Matrix Completion As Graph Bandlimited Reconstruction
2018 IEEE INTERNATIONAL CONFERENCE ON ACOUSTICS, SPEECH AND SIGNAL PROCESSING (ICASSP)(2018)
摘要
This paper develops new designs for recommender systems inspired by recent advances in graph signal processing. Recommender systems aim to predict unknown ratings by exploiting the information revealed in a subset of user-item observed ratings. Leveraging the notions of graph frequency and graph filters, we demonstrate that linear latent factor models, such as low-rank matrix completion, can be viewed as bandlimited interpolation algorithms that operate in a frequency domain given by the spectrum of a joint user and item network. This new interpretation paves the way to new methods for enhanced rating prediction. We propose a low complexity method by exploiting the eigen-vector of correlation matrices constructed from known ratings. In the MovieLens 100k dataset, our designs reduce the root mean squared error compared to the ones in benchmark matrix completion by 0.6% and benchmark nearest neighbor methods by 4.2%.
更多查看译文
关键词
Collaborative filtering, recommender systems, graph signal processing, graph filters, matrix completion
AI 理解论文
溯源树
样例
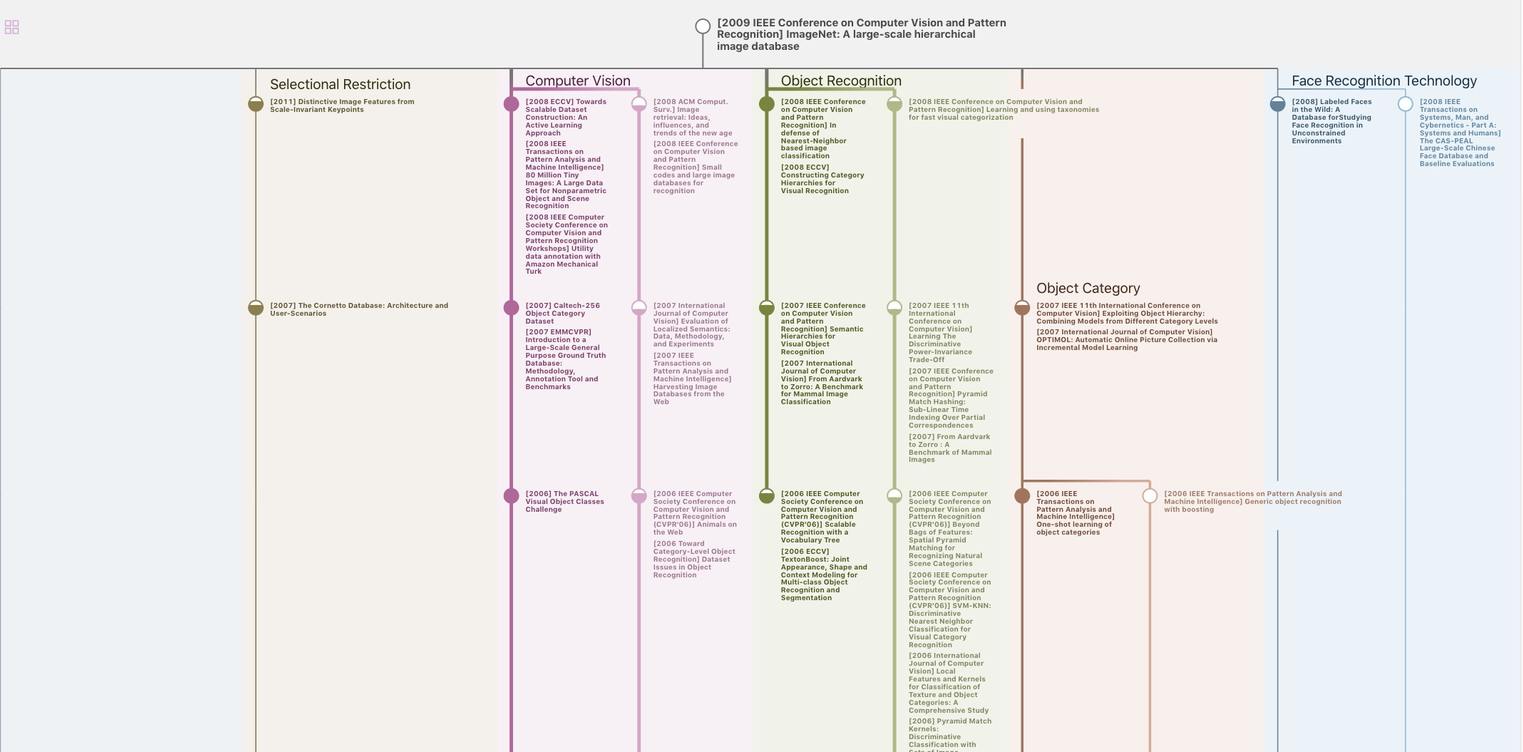
生成溯源树,研究论文发展脉络
Chat Paper
正在生成论文摘要