Speech Dereverberation Based On Convex Optimization Algorithms For Group Sparse Linear Prediction
2018 IEEE INTERNATIONAL CONFERENCE ON ACOUSTICS, SPEECH AND SIGNAL PROCESSING (ICASSP)(2018)
Abstract
In this paper, we consider methods for improving far-field speech recognition using dereverberation based on sparse multi-channel linear prediction. In particular, we extend successful methods based on nonconvex iteratively reweighted least squares, that look for a sparse desired speech signal in the short-term Fourier transform domain, by proposing sparsity promoting convex functions. Furthermore, we show how to improve performance by applying regularization into both the reweighted least squares and convex methods. We evaluate the methods using large scale simulations by mimicking the application scenarios of interest. The experiments show that the proposed convex formulations and regularization offer improvements over existing methods with added robustness and flexibility in fairly different acoustic scenarios.
MoreTranslated text
Key words
short-term Fourier transform domain,sparsity promoting convex functions,large scale simulations,acoustic scenarios,sparse desired speech signal,nonconvex iteratively reweighted least squares,sparse multichannel linear prediction,far-field speech recognition,group sparse linear prediction,convex optimization algorithms,speech dereverberation
AI Read Science
Must-Reading Tree
Example
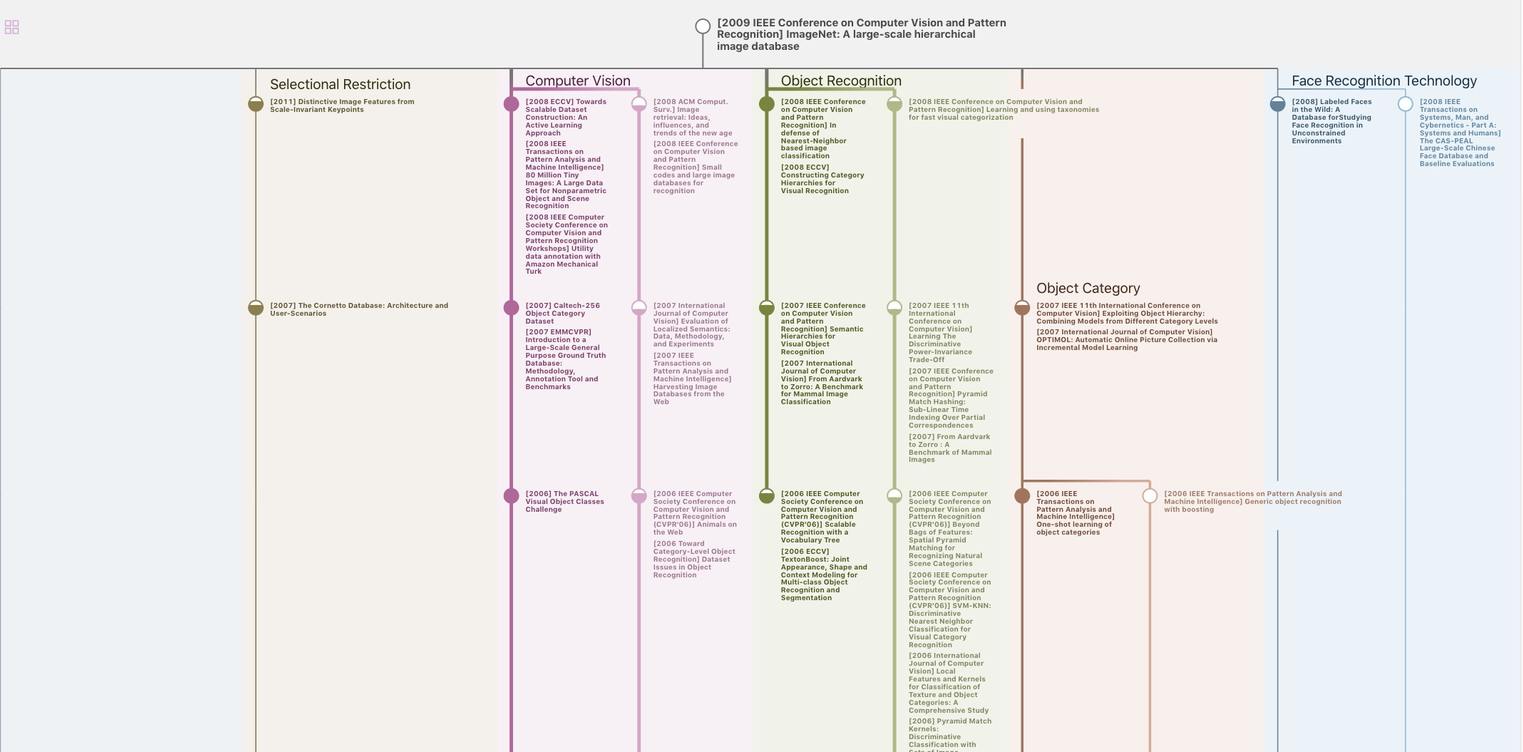
Generate MRT to find the research sequence of this paper
Chat Paper
Summary is being generated by the instructions you defined