ConvNets and ImageNet Beyond Accuracy: Understanding Mistakes and Uncovering Biases
COMPUTER VISION - ECCV 2018, PT VI(2018)
摘要
ConvNets and Imagenet have driven the recent success of deep learning for image classification. However, the marked slowdown in performance improvement combined with the lack of robustness of neural networks to adversarial examples and their tendency to exhibit undesirable biases question the reliability of these methods. This work investigates these questions from the perspective of the end-user by using human subject studies and explanations. The contribution of this study is threefold. We first experimentally demonstrate that the accuracy and robustness of ConvNets measured on Imagenet are vastly underestimated. Next, we show that explanations can mitigate the impact of misclassified adversarial examples from the perspective of the end-user. We finally introduce a novel tool for uncovering the undesirable biases learned by a model. These contributions also show that explanations are a valuable tool both for improving our understanding of ConvNets' predictions and for designing more reliable models.
更多查看译文
关键词
Bias detection, Interpretability, Adversarial examples
AI 理解论文
溯源树
样例
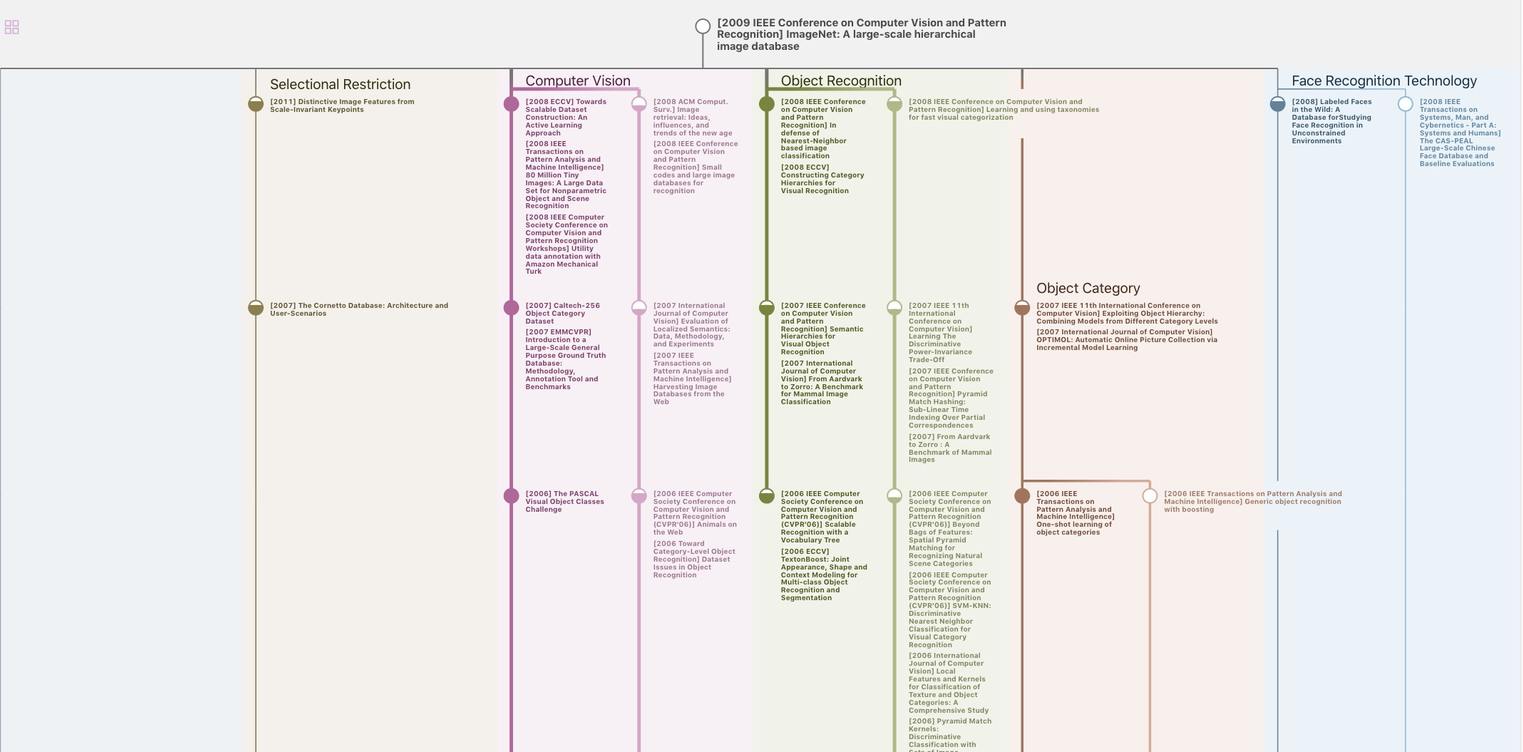
生成溯源树,研究论文发展脉络
Chat Paper
正在生成论文摘要