A robust convolutional neural network for online smartphone-based human activity recognition.
JOURNAL OF INTELLIGENT & FUZZY SYSTEMS(2018)
摘要
The online smartphone-based human activity recognition (HAR) has a variety of applications such as fitness tracking, healthcare ... etc. Currently, the signals generated from smartphone-embedded sensors are used for HAR systems. The smartphone-embedded sensors are utilized in order to provide an unobtrusive platform for HAR. In this paper, we propose a deep convolution neural network (CNN) model that provides an effective and efficient smartphone-based HAR system. For automatic local features extraction from the raw time-series data, we use the CNN while simple time-domain statistical features are used to extract more distinguishable features. Furthermore, we explore the impact of a novel data augmentation on the recognition accuracy of the proposed model. The performance of the proposed method is evaluated using two public data sets (UCI and WISDM) which are collected using smartphones. Experimentally, we show how the proposed model establishes the state-of-the-art performance using these datasets. Finally, to demonstrate the applicability of the proposed model for online smartphone-based HAR, the computational cost of the model is evaluated.
更多查看译文
关键词
Deep learning,convolutional neural network,smartphone-based human activity recognition,data augmentation,HAR
AI 理解论文
溯源树
样例
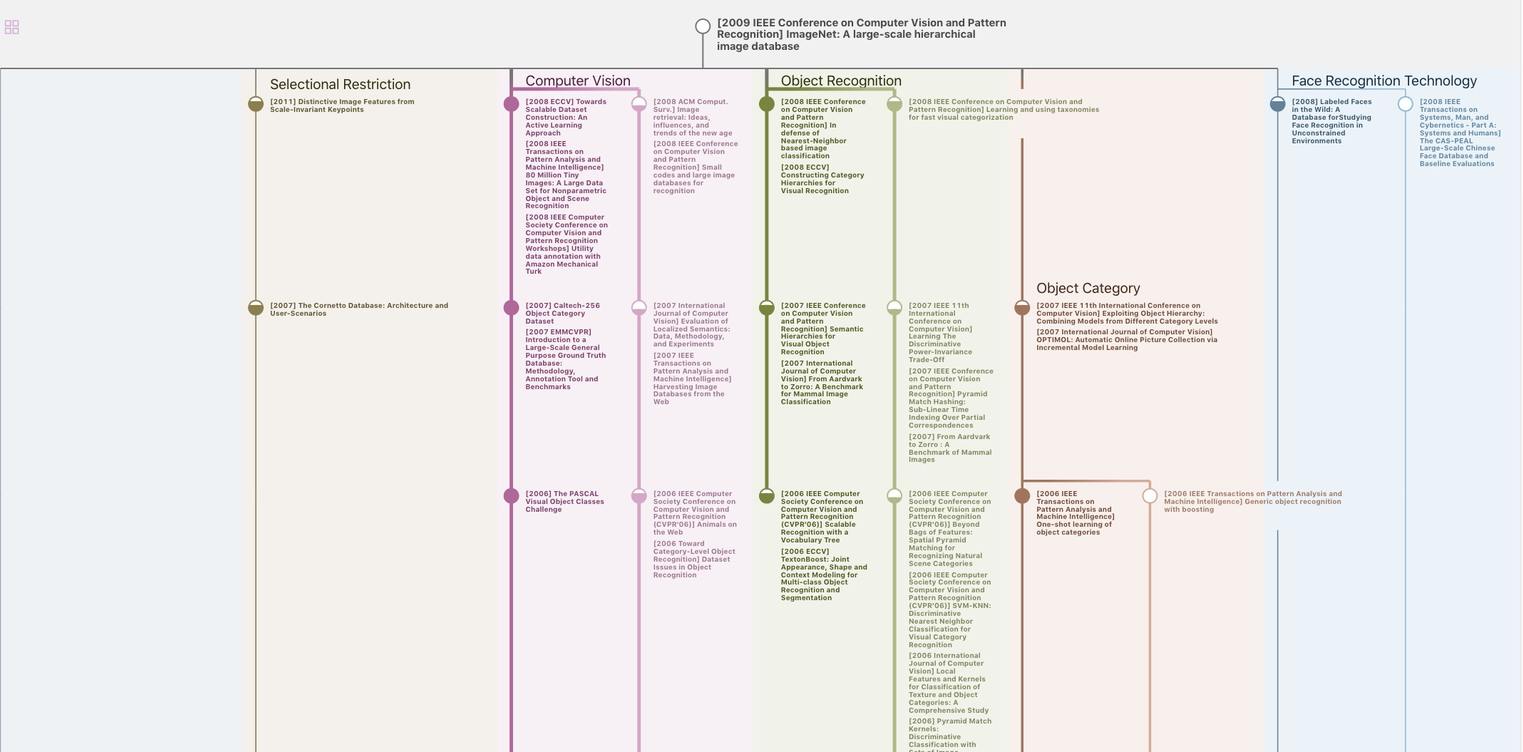
生成溯源树,研究论文发展脉络
Chat Paper
正在生成论文摘要