Matrix Factorization Based Collaborative Filtering With Resilient Stochastic Gradient Descent
2018 INTERNATIONAL JOINT CONFERENCE ON NEURAL NETWORKS (IJCNN)(2018)
Abstract
One of the leading approaches to collaborative filtering is to use matrix factorization to discover a set of latent factors that explain the pattern of preferences. In this paper, we apply a resilient stochastic gradient descent approach that uses only the sign of the gradient, similar to the R-Prop algorithm in neural network training, to matrix factorization for collaborative filtering. We evaluate the performance of our approach on the MovieLens 1M dataset, and find that test set accuracy markedly improves compared to standard gradient descent. As a follow-up experiment, we apply clustering to the learned item-factor matrix in factor space, and attempt to manually characterize each cluster of movies.
MoreTranslated text
Key words
matrix factorization,collaborative filtering,standard gradient descent,item-factor matrix,resilient stochastic gradient descent,R-Prop algorithm,neural network training,MovieLens 1M dataset,recommender system
AI Read Science
Must-Reading Tree
Example
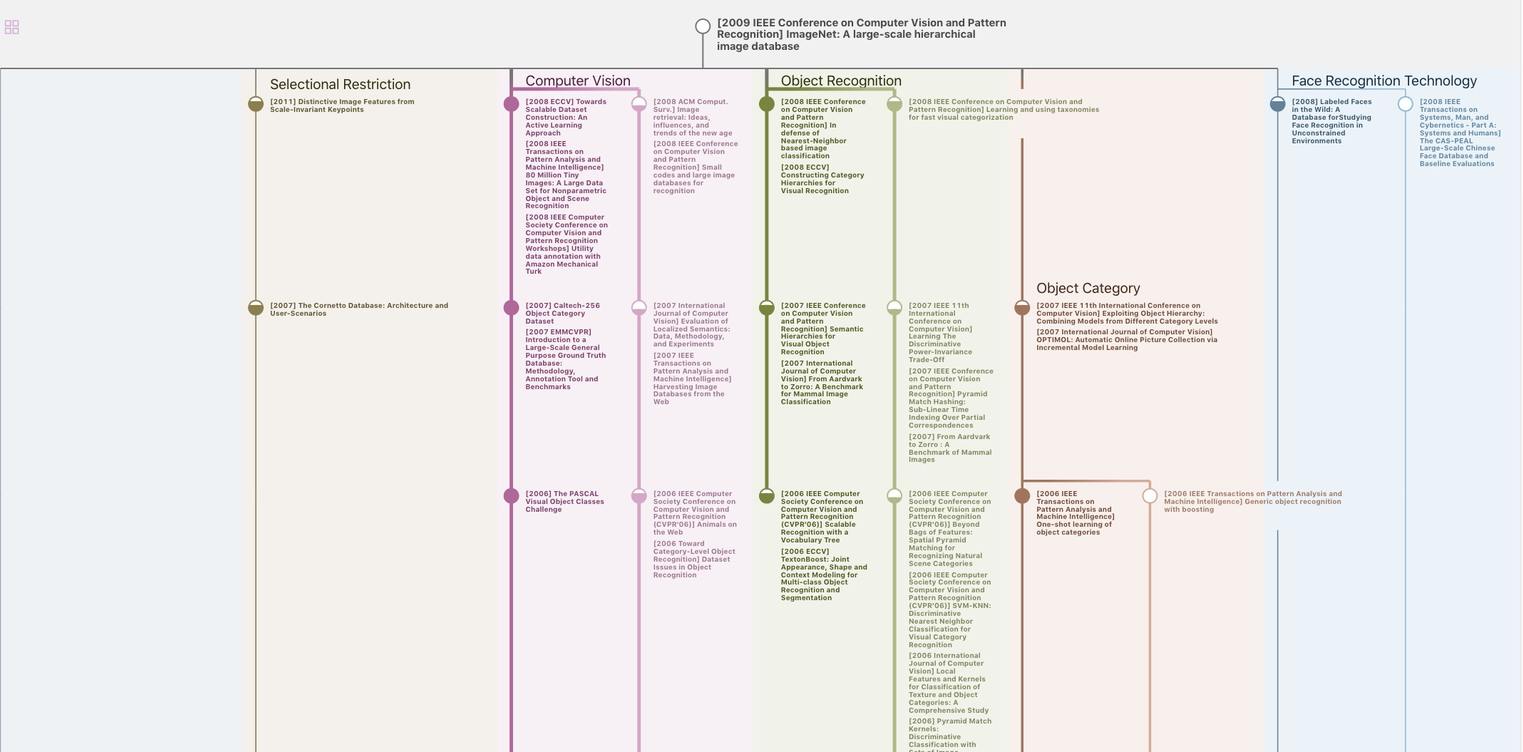
Generate MRT to find the research sequence of this paper
Chat Paper
Summary is being generated by the instructions you defined