Towards A One-Stop Solution To Both Aspect Extraction And Sentiment Analysis Tasks With Neural Multi-Task Learning
2018 INTERNATIONAL JOINT CONFERENCE ON NEURAL NETWORKS (IJCNN)(2018)
摘要
Previous studies usually divided aspect-based sentiment analysis into several subtasks in pipeline, i.e., first aspect term and/or opinion term extraction, then aspect-based sentiment prediction, resulting in error propagation and external resources dependency. To overcome the problems mentioned above, in this work we present a novel one-stop solution on aspect-based sentiment analysis. Specifically, we propose a novel multi-task neural learning framework to jointly tackle aspect extraction and sentiment prediction subtasks at the same time, and leverage attention mechanisms to learn the joint representation of aspect-sentiment relationship. We have conducted extensive comparative experiments on two benchmark datasets from SemEval-2014. The experiment results demonstrate the effectiveness of our proposed solution. Especially, our multi-task model outperforms the state-of-the-art systems on aspect extraction subtask.
更多查看译文
关键词
one-stop solution,neural multitask learning,aspect-based sentiment analysis,opinion term extraction,novel multitask neural learning framework,sentiment prediction subtasks,aspect-sentiment relationship,aspect extraction subtask,pipeline,first aspect term
AI 理解论文
溯源树
样例
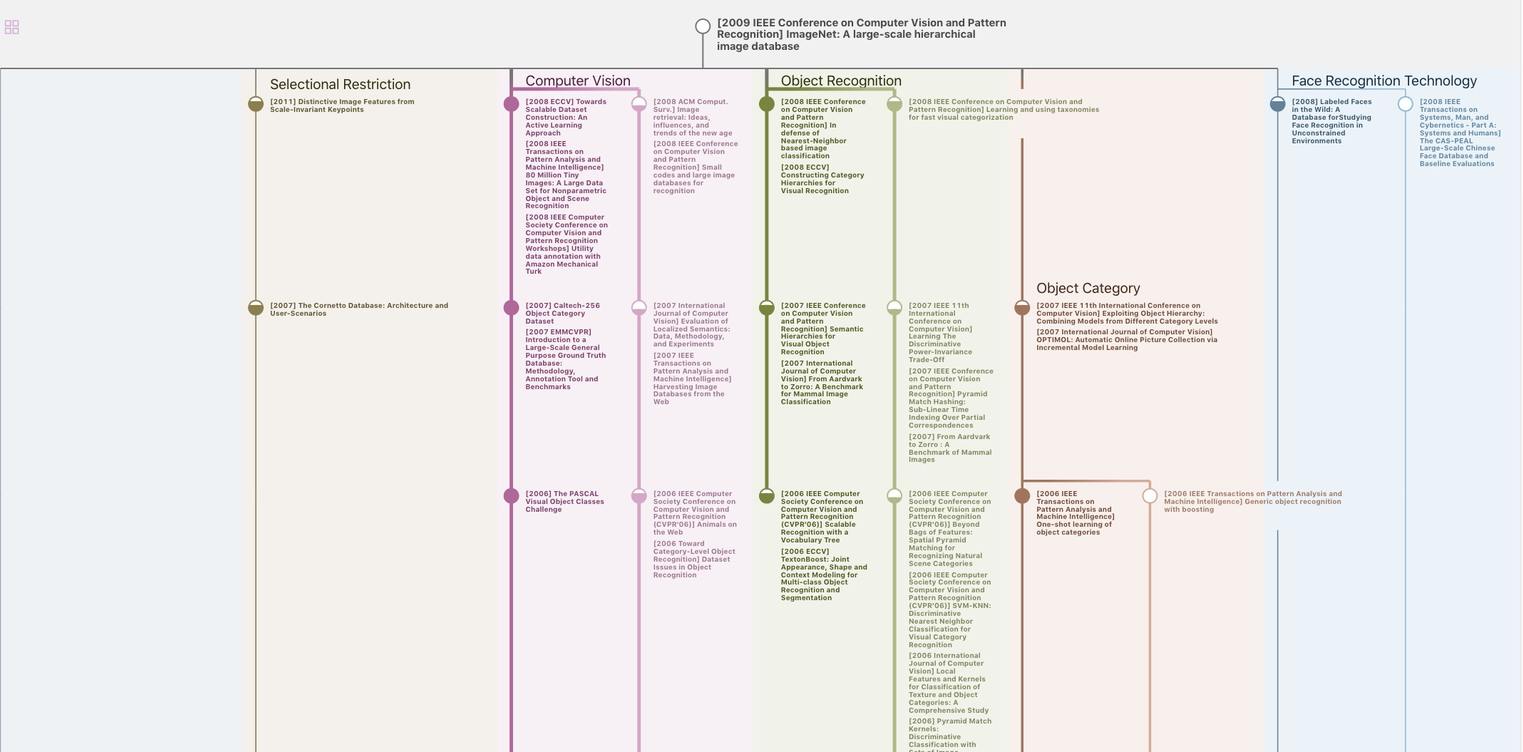
生成溯源树,研究论文发展脉络
Chat Paper
正在生成论文摘要