On Statistical Learning of Simplices: Unmixing Problem Revisited
Annals of Statistics(2019)
Abstract
Learning of a high-dimensional simplex from uniformly-sampled observations, generally known as the "unmixing problem", is a long-studied task in computer science. More recently, a significant interest is focused on this problem from other areas, such as computational biology and remote sensing. In this paper, we have studied the Probably Approximately Correct (PAC)-learnability of simplices in terms of sample complexity. Our analysis shows that a sufficient sample size for reliable learning of a $K$-simplex is only $O\left(K^2\log K\right)$, which yields a significant improvement over the current bound, i.e. $O\left(K^{22}\right)$. In particular, we propose a continuous-relaxation for Maximum Likelihood estimator which comes with a certificate of PAC-learnability, and is also followed by a scalable algorithm whose performance is extensively tested on synthetic and real-world datasets. Experimental results show that not only being comparable to rival methods on noiseless samples, our framework is superior to the state-of-the-art in noisy cases.
MoreTranslated text
AI Read Science
Must-Reading Tree
Example
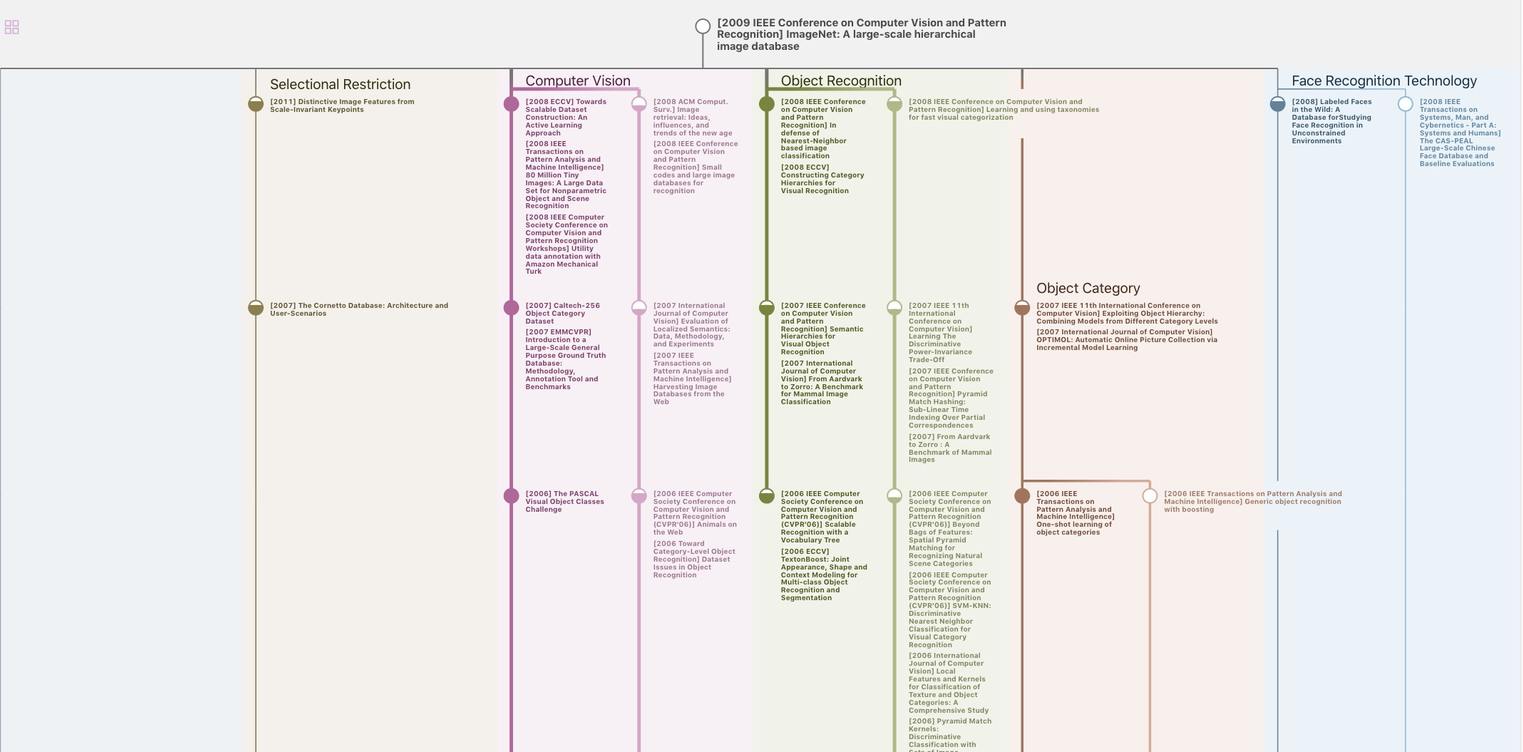
Generate MRT to find the research sequence of this paper
Chat Paper
Summary is being generated by the instructions you defined