"Bridge": Enhanced Signed Directed Network Embedding.
CIKM(2018)
摘要
Signed directed networks with positive or negative links convey rich information such as like or dislike, trust or distrust. Existing work of sign prediction mainly focuses on triangles (triadic nodes) motivated by balance theory to predict positive and negative links. However, real-world signed directed networks can contain a good number of "bridge'' edges which, by definition, are not included in any triangles. Such edges are ignored in previous work, but may play an important role in signed directed network analysis.%Such edges serve as fundamental building blocks and may play an important role in signed network analysis.
In this paper, we investigate the problem of learning representations for signed directed networks. We present a novel deep learning approach to incorporating two social-psychologic theories, balance and status theories, to model both triangles and "bridge'' edges in a complementary manner. The proposed framework learns effective embeddings for nodes and edges which can be applied to diverse tasks such as sign prediction and node ranking. Experimental results on three real-world datasets of signed directed social networks verify the essential role of "bridge" edges in signed directed network analysis by achieving the state-of-the-art performance.
更多查看译文
关键词
signed directed network embedding, balance theory, status theory
AI 理解论文
溯源树
样例
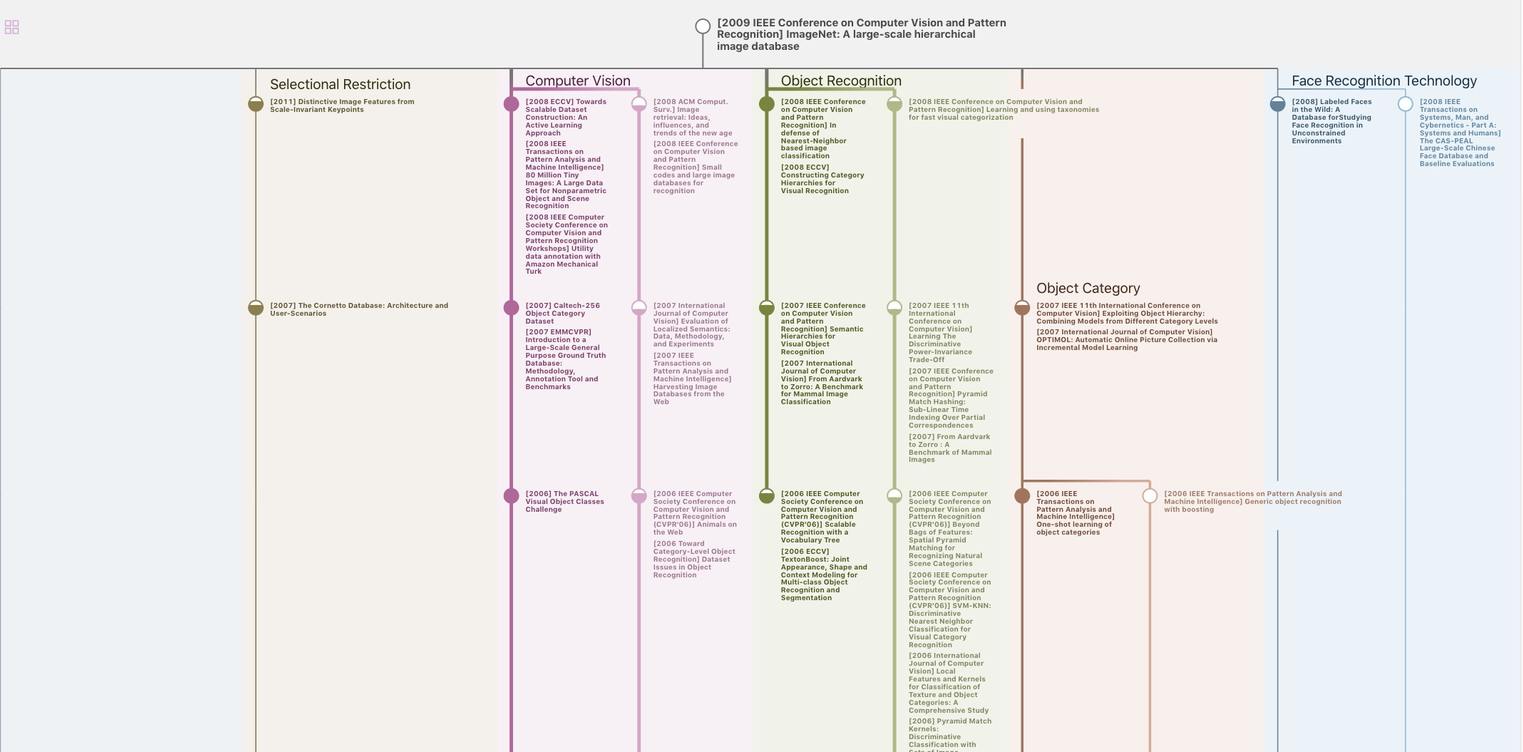
生成溯源树,研究论文发展脉络
Chat Paper
正在生成论文摘要