Weakly-Supervised Neural Text Classification.
CIKM(2018)
摘要
Deep neural networks are gaining increasing popularity for the classic text classification task, due to their strong expressive power and less requirement for feature engineering. Despite such attractiveness, neural text classification models suffer from the lack of training data in many real-world applications. Although many semi-supervised and weakly-supervised text classification models exist, they cannot be easily applied to deep neural models and meanwhile support limited supervision types. In this paper, we propose a weakly-supervised method that addresses the lack of training data in neural text classification. Our method consists of two modules: (1) a pseudo-document generator that leverages seed information to generate pseudo-labeled documents for model pre-training, and (2) a self-training module that bootstraps on real unlabeled data for model refinement. Our method has the flexibility to handle different types of weak supervision and can be easily integrated into existing deep neural models for text classification. We have performed extensive experiments on three real-world datasets from different domains. The results demonstrate that our proposed method achieves inspiring performance without requiring excessive training data and outperforms baseline methods significantly.
更多查看译文
关键词
Text Classification, Weakly-supervised Learning, Pseudo Document Generation, Neural Classification Model
AI 理解论文
溯源树
样例
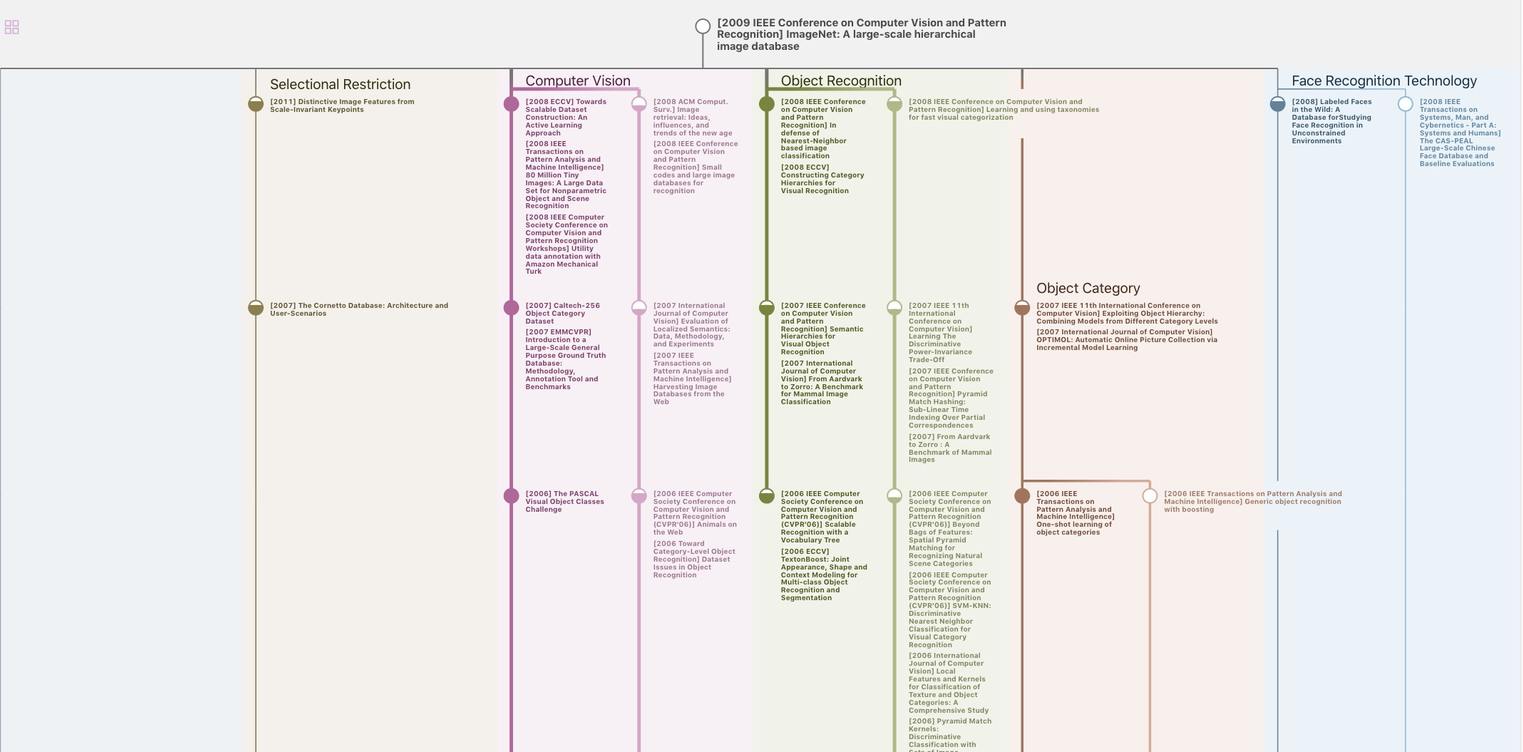
生成溯源树,研究论文发展脉络
Chat Paper
正在生成论文摘要