Fast Context Adaptation via Meta-Learning
arXiv: Learning(2019)
Abstract
We propose CAVIA, a meta-learning method for fast adaptation that is scalable, flexible, and easy to implement. CAVIA partitions the model parameters into two parts: context parameters that serve as additional input to the model and are adapted on individual tasks, and shared parameters that are meta-trained and shared across tasks. At test time, the context parameters are updated with one or several gradient steps on a task-specific loss that is backpropagated through the shared part of the network. Compared to approaches that adjust all parameters on a new task (e.g., MAML), CAVIA can be scaled up to larger networks without overfitting on a single task, is easier to implement, and is more robust to the inner-loop learning rate. We show empirically that CAVIA outperforms MAML on regression, classification, and reinforcement learning problems.
MoreTranslated text
Key words
fast context adaptation,meta-learning
AI Read Science
Must-Reading Tree
Example
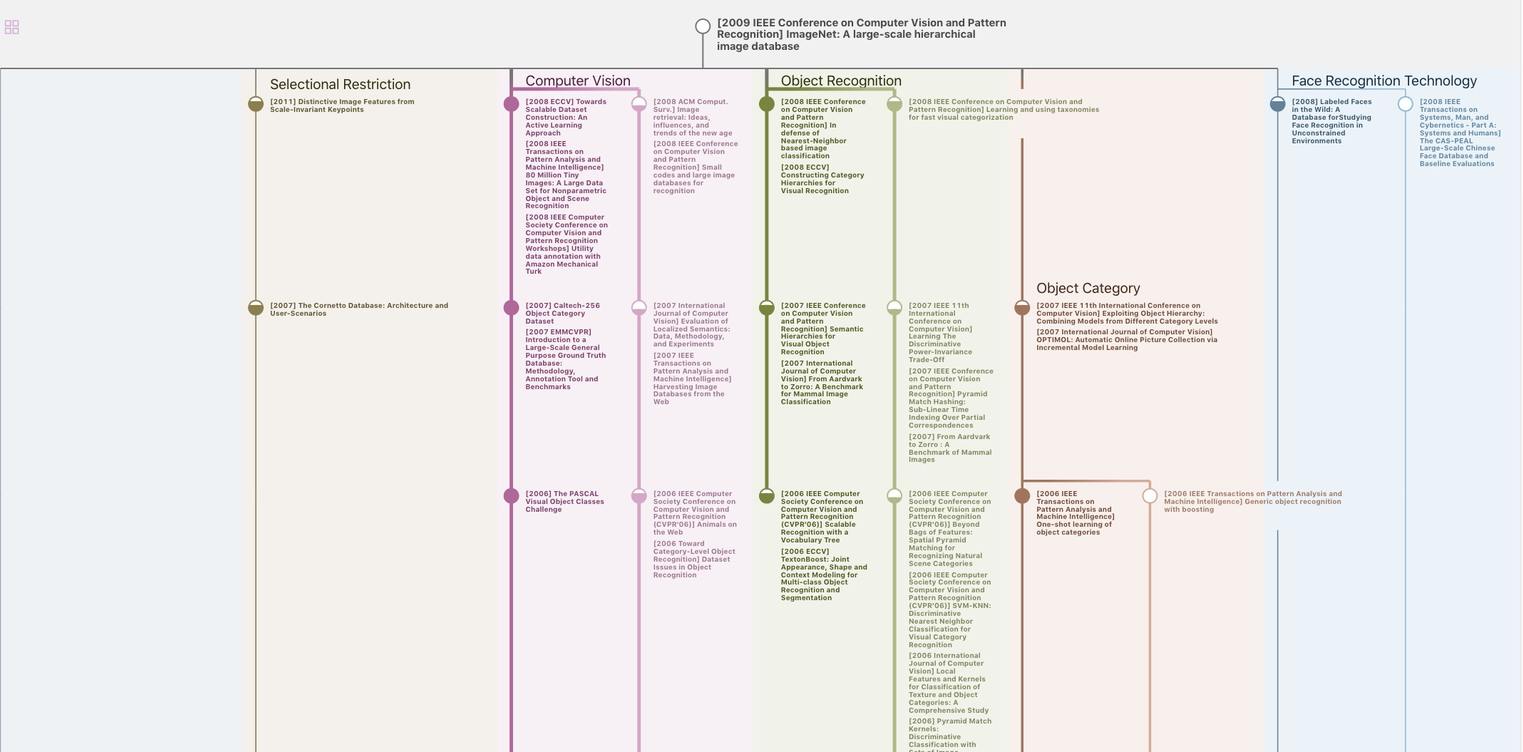
Generate MRT to find the research sequence of this paper
Chat Paper
Summary is being generated by the instructions you defined