Modeling Machine Health Using Gated Recurrent Units with Entity Embeddings and K-Means Clustering
2018 IEEE 16th International Conference on Industrial Informatics (INDIN)(2018)
摘要
We describe a machine learning approach for predicting machine health indicators two weeks into the future. The model developed uses a neural network architecture that incorporates sensor data inputs using gated recurrent units with metadata inputs using entity embeddings. Both inputs are then concatenated and fed to a fully connected neural network classifier. Furthermore, our classes are generated by clustering the continuous sensor values of the training data using K-Means. To validate the model we performed an ablation study in order to verify the effectiveness of each of the model’s components, and also compared our approach to the typical method of predicting continuous scalar values.
更多查看译文
关键词
Deep Learning,Neural Networks,Recurrent Neural Networks,Embeddings,Clustering,K- Means,Time Series,Machine Health Monitoring,Predictive Maintenance
AI 理解论文
溯源树
样例
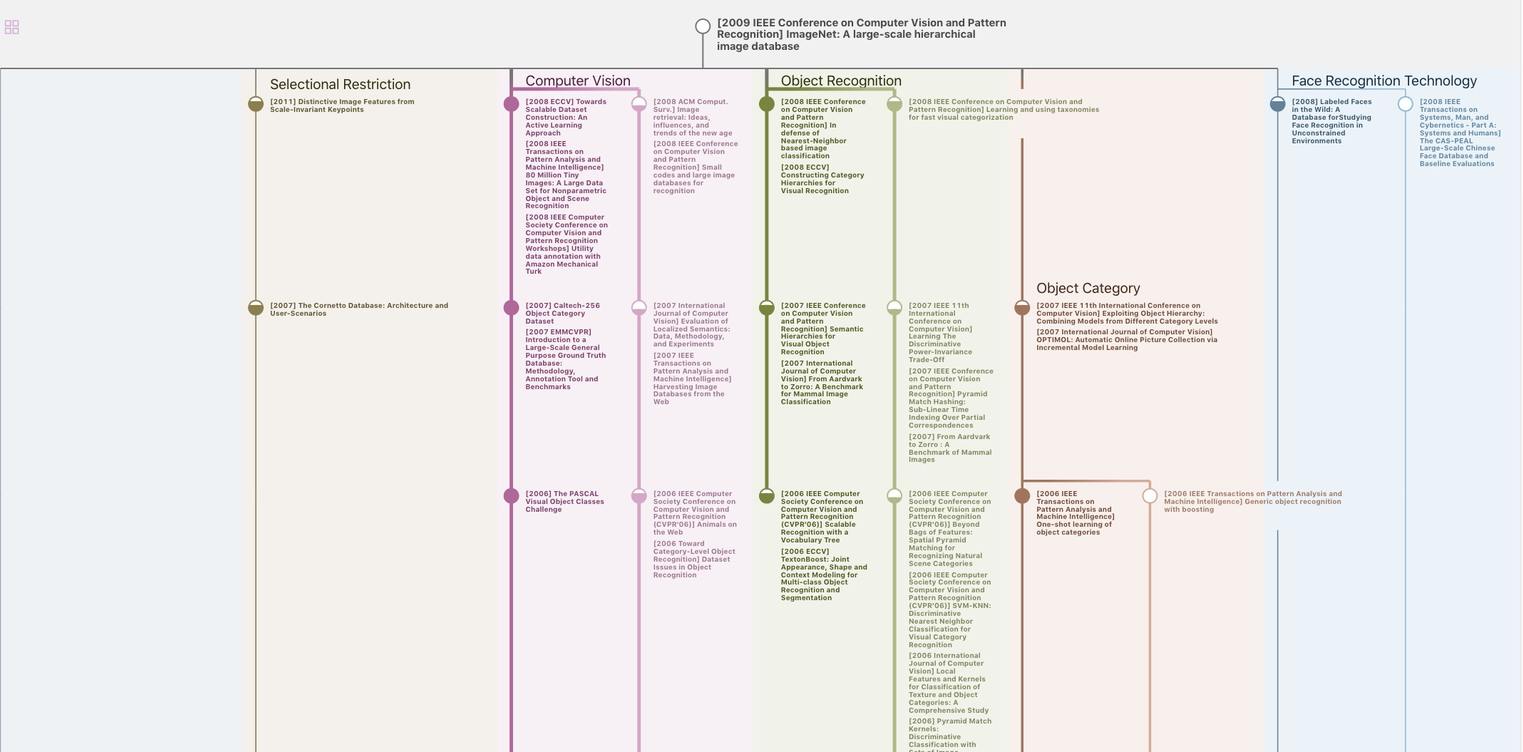
生成溯源树,研究论文发展脉络
Chat Paper
正在生成论文摘要