An Enhanced Single-Pair Learning-Based Reflectance Fusion Algorithm with Spatiotemporally Extended Training Samples.
REMOTE SENSING(2018)
摘要
Spatiotemporal fusion methods are considered a useful tool for generating multi-temporal reflectance data with limited high-resolution images and necessary low-resolution images. In particular, the superiority of sparse representation-based spatiotemporal reflectance fusion model (SPSTFM) in capturing phenology and type changes of land covers has been preliminarily demonstrated. Meanwhile, the dictionary training process, which is a key step in the sparse learning-based fusion algorithm, and its effect on fusion quality are still unclear. In this paper, an enhanced spatiotemporal fusion scheme based on the single-pair SPSTFM algorithm has been proposed through improving the process of dictionary learning, and then evaluated using two actual datasets, with one representing a rural area with phenology changes and the other representing an urban area with land cover type changes. The validated strategy for enhancing the dictionary learning process is divided into two modes to enlarge the training datasets with spatially and temporally extended samples. Compared to the original learning-based algorithm and other employed typical single-pair-based fusion models, experimental results from the proposed fusion method with two extension modes show improved performance in modeling reflectance using the two preceding datasets. Furthermore, the strategy with temporally extended training samples is more effective than the strategy with spatially extended training samples for the land cover area with phenology changes, whereas it is opposite for the land cover area with type changes.
更多查看译文
关键词
sparse learning,single image-pair,reflectance fusion,dictionary training,spatiotemporal extension
AI 理解论文
溯源树
样例
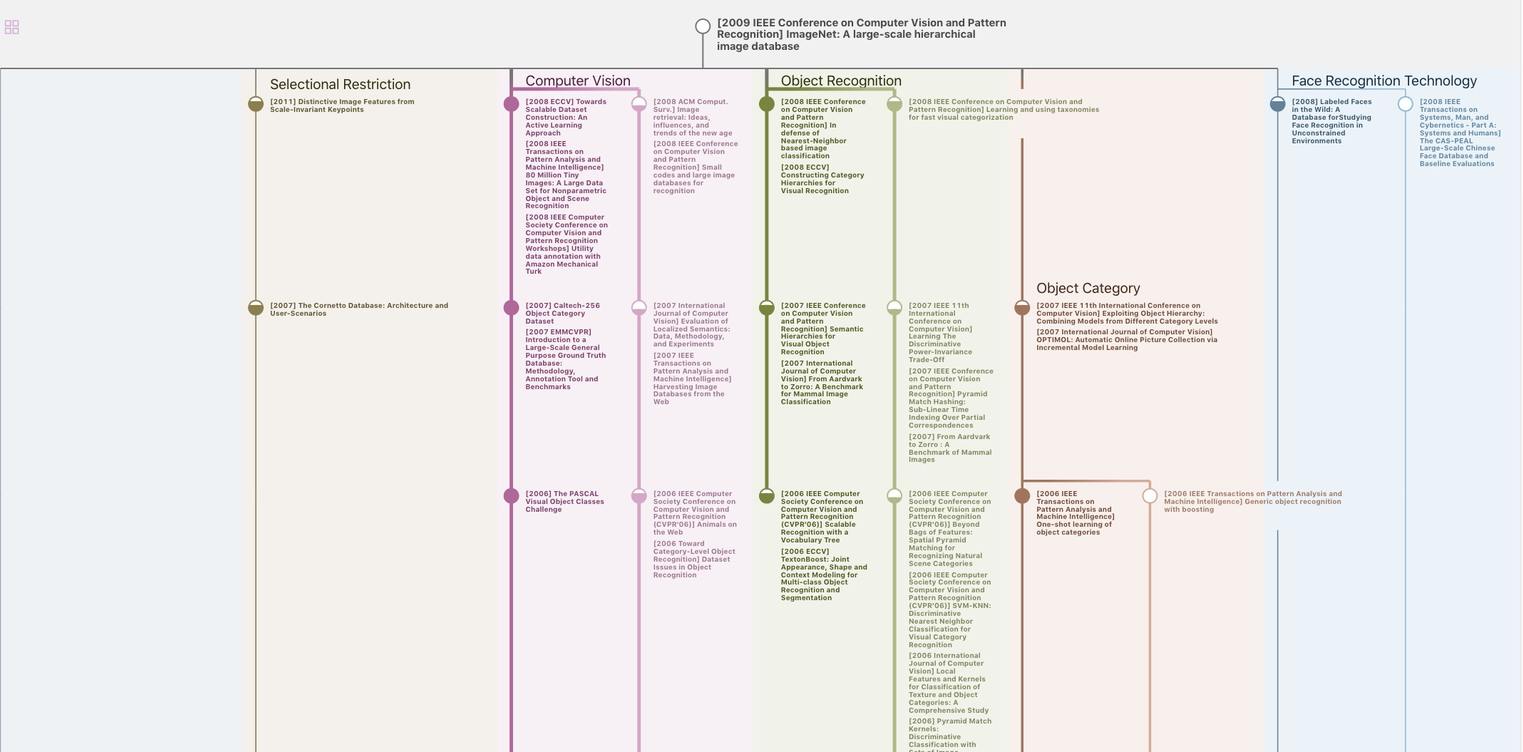
生成溯源树,研究论文发展脉络
Chat Paper
正在生成论文摘要