Co-Metric Learning for Person Re-Identification
Periodicals(2018)
摘要
AbstractPerson re-identification, aiming to identify the same pedestrian images across disjoint camera views, is a key technique of intelligent video surveillance. Although existing methods have developed both theories and experimental results, most of effective ones pertain to fully supervised training styles, which suffer the small sample size (SSS) problem a lot, especially in label-insufficient practical applications. To bridge SSS problem and learning model with small labels, a novel semisupervised co-metric learning framework is proposed to learn a discriminative Mahalanobis-like distance matrix for label-insufficient person re-identification. Different from typical co-training task that contains multiview data originally, single-view person images are firstly decomposed into pseudo two views, and then metric learning models are produced and jointly updated based on both pseudo-labels and references iteratively. Experiments carried out on three representative person re-identification datasets show that the proposed method performs better than state of the art and possesses low label sensitivity.
更多查看译文
关键词
Person Re-identification,Multiple Object Tracking,Visual Tracking,Object Recognition,Object Tracking
AI 理解论文
溯源树
样例
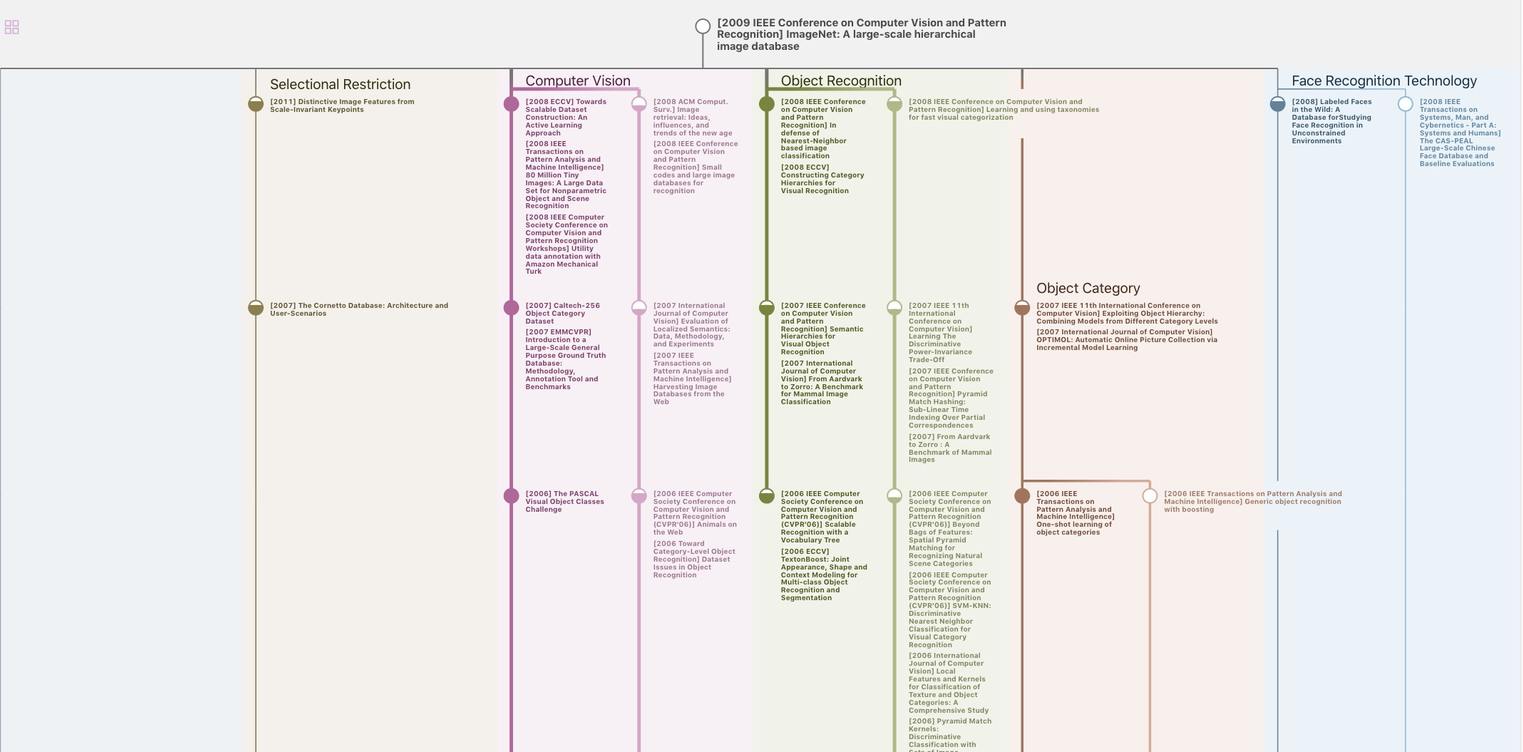
生成溯源树,研究论文发展脉络
Chat Paper
正在生成论文摘要