Improving Low-Rank Matrix Completion with Self-Expressiveness.
CIKM(2018)
摘要
In this paper, we improve the low-rank matrix completion algorithm by assuming that the data points lie in a union of low dimensional subspaces. We applied the self-expressiveness, which is a property of a dataset when the data points lie in a union of low dimensional subspaces, to the low-rank matrix completion. By considering self-expressiveness of low dimensional subspaces, the proposed low-rank matrix completion may perform well even with little information, leading to the robust completion on a dataset with high missing rate. In our experiments on movie rating datasets, the proposed model outperforms state-of-the-art matrix completion models. In clustering experiments conducted on MNIST dataset, the result indicates that our method closely recovers the subspaces of original dataset even with the high missing rate.
更多查看译文
关键词
matrix completion, subspace clustering, collaborative filtering
AI 理解论文
溯源树
样例
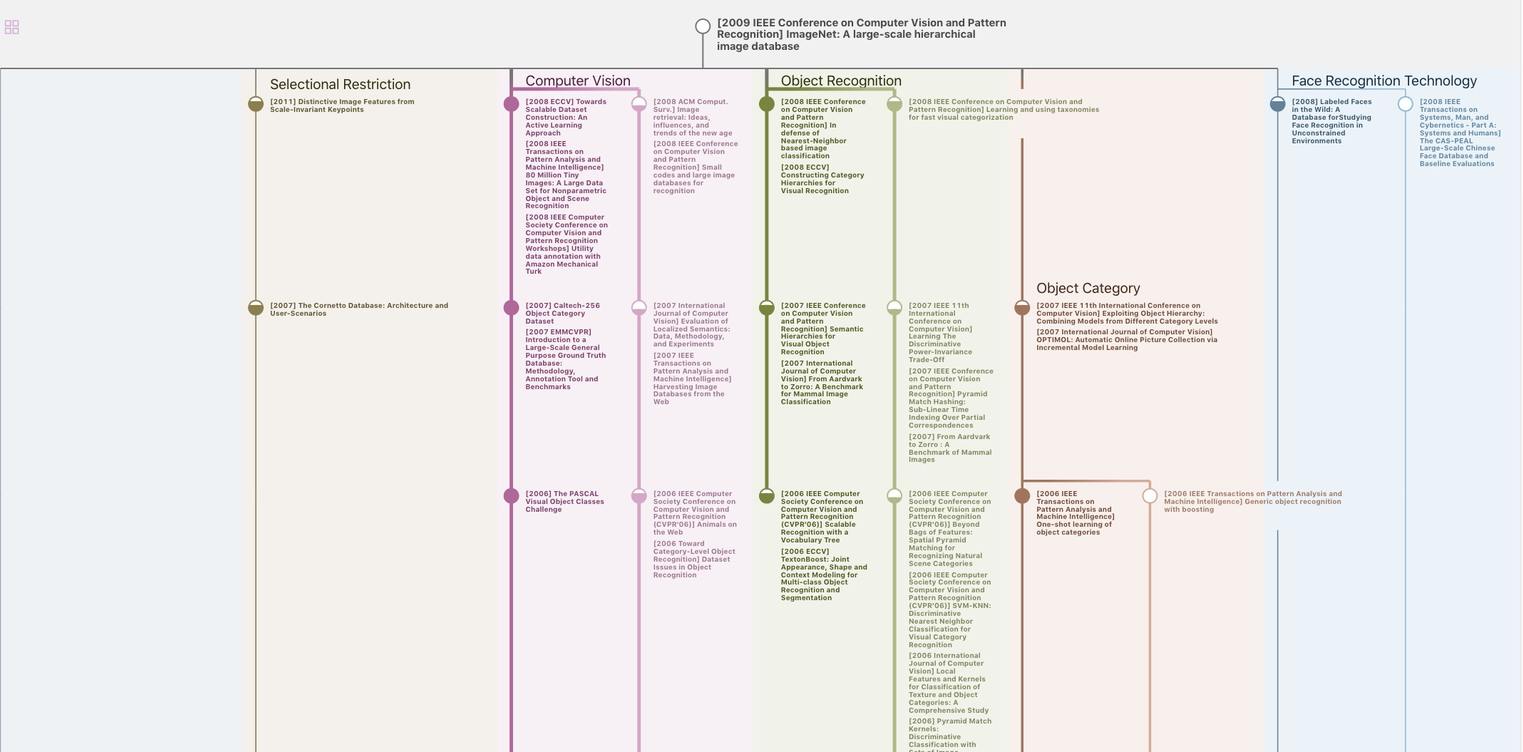
生成溯源树,研究论文发展脉络
Chat Paper
正在生成论文摘要