Deep Graph Embedding for Ranking Optimization in E-commerce.
CIKM(2018)
摘要
Matching buyers with most suitable sellers providing relevant items (e.g., products) is essential for e-commerce platforms to guarantee customer experience. This matching process is usually achieved through modeling inter-group (buyer-seller) proximity by e-commerce ranking systems. However, current ranking systems often match buyers with sellers of various qualities, and the mismatch is detrimental to not only buyers' level of satisfaction but also the platforms' return on investment (ROI). In this paper, we address this problem by incorporating intra-group structural information (e.g., buyer-buyer proximity implied by buyer attributes) into the ranking systems. Specifically, we propose De ep Gr aph E mbe dding (DEGREE), a deep learning based method, to exploit both inter-group and intra-group proximities jointly for structural learning. With a sparse filtering technique, DEGREE can significantly improve the matching performance with computation resources less than that of alternative deep learning based methods. Experimental results demonstrate that DEGREE outperforms state-of-the-art graph embedding methods on real-world e-commence datasets. In particular, our solution boosts the average unit price in purchases during an online A/B test by up to 11.93%, leading to better operational efficiency and shopping experience.
更多查看译文
关键词
A/B Test,Customer Matching,Deep Learning,E-commerce Ranking,Graph Embedding,Structure Learning
AI 理解论文
溯源树
样例
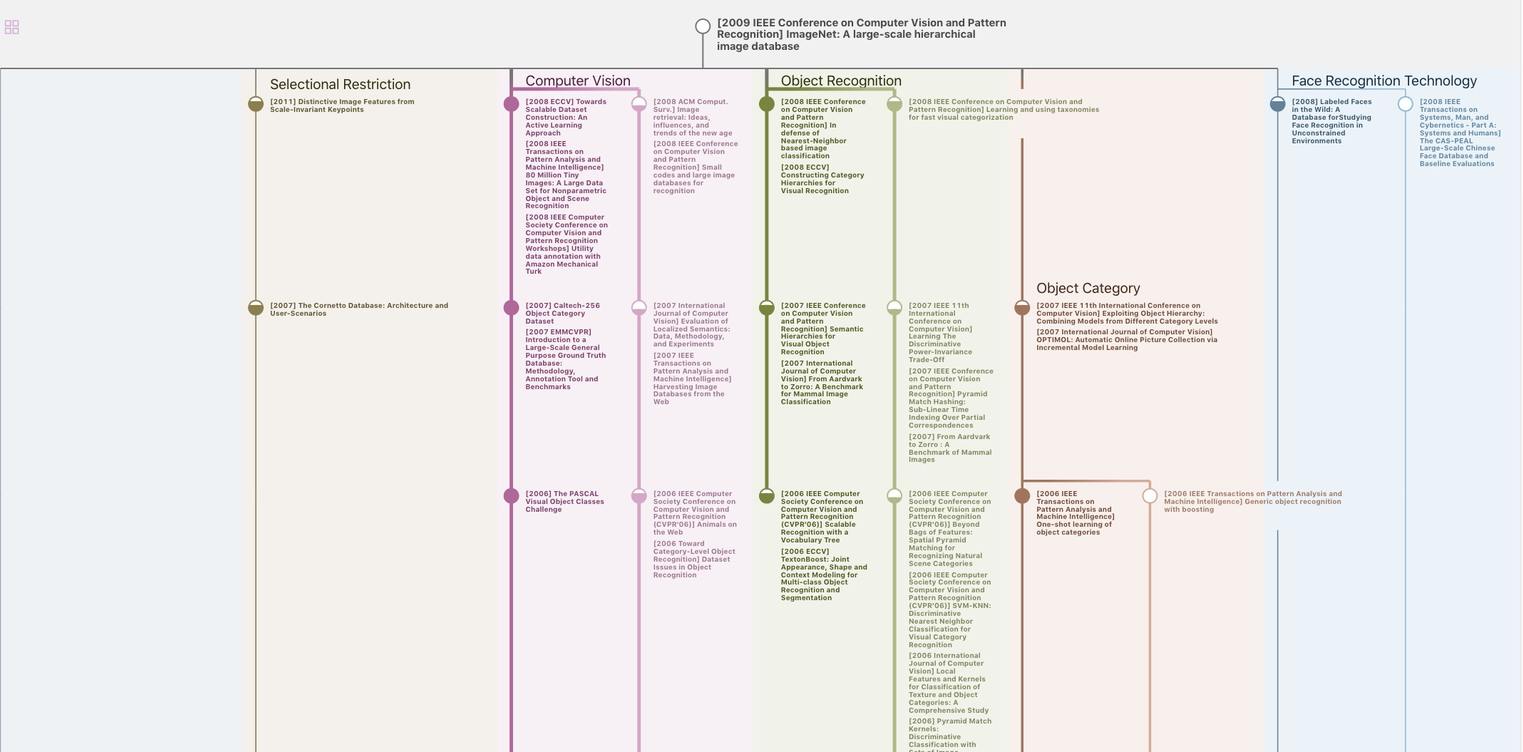
生成溯源树,研究论文发展脉络
Chat Paper
正在生成论文摘要