Target-Oriented Granular Inference With Evolutionary Updating For Converter Gas Scheduling
2018 IEEE CONGRESS ON EVOLUTIONARY COMPUTATION (CEC)(2018)
摘要
An accurate and effective scheduling of byproduct gas in steel industry is significant for cost reduction and environment protection. In this study, a target-oriented granular inference method is proposed for realizing real-time scheduling of converter gas system, in which the fluctuation characteristics of the flow of the gas users are considered for data granulation, and then granular inference is performed to construct the scheduling rules between the gas users and the system scheduling amount. To fully reflect the impact of scheduling objectives on the modeling process, a target value is proposed to be included in each rule and being further updated by employing residual gradient Q-learning method, which is used to select appropriate scheduling policies from inconsistent fuzzy rules. On their basis, the scheduling rule base is updated online by utilizing evolutionary computation method. To demonstrate the effectiveness of the proposed method, the practical data coming from the energy center of a large-sized steel industry are employed, the results indicate that the proposed model could guarantee a higher accuracy and make the operation of converter gas system safe and stable.
更多查看译文
关键词
steel industry, byproduct gas scheduling, granular inference, reinforcement learning
AI 理解论文
溯源树
样例
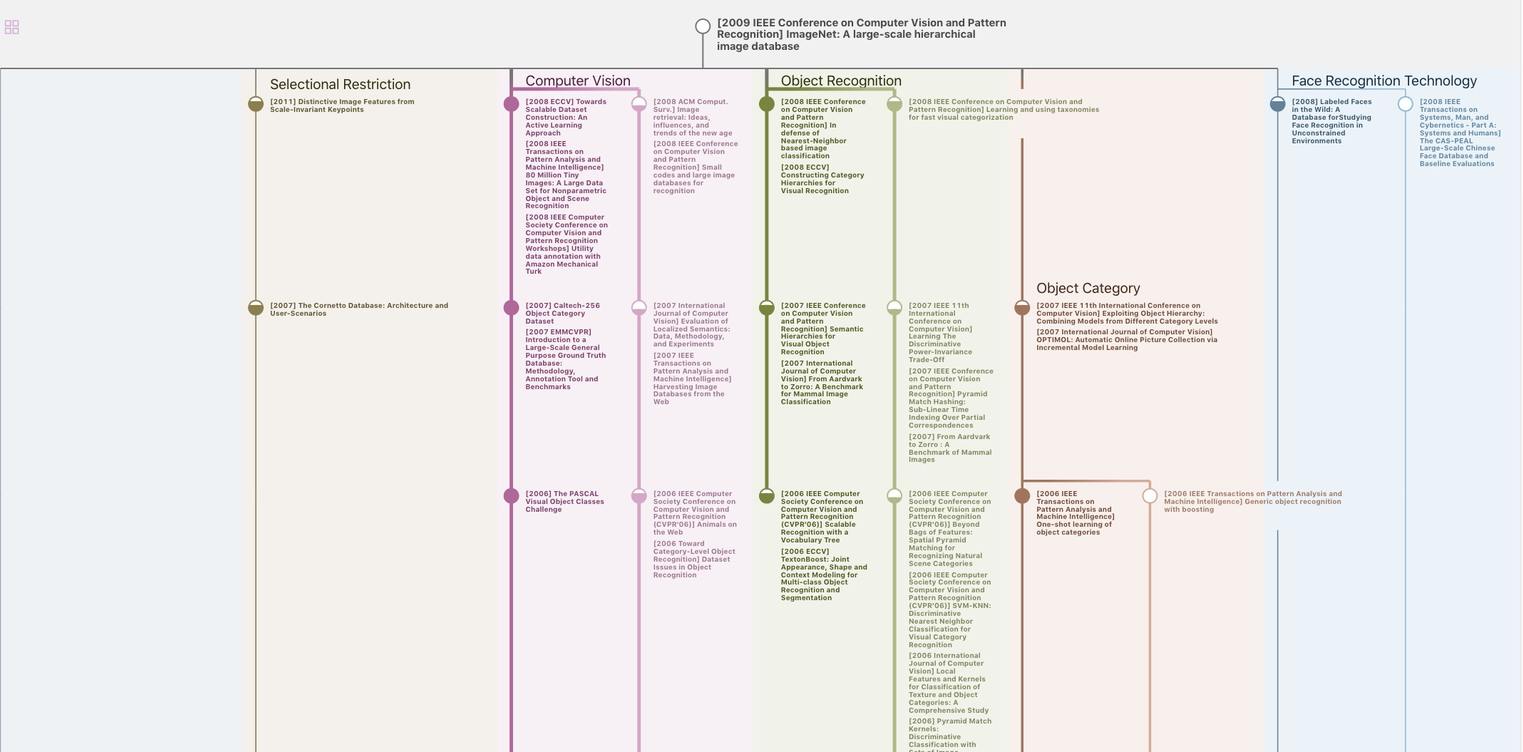
生成溯源树,研究论文发展脉络
Chat Paper
正在生成论文摘要