Improving the accuracy of intelligent forecasting models using the Perturbation Theory
2018 INTERNATIONAL JOINT CONFERENCE ON NEURAL NETWORKS (IJCNN)(2018)
摘要
In time series analysis and forecasting, machine learning (ML) models have been widely used due to their flexibility and accuracy. However, the tuning process of their parameters is a hard task, mainly when complex time series are addressed. So, it is difficult to guarantee the optimal adjustment of the ML model parameters. This paper proposes a recursive approach based on the Perturbation theory to correct the forecasting of ML models. From the initial forecasting given by an ML model, a new ML model is trained using the error series (the difference between the actual series and forecasting) of the first model to decrease the overall error of the system. This process can be recursively repeated until convergence or some stop criterion. The response of the perturbative approach is composed of the sum of the predictions (perturbations) of the ML models trained in each recursion. The proposed approach is investigated with four ML models: Support Vector Regression, Multilayer Perceptron, Long Short-Term Memory, and Radial Basis Function network. The evaluation is performed with an experimental investigation conducted on four time series: Canadian Lynx, Sunspot, Star Brightness, and S&P500 index. The results show that the perturbative approach improves significantly the accuracy of all evaluated ML models.
更多查看译文
关键词
ML model parameters,intelligent forecasting models,time series analysis,perturbation theory,machine learning models,recursive approach,error series,convergence,stop criterion,predictions,support vector regression,multilayer perceptron,long short-term memory,radial basis function network,Canadian Lynx,Sunspot,Star Brightness
AI 理解论文
溯源树
样例
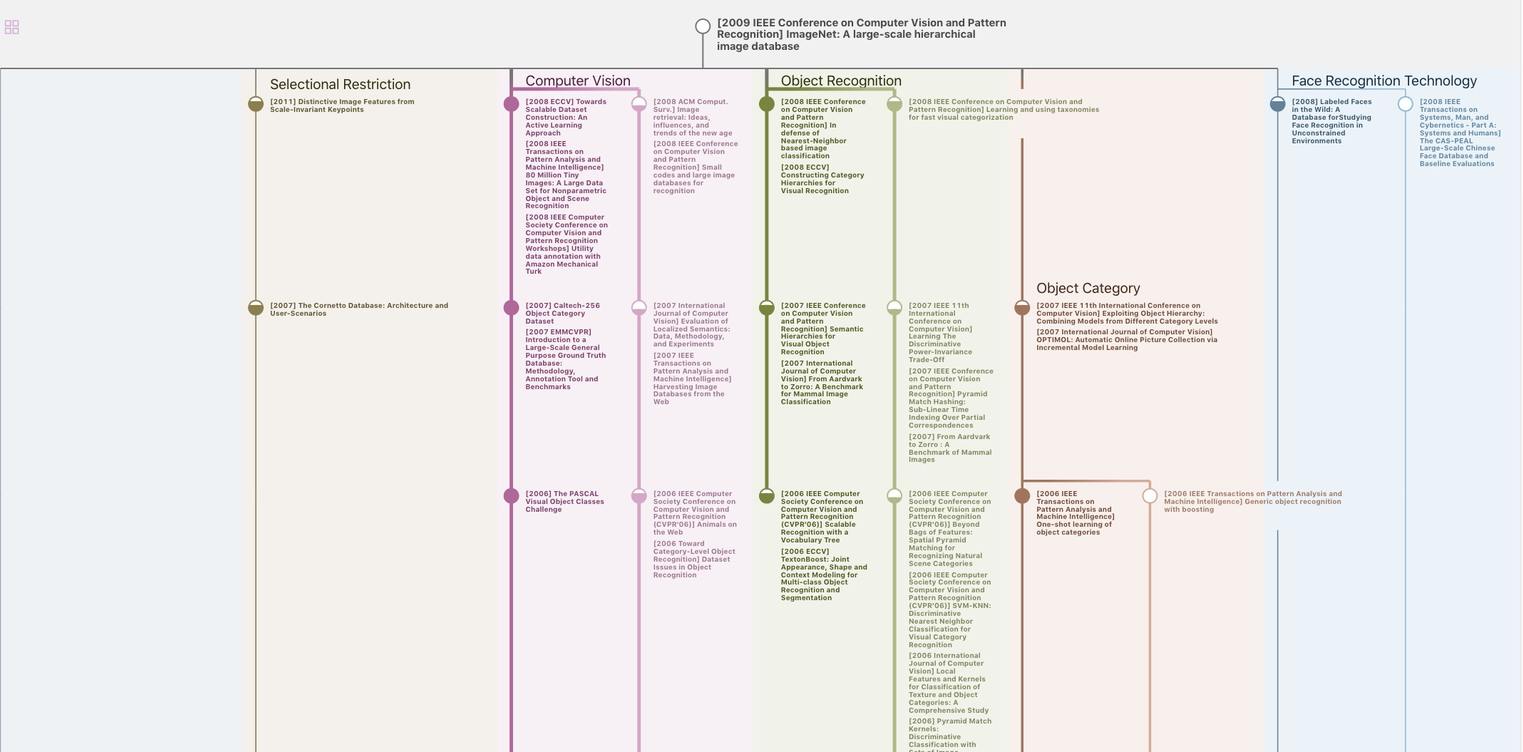
生成溯源树,研究论文发展脉络
Chat Paper
正在生成论文摘要