effective ways of using multi-subject and whole-brain fMRI data. Searchlight technique can extend RSA from the localized brain regions to the whole-brain regions with smaller memory footprint in each process. Based on Searchlight, we propose a new method called Spatiotemporal Searchlight GRSA (SSL-GRSA) that generalizes our ROI-based GRSA algorithm to the whole-brain data. Further, our approach can handle some computational challenges while dealing with large-scale, multi-subject fMRI data. Experimental studies on multi-subject datasets confirm that both proposed approaches achieve superior performance to other state-of-the-art RSA algorithms.
更多查看译文
关键词
RSA,Gradient,Searchlight,Whole-brain fMRI data
AI 理解论文
溯源树
样例
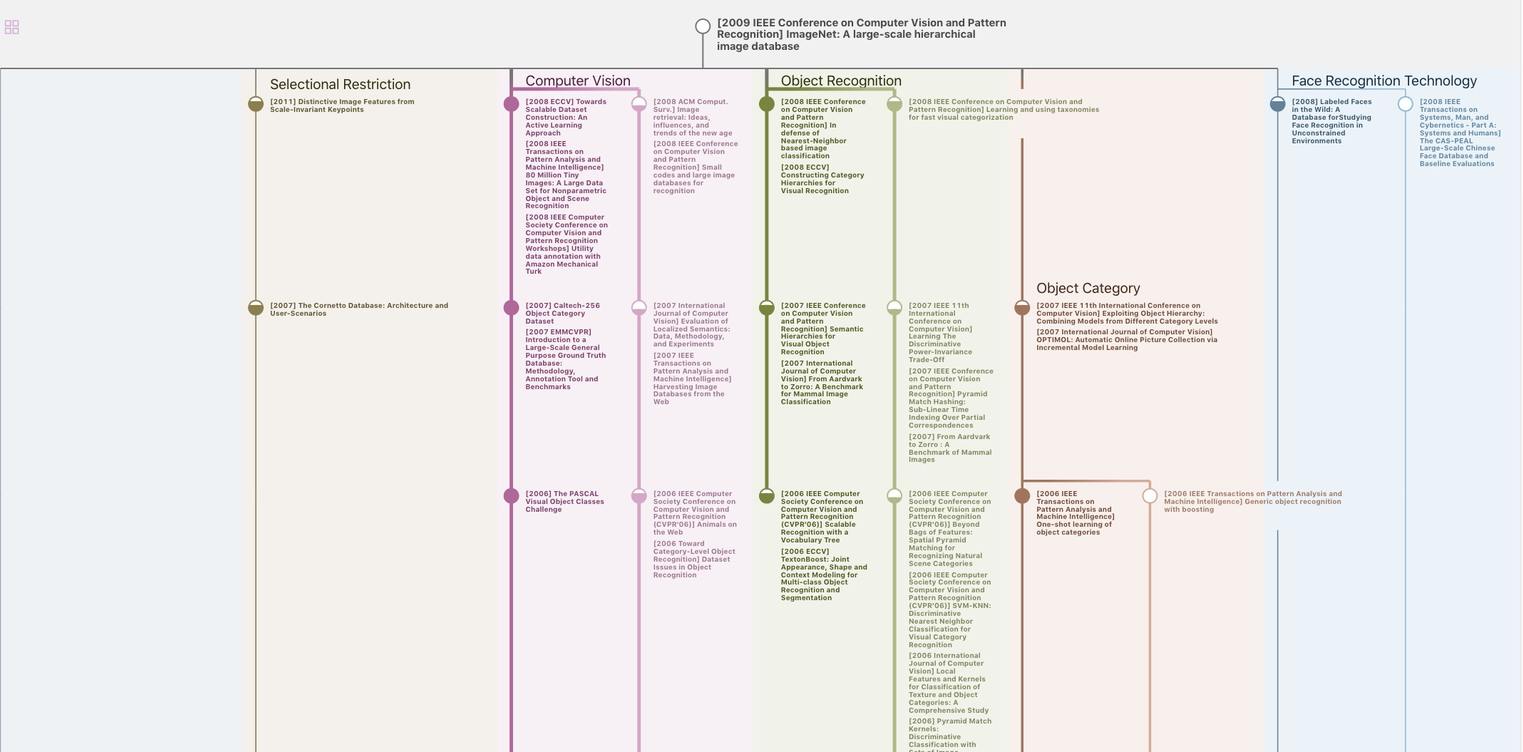
生成溯源树,研究论文发展脉络
Chat Paper
正在生成论文摘要