An Integrated Graph Model For Document Summarization
INFORMATION(2018)
摘要
Extractive summarization aims to produce a concise version of a document by extracting information-rich sentences from the original texts. The graph-based model is an effective and efficient approach to rank sentences since it is simple and easy to use. However, its performance depends heavily on good text representation. In this paper, an integrated graph model (iGraph) for extractive text summarization is proposed. An enhanced embedding model is used to detect the inherent semantic properties at the word level, bigram level and trigram level. Words with part-of-speech (POS) tags, bigrams and trigrams were extracted to train the embedding models. Based on the enhanced embedding vectors, the similarity values between the sentences were calculated in three perspectives. The sentences in the document were treated as vertexes and the similarity between them as edges. As a result, three different types of semantic graphs were obtained for every document, with the same nodes and different edges. These three graphs were integrated into one enriched semantic graph in a naive Bayesian fashion. After that, TextRank, which is a graph-based ranking algorithm, was applied to rank the sentences, before the top scored sentences were selected for the summary according to the compression rate. Evaluated on the DUC 2002 and DUC 2004 datasets, our proposed method shows competitive performance compared to the state-of-the-art methods.
更多查看译文
关键词
document summarization, word embedding, graph integration, TextRank
AI 理解论文
溯源树
样例
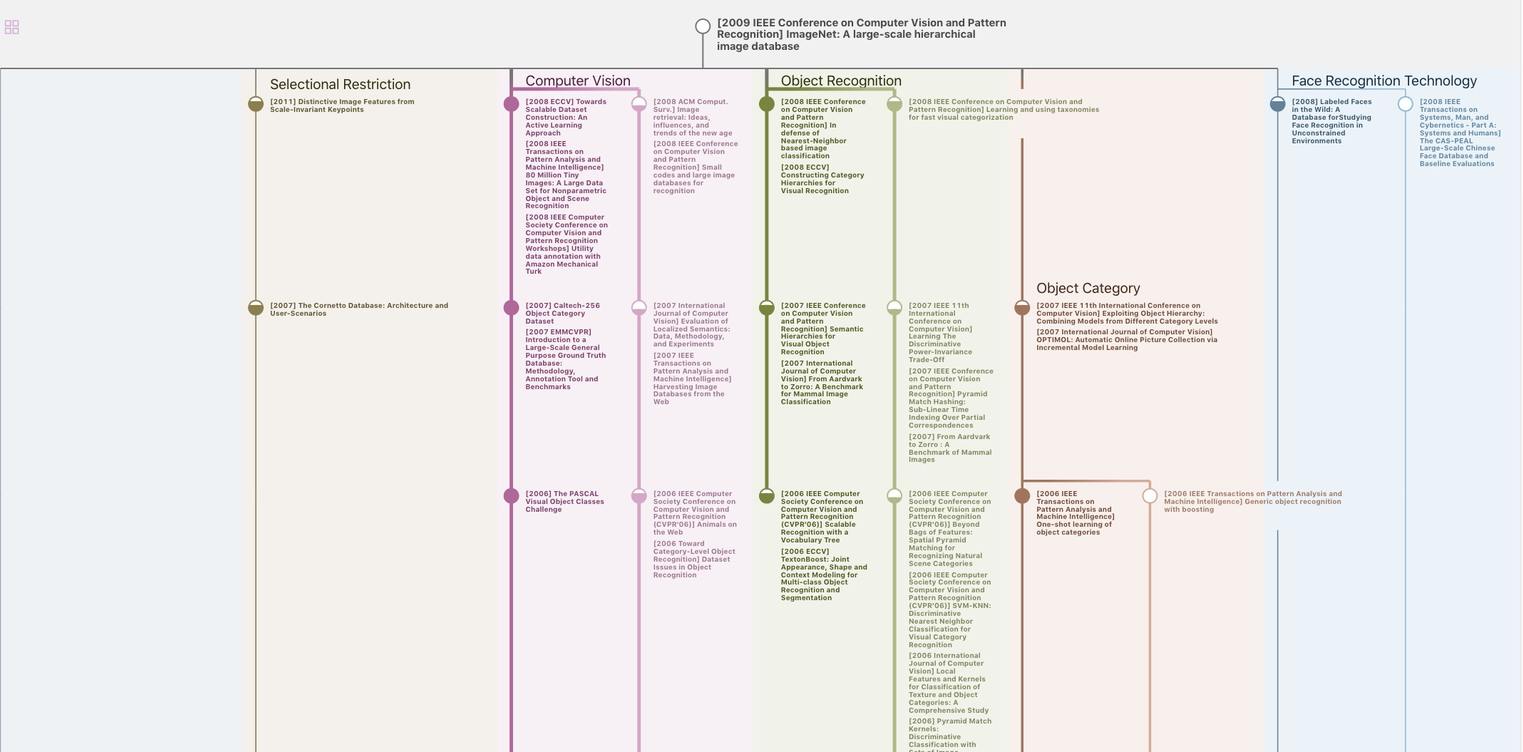
生成溯源树,研究论文发展脉络
Chat Paper
正在生成论文摘要