St-Drn: Deep Residual Networks For Spatio-Temporal Metro Stations Crowd Flows Forecast
2018 INTERNATIONAL JOINT CONFERENCE ON NEURAL NETWORKS (IJCNN)(2018)
摘要
Forecasting the inflow and outflow of crowds at metro station, immediately controlling the number of people entering at some special times and places to avoid the occurrence of malignant events for public safety, is of great significance to subway stations management and very challenging as it is affected by many complex elements, such as inter region station flows, major events or activities, and weather. We propose a approach based on deep residual learning, called ST-DRN, to discern the pattern of spatial and temporal and integrally predict the inflow and outflow of crowds in each subway station of a city. We propose an end-to-end structure of ST-DRN based on distinct attributes of spatio-temporal data. More specifically, we apply the residual neural network framework to model the temporal nearby, day, and week properties of crowd in subway station. For each feature, we design a branch of residual convolutional units, each of which handles the spatial properties of subway crowd. ST-DRN learns to dynamically summation the output of the three residual neural networks, assigning different weights to each branch. The summation is also further combined with external elements, such as weather, holiday and workdays or weekends, to forecast the final traffic flow of crowds in each station. Evaluations on the automatic fare collection (AFC) system subway record data in Suzhou demonstrate that we proposed ST-DRN outperforms than three prominent baseline methods.
更多查看译文
关键词
public safety, deep residual learning, flow forecasting, ST-DRN
AI 理解论文
溯源树
样例
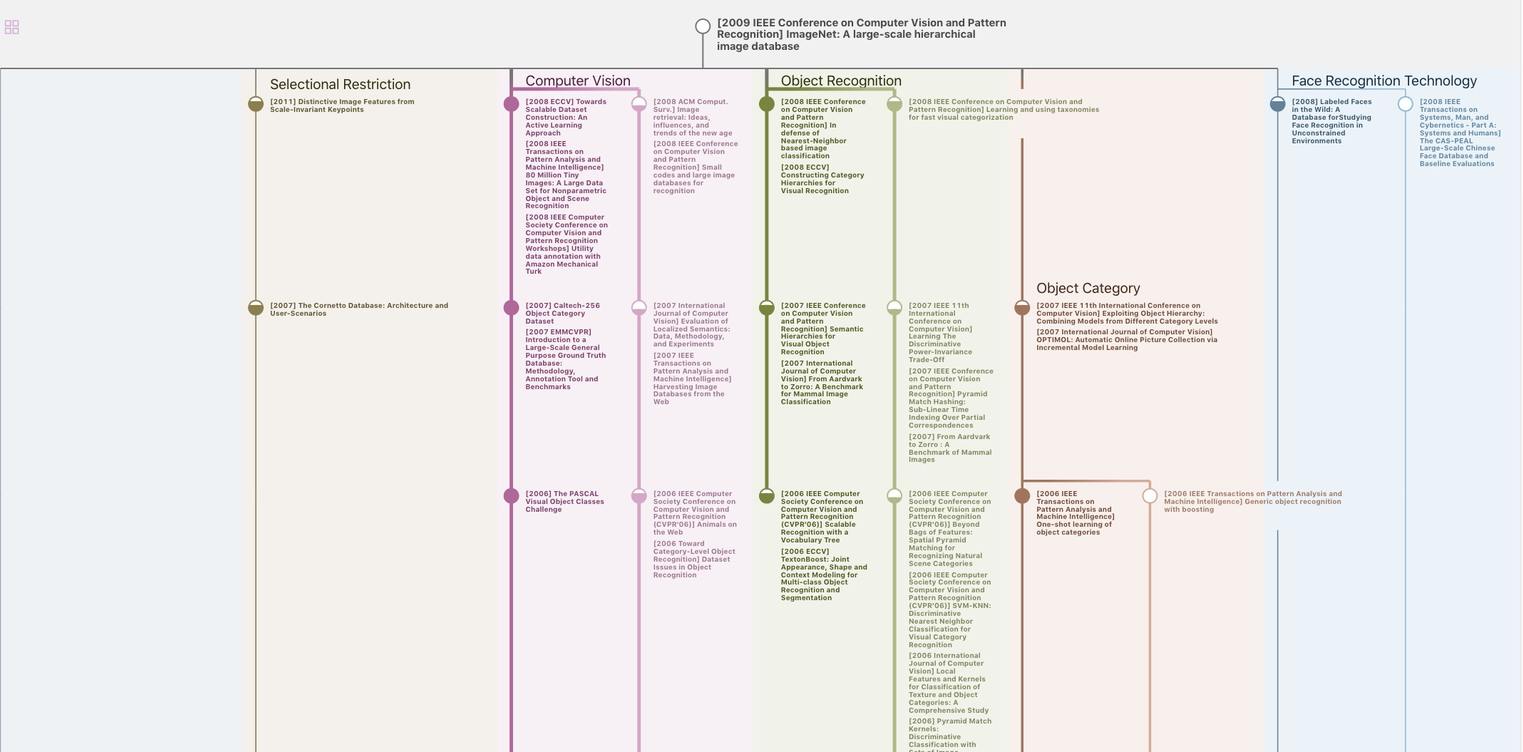
生成溯源树,研究论文发展脉络
Chat Paper
正在生成论文摘要