Learning with Random Learning Rates.
arXiv: Learning(2018)
摘要
Hyperparameter tuning is a bothersome step in the training of deep learning models. One of the most sensitive hyperparameters is the learning rate of the gradient descent. We present the u0027All Learning Rates At Onceu0027 (Alrao) optimization method for neural networks: each unit or feature in the network gets its own learning rate sampled from a random distribution spanning several orders of magnitude. This comes at practically no computational cost. Perhaps surprisingly, stochastic gradient descent (SGD) with Alrao performs close to SGD with an optimally tuned learning rate, for various architectures and problems. Alrao could save time when testing deep learning models: a range of models could be quickly assessed with Alrao, and the most promising models could then be trained more extensively. This text comes with a PyTorch implementation of the method, which can be plugged on an existing PyTorch model: this https URL .
更多查看译文
关键词
learning
AI 理解论文
溯源树
样例
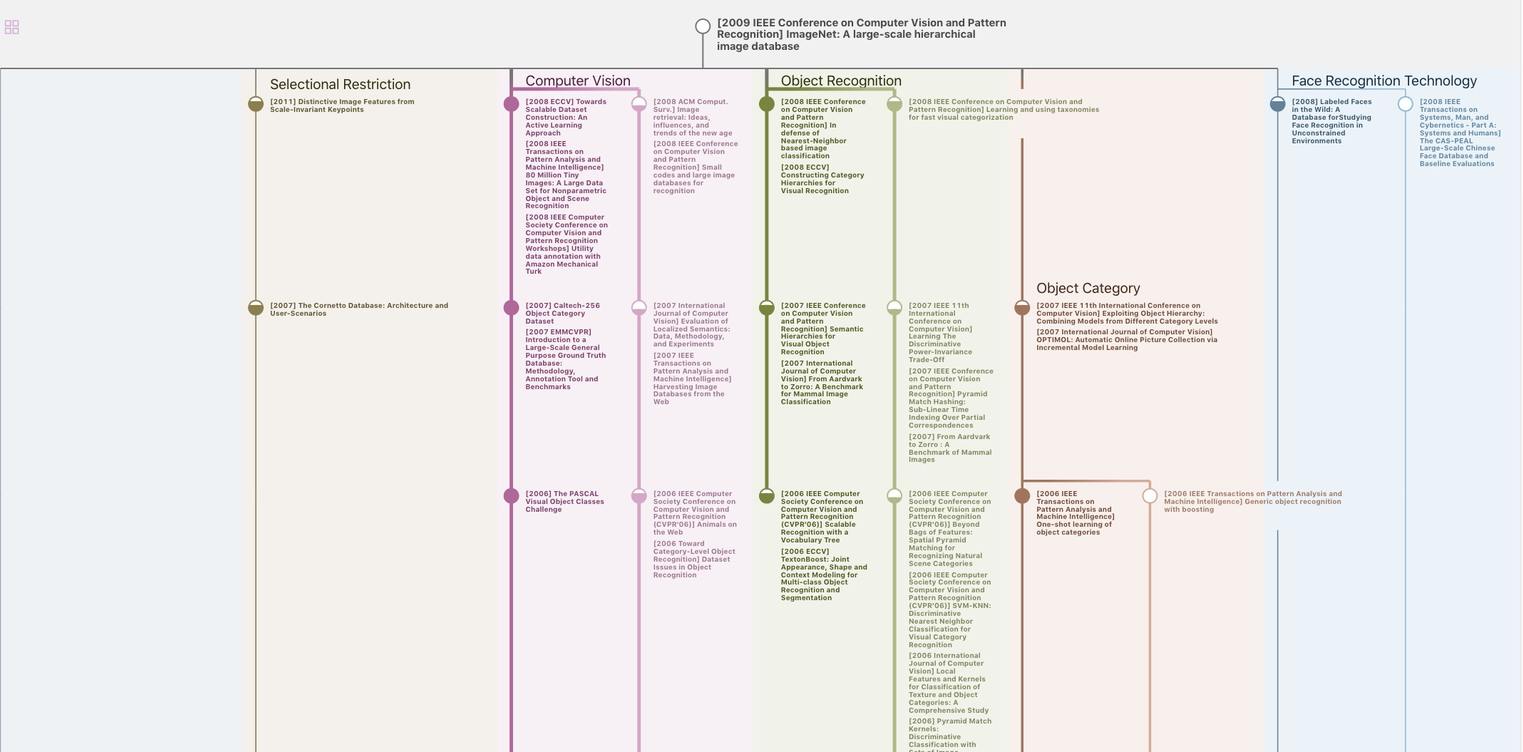
生成溯源树,研究论文发展脉络
Chat Paper
正在生成论文摘要