Attributed Network Representation Learning Approaches for Link Prediction.
ASONAM '18: International Conference on Advances in Social Networks Analysis and Mining Barcelona Spain August, 2018(2018)
摘要
Network representation learning algorithms seek to embed the nodes of a network into a lower-dimensional feature space such that nodes that are in close proximity to each other share a similar representation. In this paper, we investigate the effectiveness of using network representation learning algorithms for link prediction problems. Specifically, we demonstrate the limitations of existing algorithms in terms of their ability to accurately predict links between nodes that are in the same or different communities and nodes that have low degrees. We also show that incorporating node attribute information can help alleviate this problem and compare three different approaches to integrate this information with network representation learning for link prediction problems. Using five real-world network datasets, we demonstrate the efficacy of one such approach, called SPIN, that can effectively combine the link structure with node attribute information and predict links between nodes in the same and different communities without favoring high degree nodes.
更多查看译文
关键词
attributed network representation learning,network representation learning algorithms,lower-dimensional feature space,link prediction problems,real-world network datasets,link structure,node attribute information,SPIN approach
AI 理解论文
溯源树
样例
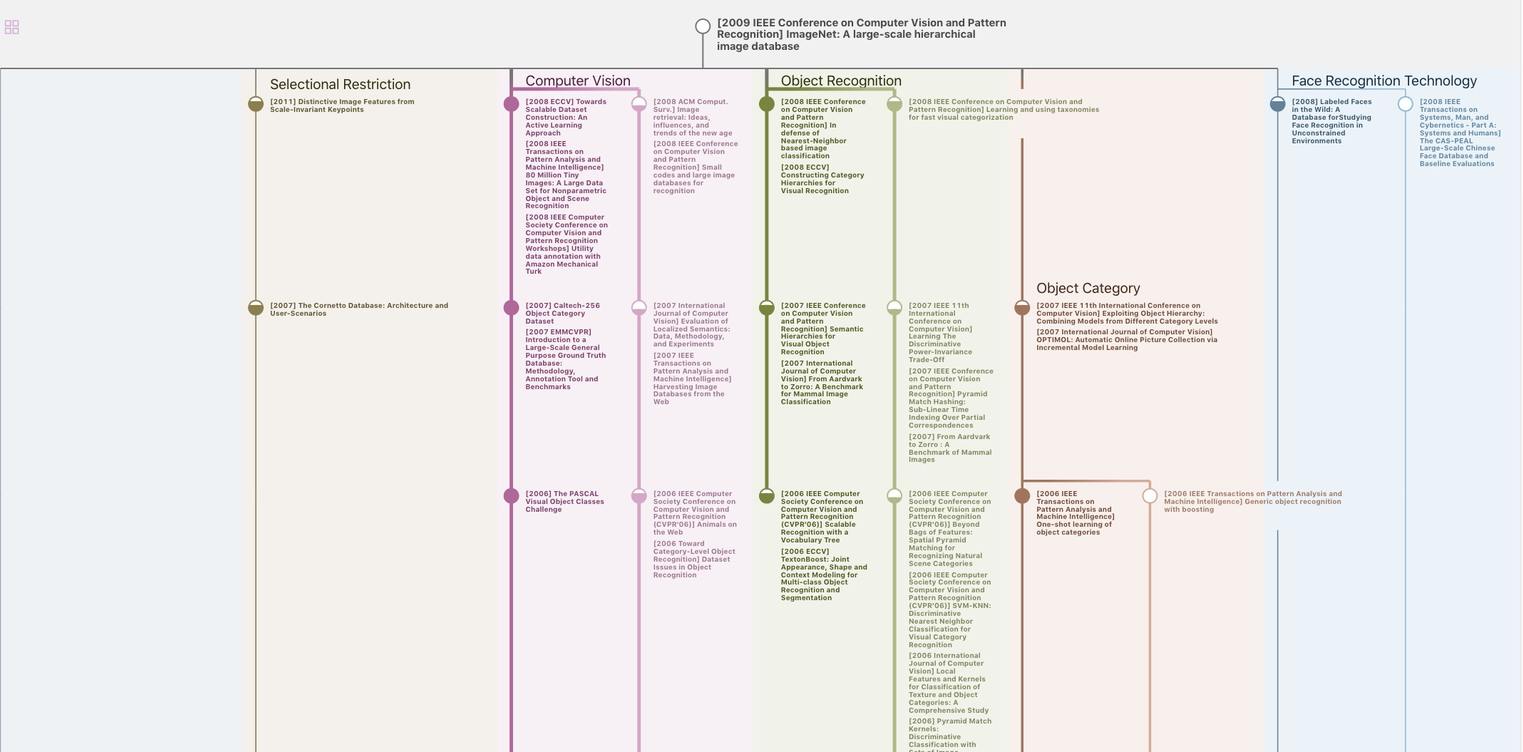
生成溯源树,研究论文发展脉络
Chat Paper
正在生成论文摘要