Jo-DPMF: Differentially private matrix factorization learning through joint optimization.
Information Sciences(2018)
摘要
Stochastic gradient descent (SGD) is a widely-used technique to implement matrix factorization. SGD-based matrix factorization involves many iterative computations. Therefore, according to the sequential composition theory of differential privacy, conventional implementation strategies of differentially private matrix factorization may lead to significant error accumulation, no matter whether the Laplace noise is added to the original matrix or to the factorized matrices. In fact, the implementation of differentially private matrix factorization is so challenging that results proposed to date have the problem of inefficient privacy and data utility. In this paper, we employ the objective perturbation method to address the challenge; this method dramatically alleviates error accumulation by perturbing the objective function instead of perturbing the results. Our method outperforms the state-of-the-art methods since it only requires a scalar noise rather than a vector noise to achieve the same magnitude of privacy. Furthermore, our method may learn the resulted matrices by joint optimization, which follows the conventional learning procedure of SGD and optimizes its convergence speed and accuracy as much as possible. In addition to the differential privacy guarantee, we also empirically show the way that the novel model works together with k-coRating, a k-anonymity-like privacy preserving model, to enhance data utility.
更多查看译文
关键词
Matrix factorization,Differential privacy,Recommender systems,Collaborative filtering,Stochastic gradient descent
AI 理解论文
溯源树
样例
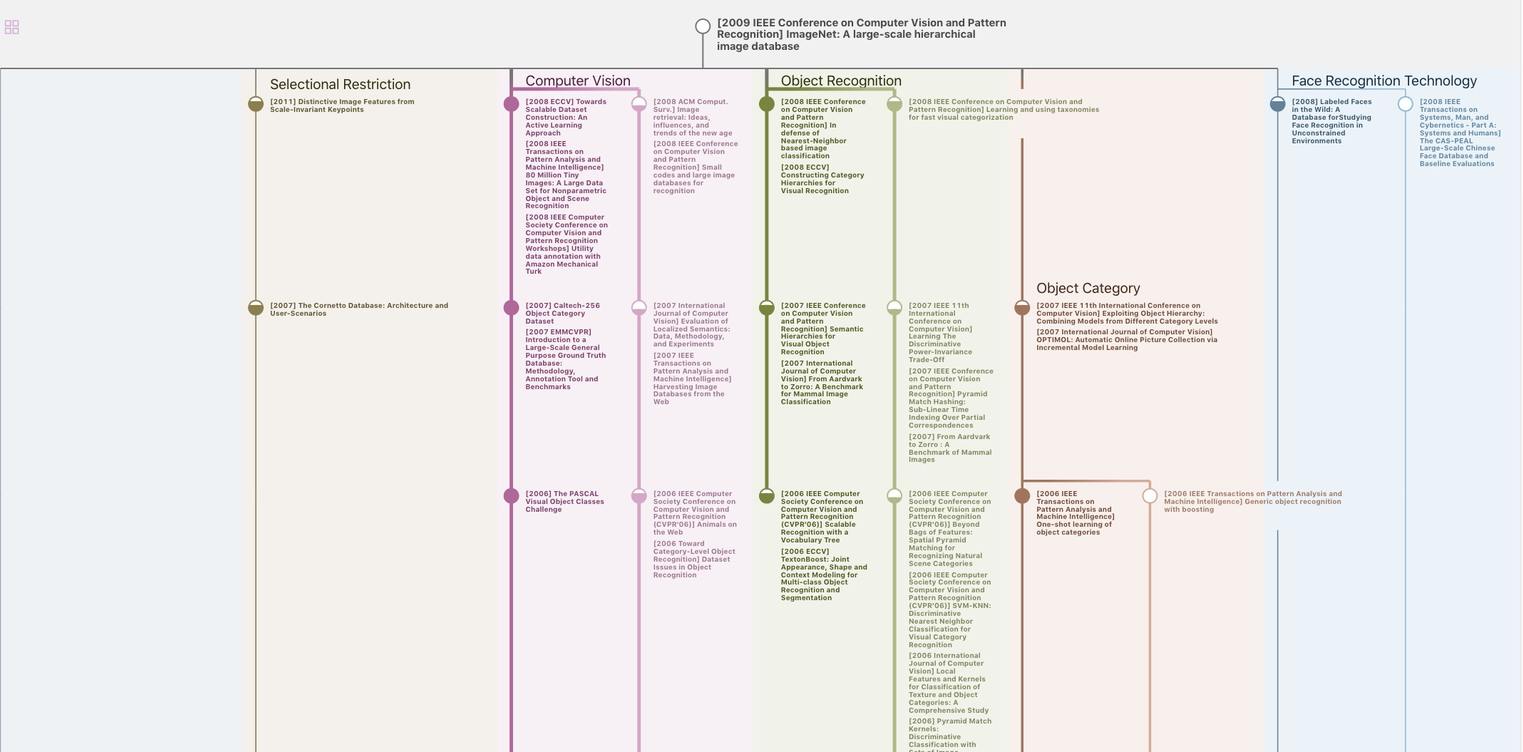
生成溯源树,研究论文发展脉络
Chat Paper
正在生成论文摘要