PRIMA: An End-to-End Framework for Privacy at Scale
2018 IEEE 34TH INTERNATIONAL CONFERENCE ON DATA ENGINEERING (ICDE)(2018)
摘要
Person-specific data offer enormous opportunities for deriving insights that can radically improve different facets of our everyday lives, ranging from the provisioning of personalized medicine and healthcare, to the offering of smart transportation and smart energy. At the same time, the use of person-specific data to support these applications can come at a high cost to individuals' privacy, unless proper de-identification technology is in place to provide rigorous privacy guarantees. In this paper we introduce PRIMA, an end-to-end solution allowing decision makers to map out and execute their data privacy strategy through a comprehensive workflow. Our toolkit offers an intuitive risk-utility exploration framework for end users to navigate through the enormous number of possible combinations of anonymization settings and provide meaningful reports that help them understand the impact of each strategy in terms of utility and risk. Unlike traditional approaches, that rely on limited scale tools and manual analyses, our toolkit is the first scalable, production-grade system that can execute all of its components (such as vulnerability analysis, anonymization, risk and information loss measurements) on arbitrarily large datasets. Furthermore, it offers a flexible library for developers to integrate and extend its functionality to embed de-identification components into their applications.
更多查看译文
关键词
privacy,scalability,k-anonymity,privacy risk
AI 理解论文
溯源树
样例
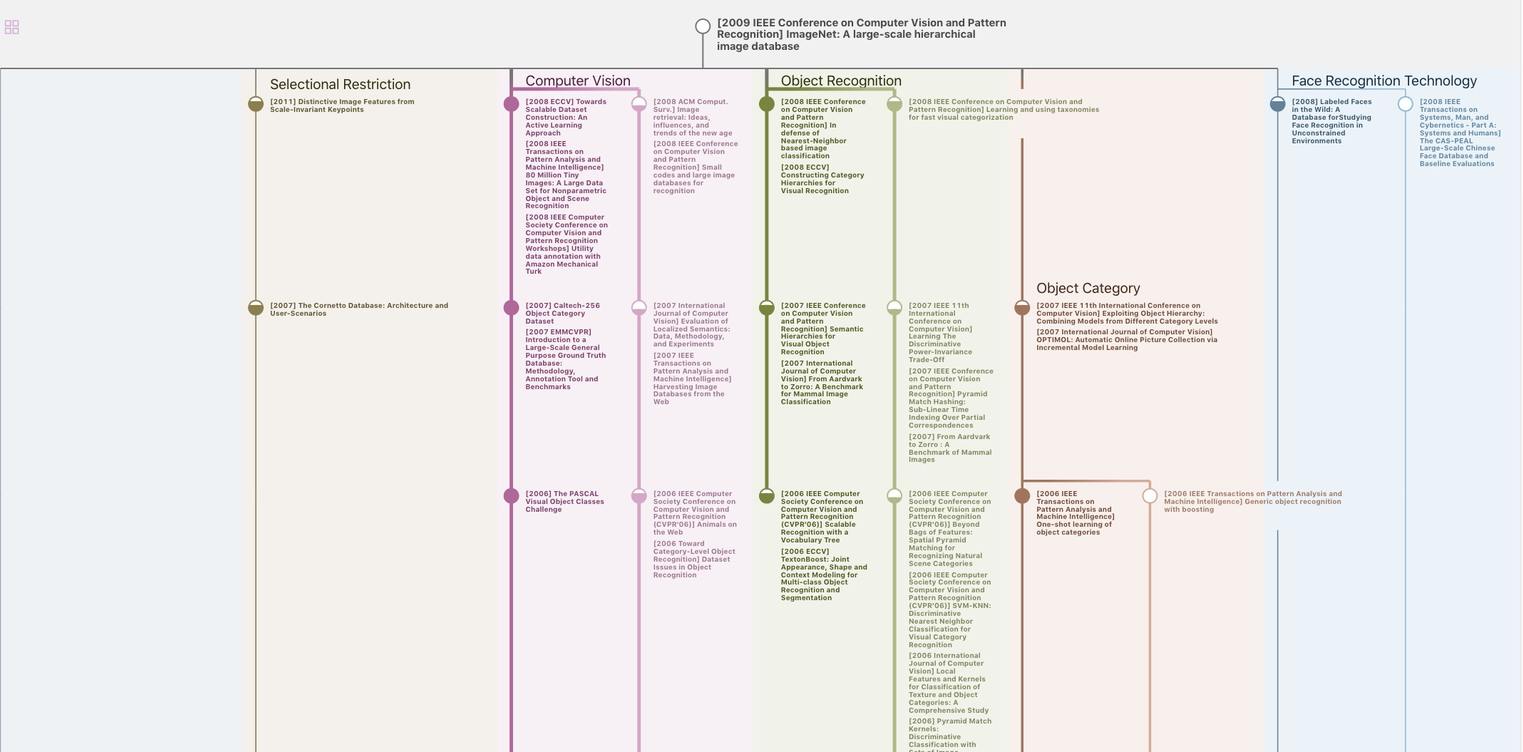
生成溯源树,研究论文发展脉络
Chat Paper
正在生成论文摘要