Independent Component Analysis Using Semi-Parametric Density Estimation Via Entropy Maximization.
SSP(2018)
摘要
Independent component analysis (ICA) is one of the most popular methods for blind source separation with a diverse set of applications, such as: biomedical signal processing, video and image analysis, and communications. The success of ICA is tied to proper characterization of the probability density function (PDF) of the latent sources; information that is generally unknown. In this work, we propose a new and efficient ICA algorithm based on entropy maximization with kernels, (ICA-EMK), which uses both global and local measuring functions as constraints to dynamically estimate the PDF of the sources. We present a mathematical justification of its convergence and demonstrate its superior performance over competing ICA algorithms using simulated as well as real-world data.
更多查看译文
关键词
Independent component analysis, entropy maximization
AI 理解论文
溯源树
样例
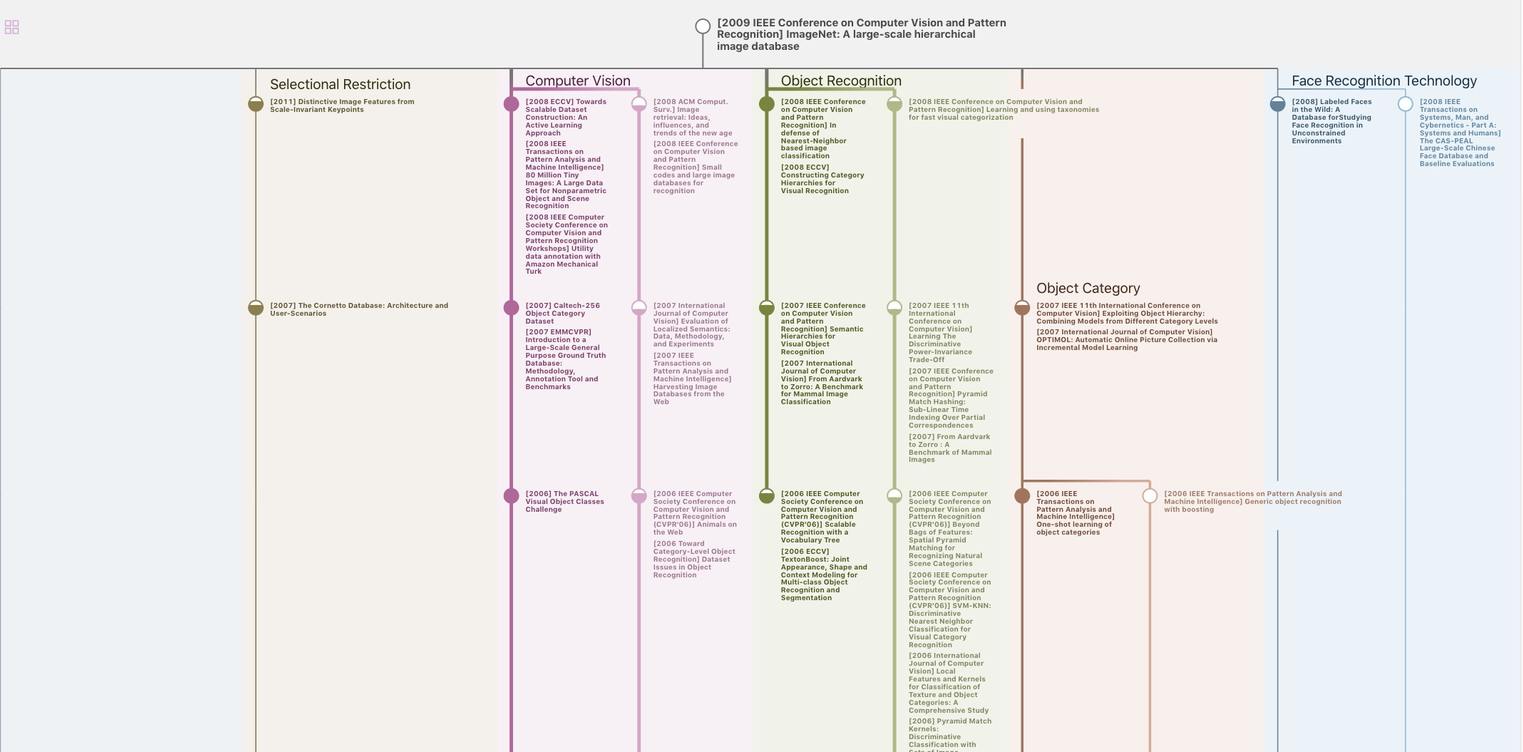
生成溯源树,研究论文发展脉络
Chat Paper
正在生成论文摘要