DeepHPS: End-to-end Estimation of 3D Hand Pose and Shape by Learning from Synthetic Depth
2018 International Conference on 3D Vision (3DV)(2018)
摘要
Articulated hand pose and shape estimation is an important problem for vision-based applications such as augmented reality and animation.In contrast to the existing methods which optimize only for joint positions, we propose a fully supervised deep network which learns to jointly estimate a full 3D hand mesh representation and pose from a single depth image.To this end, a CNN architecture is employed to estimate parametric representations i.e. hand pose, bone scales and complex shape parameters. Then, a novel hand pose and shape layer, embedded inside our deep framework, produces 3D joint positions and hand mesh. Lack of sufficient training data with varying hand shapes limits the generalized performance of learning based methods. Also, manually annotating real data is suboptimal. Therefore, we present SynHand5M: a million-scale synthetic benchmark with accurate joint annotations, segmentation masks and mesh files of depth maps. Among model based learning (hybrid) methods, we show improved results on two of the public benchmarks i.e. NYU and ICVL. Also, by employing a joint training strategy with real and synthetic data, we recover 3D hand mesh and pose from real images in 30ms.
更多查看译文
关键词
hand pose and shape,synthetic depth,convolutional neural networks
AI 理解论文
溯源树
样例
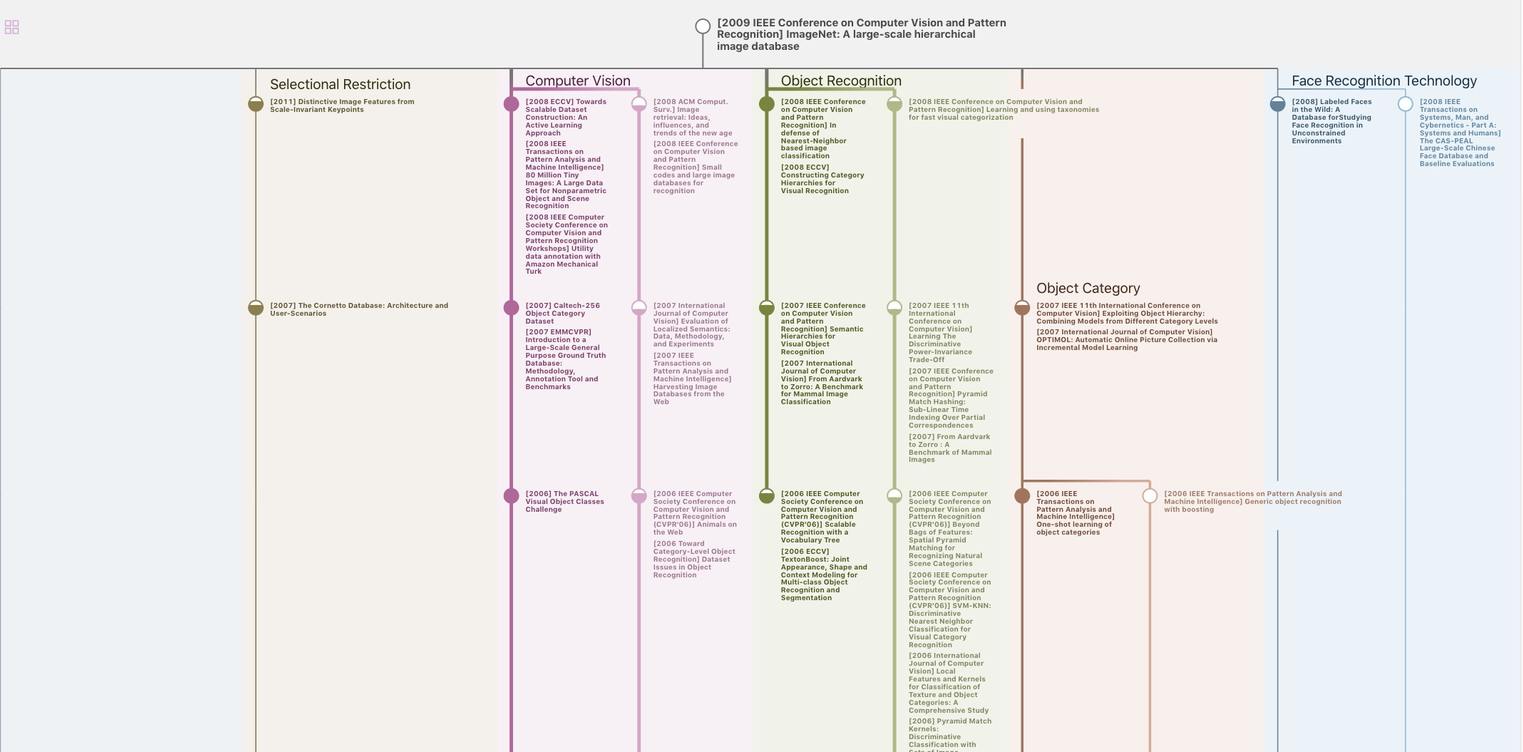
生成溯源树,研究论文发展脉络
Chat Paper
正在生成论文摘要