Enhancement Of Deep Architecture Using Dropout / Dropconnect Techniques Applied For Ahr System
2018 INTERNATIONAL JOINT CONFERENCE ON NEURAL NETWORKS (IJCNN)(2018)
摘要
Remarkable performance on computer vision, and especially on pattern recognition field has been known for a long time to be produced by Deep learning algorithms. It is clear that amongst the successful applications in the pattern recognition domain, Arabic handwriting recognition (AHR) is a must. In this survey, we use two deep networks: Deep Belief Network (DBN) and Convolutional Neural Networks (CNN), for Arabic handwritten script (AHS) recognition. Despite the triumph of DBN and CNN methods, over-fitting is able to take place on these networks thanks to the massive number of parameters. In order to fight over-fitting, we have deeply inquired two regularization techniques called Dropout and DropConnect. While training with the two regularization methods, a randomly chosen subsets of activations/weights are dropped. Consequently, the assessment on the HACDB database to treat character level proves shows an improvement of classification error rate once adding Dropout and DropConnect techniques.
更多查看译文
关键词
Arabic handwritten script, CNN, Deep learning, DBN, Dropout, DropConnect, over-fitting
AI 理解论文
溯源树
样例
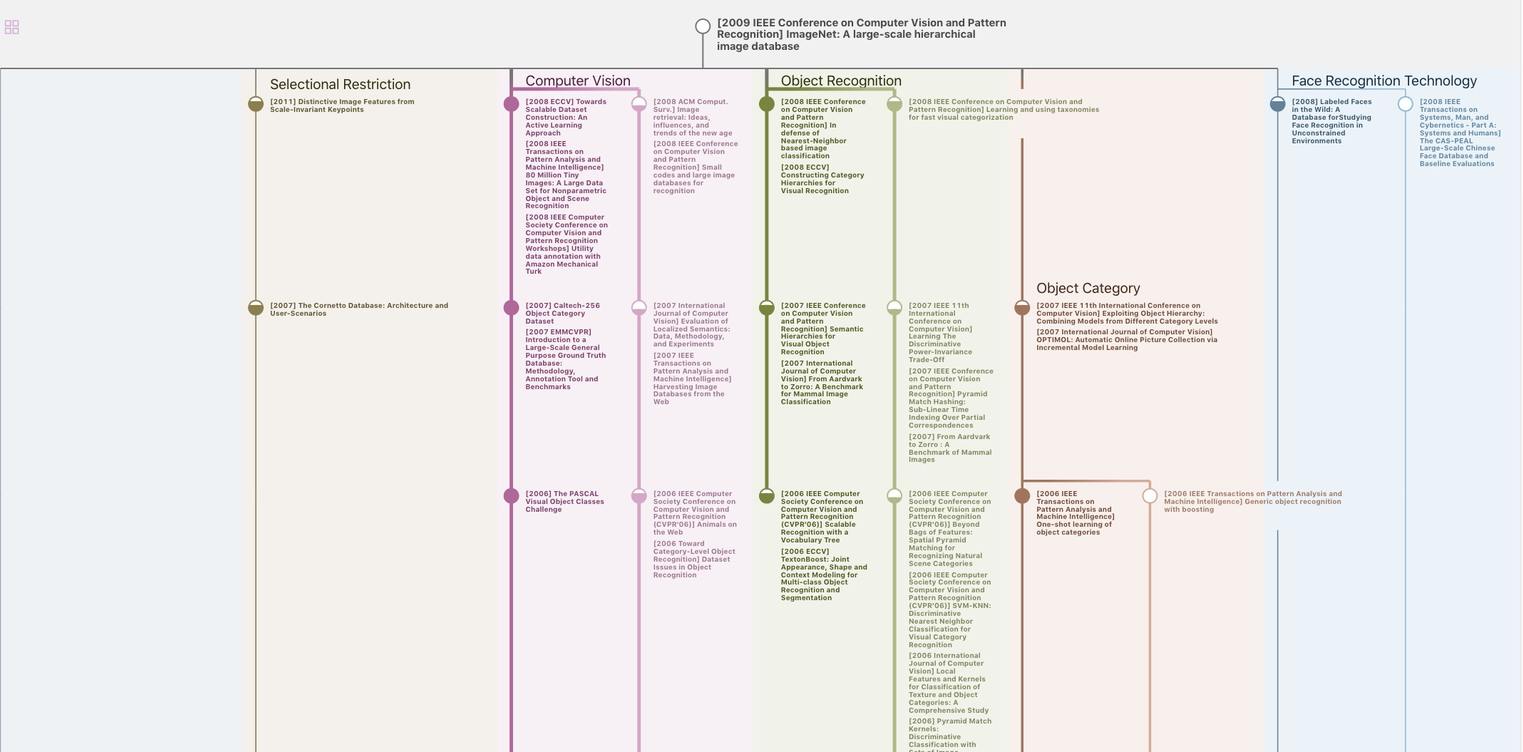
生成溯源树,研究论文发展脉络
Chat Paper
正在生成论文摘要